双能锥束CT成像多材料分解技术研究
项目介绍
AI项目解读
基本信息
- 批准号:81871351
- 项目类别:面上项目
- 资助金额:57.0万
- 负责人:
- 依托单位:
- 学科分类:H2702.X射线与CT、电子与离子束
- 结题年份:2022
- 批准年份:2018
- 项目状态:已结题
- 起止时间:2019-01-01 至2022-12-31
- 项目参与者:王静; 罗辰; 施利明; 江阳康; 许镭; 薛一; 杨春林; 刘桥;
- 关键词:
项目摘要
Cone-beam CT is a concise and flexible technique to realize the structure imaging in clinical treatment. The dual-energy cone-beam CT utilizes the energy dependence of dual-energy X-ray data to realize a variety of human body components resolution and relative proportional quantitative analysis. Subject to the ill-posed problem of the material decomposition process and the relativity of the signal transmission, there are a limited number of material images generated by the decomposition of the dual-cone cone-beam CT images with high correlation noise and poor image accuracy. On the basis of the previous work, we study the transmission of noise from dual-energy CT image to decomposed image after scattering correction. We construct the variance of decomposed image according to the best linear unbiased estimation strategy and get the variance matrix for data error estimation. At the same time, based on the hypothesis of the finite composition of human body and the gray distribution similarity in each material, a generalized model of multi-material decomposition is established by using sparse constraints and total variation as regularization term. In order to improve the speed and accuracy of the solution, we use the block matching technique to search the gray similarity of human tissues and utilize the three-material hypothesis to approximate the sparse constraints. The method can effectively suppress the decomposition noise and improve the decomposition accuracy under the premise of maintaining the spatial resolution. This project intends to further improve the method performance and clinical practicability through meticulous method optimization and multiple image data studies. The proposed method can significantly improve the decomposition accuracy and suppress the decomposition of image noise while maintaining spatial resolution. This project will promote the clinical application of dual-energy cone-beam CT in radiation therapy, orthopedic, dentistry and so on.
锥束CT结构简洁灵活,是实现治疗中结构成像的重要手段。双能锥束CT挖掘双能谱X射线数据的能量依赖特性,实现多种人体成分分辨和相对比例定量化分析。受制于材料分解过程的病态性质和信号传递相关性,目前双能锥束CT图像分解产生的材料图数量有限、相关噪声强且图像精度差。本课题在前期工作基础上,对双能锥束CT图像进行散射修正后,研究噪声从双能CT图像到分解图像的传递过程,根据最佳线性无偏估计策略,构造分解图像的方差-协方差矩阵进行数据误差估计。同时,课题基于人体组成材料有限性和同种材料内部灰度相似性,使用稀疏约束和边界保持作为正则化项,建立多材料分解的通用模型。为提升求解速度和精度,课题借助区块匹配技术挖掘人体组织的灰度相似性,使用三材料假设近似稀疏约束。该方法可在保持空间分辨的前提下有效抑制分解图像噪声,并显著提升分解精度,推动双能锥束CT在放射治疗、牙科和骨科等治疗中引导和治疗后评估中的临床应用。
结项摘要
当前锥束CT成像受分解图像噪声污染、材料分解数量有限和分解精度低等问题制约,图像质量在CT值的准确性及均匀性上欠佳,造成双能成像的物质分解性能不理想,锥束CT双能成像的优势难以体现。为解决上述难题,本项目围绕双能锥束CT成像多材料分解技术,首先对锥束双能CT图像进行预处理,消除散射伪影;然后噪声从原始CT图像到分解图像的数学传递关系并有针对性的进行降噪方法学设计;研究双能多材料分解问题的病态性质,将材料内部相关性和材料间稀疏性引入到分解模型,建立通用锥束CT双能材料分解模型;研究基于临床先验知识引导的解决框架,实现双能多材料分解的高效率,高精度求解。按照申请书和资助计划书已完成全部研究内容和研究目标,包括:1)实现相关噪声的定向抑制,降低噪声标准差至常规值的20%以下,空间分辨不低于9线对/cm;2)通过三材料组成的假设近似稀疏约束,设计高效率和高精度的求解算法,提升分解精度至真值的80%以上;3)在实测患者数据上进行评估,综合衡量临床数据采集条件对分解图像质量的影响。采集20组患者数据进行评估测试,验证算法性能的统计显著性。在本项目执行期间,项目组共发表SCI论文20篇,所投期刊包括IEEE Transactions on Medical Imaging、IEEE Transactions on Biomedical Engineering、IEEE Transactions on Computational Imaging、Physics in Medicine and Biology等。申请了9项中国发明专利,国际会议口头报告5次,超额完成了发表SCI论文、国际会议报告的量化指标。本项目的开展能够为双能CT的广泛临床应用提供技术支持,有助于提升放疗计划的准确性,更好地实现疾病精准治疗。
项目成果
期刊论文数量(20)
专著数量(0)
科研奖励数量(0)
会议论文数量(0)
专利数量(9)
Extracting and Selecting Robust Radiomic Features from PET/MR Images in Nasopharyngeal Carcinoma
从鼻咽癌 PET/MR 图像中提取和选择稳健的放射学特征
- DOI:10.1007/s11307-020-01507-7
- 发表时间:2020-06-15
- 期刊:MOLECULAR IMAGING AND BIOLOGY
- 影响因子:3.1
- 作者:Yang, Pengfei;Xu, Lei;Niu, Tianye
- 通讯作者:Niu, Tianye
A temporo-spatial description of moving tumor and organs by the probability of presence time proportion: concept and implementation for 4D dose calculation and optimization
通过存在时间比例的概率对移动肿瘤和器官进行时空描述:4D剂量计算和优化的概念和实现
- DOI:10.1088/1361-6560/aca86c
- 发表时间:2022-12
- 期刊:Physics in Medicine & Biology
- 影响因子:3.5
- 作者:Qing Ren;Tianye Niu
- 通讯作者:Tianye Niu
Scatter correction of cone-beam CT using a deep residual convolution neural network (DRCNN)
使用深度残差卷积神经网络 (DRCNN) 对锥束 CT 进行散射校正
- DOI:10.1088/1361-6560/ab23a6
- 发表时间:2019-07-01
- 期刊:PHYSICS IN MEDICINE AND BIOLOGY
- 影响因子:3.5
- 作者:Jiang, Yangkang;Yang, Chunlin;Niu, Tianye
- 通讯作者:Niu, Tianye
Preoperative recurrence prediction in pancreatic ductal adenocarcinoma after radical resection using radiomics of diagnostic computed tomography.
使用诊断性计算机断层扫描放射组学预测胰腺导管腺癌根治术后复发
- DOI:10.1016/j.eclinm.2021.101215
- 发表时间:2022-01
- 期刊:EClinicalMedicine
- 影响因子:15.1
- 作者:Li X;Wan Y;Lou J;Xu L;Shi A;Yang L;Fan Y;Yang J;Huang J;Wu Y;Niu T
- 通讯作者:Niu T
Radiomics analysis combining unsupervised learning and handcrafted features: A multiple-disease study
结合无监督学习和手工特征的放射组学分析:多种疾病研究
- DOI:--
- 发表时间:2021
- 期刊:Medical Physics
- 影响因子:3.8
- 作者:Yidong Wan;Pengfei Yang;Lei Xu;Jing Yang;Chen Luo;Jing Wang;Feng Chen;Yan Wu;Yun Lu;Dan Ruan;Tianye Niu
- 通讯作者:Tianye Niu
数据更新时间:{{ journalArticles.updateTime }}
{{
item.title }}
{{ item.translation_title }}
- DOI:{{ item.doi || "--"}}
- 发表时间:{{ item.publish_year || "--" }}
- 期刊:{{ item.journal_name }}
- 影响因子:{{ item.factor || "--"}}
- 作者:{{ item.authors }}
- 通讯作者:{{ item.author }}
数据更新时间:{{ journalArticles.updateTime }}
{{ item.title }}
- 作者:{{ item.authors }}
数据更新时间:{{ monograph.updateTime }}
{{ item.title }}
- 作者:{{ item.authors }}
数据更新时间:{{ sciAawards.updateTime }}
{{ item.title }}
- 作者:{{ item.authors }}
数据更新时间:{{ conferencePapers.updateTime }}
{{ item.title }}
- 作者:{{ item.authors }}
数据更新时间:{{ patent.updateTime }}
其他文献
其他文献
{{
item.title }}
{{ item.translation_title }}
- DOI:{{ item.doi || "--" }}
- 发表时间:{{ item.publish_year || "--"}}
- 期刊:{{ item.journal_name }}
- 影响因子:{{ item.factor || "--" }}
- 作者:{{ item.authors }}
- 通讯作者:{{ item.author }}
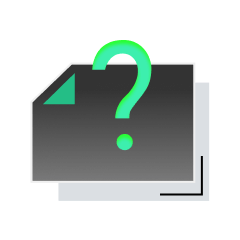
内容获取失败,请点击重试
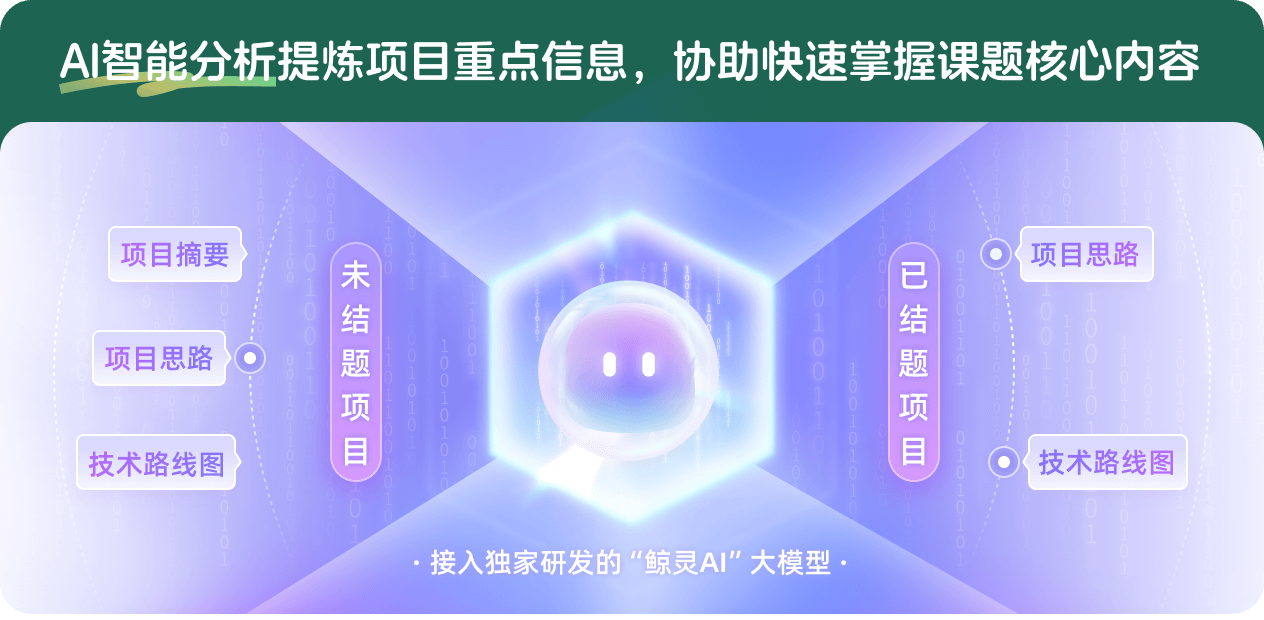
查看分析示例
此项目为已结题,我已根据课题信息分析并撰写以下内容,帮您拓宽课题思路:
AI项目摘要
AI项目思路
AI技术路线图
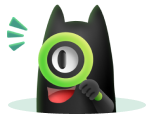
请为本次AI项目解读的内容对您的实用性打分
非常不实用
非常实用
1
2
3
4
5
6
7
8
9
10
您认为此功能如何分析更能满足您的需求,请填写您的反馈:
牛田野的其他基金
使用单次扫描和固定阻挡器的锥束CT散射修正方法研究
- 批准号:81201091
- 批准年份:2012
- 资助金额:24.0 万元
- 项目类别:青年科学基金项目
相似国自然基金
{{ item.name }}
- 批准号:{{ item.ratify_no }}
- 批准年份:{{ item.approval_year }}
- 资助金额:{{ item.support_num }}
- 项目类别:{{ item.project_type }}
相似海外基金
{{
item.name }}
{{ item.translate_name }}
- 批准号:{{ item.ratify_no }}
- 财政年份:{{ item.approval_year }}
- 资助金额:{{ item.support_num }}
- 项目类别:{{ item.project_type }}