基于记忆的不变图像特征学习方法研究
项目介绍
AI项目解读
基本信息
- 批准号:61502195
- 项目类别:青年科学基金项目
- 资助金额:20.0万
- 负责人:
- 依托单位:
- 学科分类:F0605.模式识别与数据挖掘
- 结题年份:2018
- 批准年份:2015
- 项目状态:已结题
- 起止时间:2016-01-01 至2018-12-31
- 项目参与者:赵刚; 邓鹤; 刘乐元; 张文明; 杨蕾; 王翔;
- 关键词:
项目摘要
Image feature learning is the key part of image classification, and a research hotspot in the fields of pattern recognition and computer vision. However, the traditional feature extraction methods have constraints of complex background, rotation, scale changes, and so on. This makes image classification systems cannot achieve good generalization performance and have high sample complexity. This project will study memory-based invariant feature learning method for image classification by introducing manifold learning, invariant group and entropy theory etc. Firstly, this project will study the coding theory and method in the memory-based hierarchical learning model by fusing manifold learning, and pooling method which makes use of the statistical characteristics of the output of the coding layer and has the outputs with vector form. This study can enhance invariance to rotation, scale changes, local deformation, and so on. And the invariant features can reduce the sample complexity of image classification systems. Secondly, template learning methods in the memory-based invariant feature learning method will be designed according to the characteristic of different layers. The proposed methods can enhance invariance and discriminability of image features, reduce the impact of the complex background, and improve the classification accuracy. Finally, the invariance and discriminant analysis of the proposed feature learning methods will be given from the perspectives of group and entropy. It can reveal the mechanism of the hierarchical invariant feature learning methods. This research will enrich theoretical basis of image feature learning and provide key techniques for the design and develop of the computer vision systems.
图像特征学习是图像分类的关键环节,是模式识别与计算机视觉领域中的研究热点。传统特征提取方法受到复杂背景、旋转以及尺度变化等多种因素制约,导致图像分类系统的泛化性较差且样本复杂度较高。本项目拟面向图像分类,引入流形学习、不变群以及熵等理论开展基于记忆的不变特征学习方法研究。首先,融合流形学习理论,研究基于记忆的分层学习模型中的编码理论与方法,并利用编码输出的统计特征探讨具有向量形式输出的汇聚方法,提升特征对旋转、尺度变化以及局部形变等变化的不变性,降低图像分类系统的样本复杂度;其次,根据基于记忆的特征学习方法中不同层次的特点研究模板学习理论与方法,进一步增强图像特征的不变性与判别性,降低复杂背景的影响,提高分类精度;最后,从群和熵的角度分析特征学习方法的不变性与判别性,揭示分层不变特征学习方法的内在机制。本研究将进一步丰富图像特征学习的理论基础,为计算机视觉系统的设计和开发提供关键技术支撑。
结项摘要
图像特征学习是图像分类过程中的关键环节。获取对图像中无关变换具有不变性的特征一直是机器学习和计算机视觉领域重点关注的内容。本项目在分层学习框架下,针对不变图像特征学习中的关键问题进行了深入研究,在理论分析和算法设计两个方面都取得了比较好的研究成果,主要研究内容包括:针对高光谱图像分类中的不变特征学习问题构建了一种分层学习理论框架:空谱反应,设计了相应的编码和汇聚方法;并结合流形学习理论,在空谱反应理论框架下通过设计两种模板(记忆)学习方法构建了基于子空间学习的网络,用来学习高光谱图像的不变空谱特征,该算法样本复杂度低;针对基于子空间学习的网络易导致数据内在结构信息丢失的问题,设计了栈式张量子空间学习算法,该算法主要利用张量型学习算法充分保持图像的结构信息;结合高光谱图像“空谱合一”特性设计了栈式核极限学习机,该算法利用小波、核极限学习机和Gabor滤波器构建分层结构,提取高光谱图像的不变空谱特征;在Rényi信息熵的框架下,项目组设计了基于相关信息原则的高光谱图像特征学习方法,从熵的角度来平衡特征学习过程中的不变性与区分性,该方法是一种融合流形学习与熵理论的分层模型,有效融合高光谱数据的光谱和空间特征,可以获得具不变性的空谱特征,且其样本复杂度较低;针对学生行为识别中的特征学习困难,项目组利用流形学习理论改进宽度学习系统,并结合局部Log-Euclidean多变量高斯描述子构建了一种分层学习模型,该模型可以从小样本中学习得到有效的图像特征。到目前为止,项目组共发表学术论文16篇,其中SCI检索论文9篇,论文他引64次,申请发明专利2项,较为圆满地完成了项目研究目标。
项目成果
期刊论文数量(10)
专著数量(0)
科研奖励数量(0)
会议论文数量(6)
专利数量(2)
Multiscale patch-based contrast measure for small infrared target detection
用于小型红外目标检测的基于多尺度块的对比度测量
- DOI:10.1016/j.patcog.2016.04.002
- 发表时间:2016-10-01
- 期刊:PATTERN RECOGNITION
- 影响因子:8
- 作者:Wei, Yantao;You, Xinge;Li, Hong
- 通讯作者:Li, Hong
An infrared small target detection method based on multiscale local homogeneity measure
基于多尺度局部均匀性测度的红外小目标检测方法
- DOI:10.1016/j.infrared.2018.03.006
- 发表时间:2018-05-01
- 期刊:INFRARED PHYSICS & TECHNOLOGY
- 影响因子:3.3
- 作者:Nie, Jinyan;Qu, Shaocheng;Deng, Lizhen
- 通讯作者:Deng, Lizhen
A low-cost real-time face tracking system for ITSs and SDASs
适用于 ITS 和 SDAS 的低成本实时人脸跟踪系统
- DOI:10.1002/spe.2455
- 发表时间:2017
- 期刊:SOFTWARE-PRACTICE & EXPERIENCE
- 影响因子:3.5
- 作者:Liu Leyuan;Chen Jingying;Gao Changxin;Sang Nong
- 通讯作者:Sang Nong
Small infrared target detection based on image patch ordering
基于图像块排序的红外小目标检测
- DOI:10.1142/s0219691316400075
- 发表时间:2016-03
- 期刊:International Journal of Wavelets Multiresolution and Information Processing
- 影响因子:1.4
- 作者:Yantao Wei;Xinge You;He Deng
- 通讯作者:He Deng
深度学习在基于单幅图像的物体三维重建中的应用综述
- DOI:--
- 发表时间:--
- 期刊:自动化学报
- 影响因子:--
- 作者:陈加;张玉麒;宋鹏;魏艳涛;王煜
- 通讯作者:王煜
数据更新时间:{{ journalArticles.updateTime }}
{{
item.title }}
{{ item.translation_title }}
- DOI:{{ item.doi || "--"}}
- 发表时间:{{ item.publish_year || "--" }}
- 期刊:{{ item.journal_name }}
- 影响因子:{{ item.factor || "--"}}
- 作者:{{ item.authors }}
- 通讯作者:{{ item.author }}
数据更新时间:{{ journalArticles.updateTime }}
{{ item.title }}
- 作者:{{ item.authors }}
数据更新时间:{{ monograph.updateTime }}
{{ item.title }}
- 作者:{{ item.authors }}
数据更新时间:{{ sciAawards.updateTime }}
{{ item.title }}
- 作者:{{ item.authors }}
数据更新时间:{{ conferencePapers.updateTime }}
{{ item.title }}
- 作者:{{ item.authors }}
数据更新时间:{{ patent.updateTime }}
其他文献
深度学习在基于单幅图像的物体三维重建中的应用
- DOI:10.16383/j.aas.2018.c180236
- 发表时间:2019
- 期刊:自动化学报
- 影响因子:--
- 作者:陈加;张玉麒;宋鹏;魏艳涛;王煜
- 通讯作者:王煜
其他文献
{{
item.title }}
{{ item.translation_title }}
- DOI:{{ item.doi || "--" }}
- 发表时间:{{ item.publish_year || "--"}}
- 期刊:{{ item.journal_name }}
- 影响因子:{{ item.factor || "--" }}
- 作者:{{ item.authors }}
- 通讯作者:{{ item.author }}
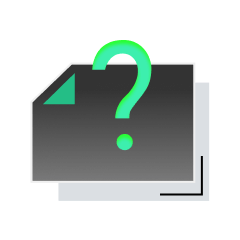
内容获取失败,请点击重试
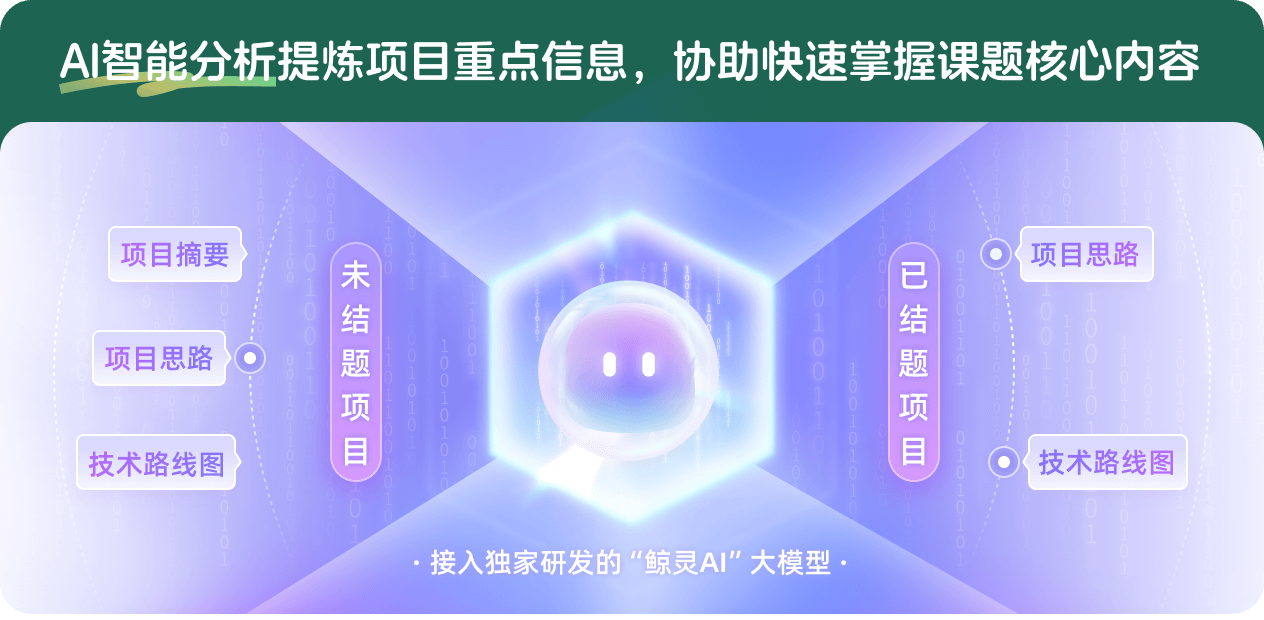
查看分析示例
此项目为已结题,我已根据课题信息分析并撰写以下内容,帮您拓宽课题思路:
AI项目摘要
AI项目思路
AI技术路线图
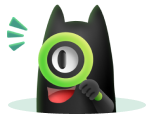
请为本次AI项目解读的内容对您的实用性打分
非常不实用
非常实用
1
2
3
4
5
6
7
8
9
10
您认为此功能如何分析更能满足您的需求,请填写您的反馈:
魏艳涛的其他基金
面向同步直播课堂的可解释学习投入自动评测方法研究
- 批准号:62277029
- 批准年份:2022
- 资助金额:50 万元
- 项目类别:面上项目
相似国自然基金
{{ item.name }}
- 批准号:{{ item.ratify_no }}
- 批准年份:{{ item.approval_year }}
- 资助金额:{{ item.support_num }}
- 项目类别:{{ item.project_type }}
相似海外基金
{{
item.name }}
{{ item.translate_name }}
- 批准号:{{ item.ratify_no }}
- 财政年份:{{ item.approval_year }}
- 资助金额:{{ item.support_num }}
- 项目类别:{{ item.project_type }}