高危型人乳头瘤病毒与肺癌发生的分子流行病学机制研究
项目介绍
AI项目解读
基本信息
- 批准号:81273148
- 项目类别:面上项目
- 资助金额:70.0万
- 负责人:
- 依托单位:
- 学科分类:H3010.非传染病流行病学
- 结题年份:2016
- 批准年份:2012
- 项目状态:已结题
- 起止时间:2013-01-01 至2016-12-31
- 项目参与者:张广健; 邓惠兴; 胡森科; 张敬华; 杨宇轩; 闫思琪; 陈新欢;
- 关键词:
项目摘要
Considering the key point of unknown mechanism and deficiency earlier and effective diagnosis index to predict the lung cancer development after the high-risk HPV infection, this study adopted the genetic and molecular biology technology, and epidemiological method to study the E6, E7, p53, pRb, FHIT gene mutation and their related protein expression changes after HPV integrated into the host cell DNA, determine the mutational site and the changes of protein expression and explore the direct evidence of HPV16 E6 induce p53 mutation through sequencing the E6, E7, mutant p53, and utilized the gene chip technology to detect the gene expression of E6, E7, p53, pRb, FHIT, single base mutation detection, genome polymorphism analysis and hybrid sequencing on the basis of the different levels of cells, genetic and protein. Meanwhile,Combined with epidemiology and the molecular biology research results, we discussed the relationship and the probably mechanism between the molecular biology change and the clinical and epidemiological distribution characteristic in lung cancer patients after the high-risk HPV infection. To provid the basis scientific data for lung cancer pathogenesis research, we also tried to reveal the earlier damage mechanism on the different aspect from the basic to clinical.
本研究针对高危型HPV感染宿主细胞致肺癌机制不明且缺乏早期有效诊断指标的难题,应用基因和蛋白表达等分子生物学技术和流行病学手段,从人体、细胞、基因、蛋白不同层次,集中研究HPV整合入宿主细胞DNA后,导致E6、E7、p53、pRb、FHIT基因突变,而引发其相关蛋白表达的下调或缺失;并通过对E6、E7、突变型p53序列测定,以确定E6、E7碱基突变位点及突变所致的蛋白表达改变,确定HPV16 E6诱导p53突变的直接证据;利用基因芯片对E6、E7、p53、pRb、FHIT基因表达、单碱基突变检测、基因组多态性分析及杂交测序,从流行病学角度,结合分子生物学研究结果,探讨高危型HPV感染导致的分子生物学改变与肺癌患者的临床和流行病学分布特征的关系,阐述高危型HPV与肺癌的分子流行病学机制。力求从基础与临床不同角度研究高危型HPV感染与肺癌发生的早期损伤机制,为肺癌发病机制的研究,提供科学依据。
结项摘要
肺癌的HPV感染率显著高于非癌对照,高危型HPV16和HPV18在肺癌组感染率均明显高于非癌对照。低危型HPV6的感染率显着低于非癌对照,说明高危型HPV16/18的感染与肺癌的发生关系更密切。HPV16与肿瘤细胞分化程度、TNM分期、淋巴结转移、性别和年龄均无关,但与肿瘤组织学类型和吸烟史显著相关。. 研究采用了logistic回归模型,将HPV的感染状态、高危险型HPV16及肺鳞癌作为三个因素的交互作用,将吸烟习惯纳入回归模型中,以评估校正可能存在的影响因素。结果显示,HPV阳性者患肺鳞癌的危险性为HPV阴性者的3.6倍。以吸烟习惯因素调整后,HPV阳性者患肺鳞癌的危险性为HPV阴性者的3.7倍。而高危型HPV16阳性者患肺鳞癌的危险性为HPV16阴性者的18.4倍。结果表明,吸烟习惯本身可能与人患肺癌无关,但若患者HPV16感染阳性,同时又是吸烟者,其患肺癌风险极高。. 肺鳞癌和腺癌患者中突变p53蛋白表达明显高于肺良性病变者,说明p53突变与肺癌发生关系密切。肺鳞癌患者中,78.1%的HPV阳性者出现p53突变蛋白表达,明显高于HPV阴性者,46.7%肺鳞癌中存在HPV感染和p53突变共存的现象,该结果进一步表明,肺鳞癌患者中HPV感染与p53基因突变存在明显关联性,HPV感染与p53突变在肺癌发生发展中可能起协同作用。且肺鳞癌患者中淋巴结转移越多、TNM分期越高,HPV感染和p53突变共存现象越明显。该结果进一步提示肺癌患者的HPV感染和p53突变与肿瘤的不良预后有关。. 肺鳞癌患者HPV感染率明显高于肺腺癌和小细胞肺癌,有吸烟史的HPV感染率明显高于无吸烟史者。肺癌组患者的FHIT基因缺失率明显高于非癌对照,其中FHIT基因的缺失与肿瘤的TNM分期、淋巴结转移、性别和年龄无关,但与组织学类型、细胞分化程度和吸烟史显著相关。肺鳞癌患者的FHIT基因缺失率明显高于肺腺癌和小细胞肺癌,细胞分化程度越低,FHIT基因缺失率越高;肺鳞癌患者有吸烟史的FHIT基因缺失率亦明显高于无吸烟史者。肺癌患者中FHIT基因缺失与吸烟史的并存率高达38.33%。 HPV16E6序列测定结果提示,其突变位点主要集中在第362 、350、83、176位碱基。. 以上研究结果将为进一步探讨肺癌的发病机制、早期诊断,提供科学依据。
项目成果
期刊论文数量(2)
专著数量(0)
科研奖励数量(0)
会议论文数量(0)
专利数量(0)
FHIT缺失和p53突变对HPV感染的肺癌发展的影响
- DOI:--
- 发表时间:2015
- 期刊:ONCOLOGY LETTERS
- 影响因子:2.9
- 作者:于燕
- 通讯作者:于燕
人乳头状瘤病毒16/18感染与肺癌中p53突变的显著相关性
- DOI:--
- 发表时间:2013
- 期刊:The Clinical Respiratory Journal
- 影响因子:--
- 作者:于燕
- 通讯作者:于燕
数据更新时间:{{ journalArticles.updateTime }}
{{
item.title }}
{{ item.translation_title }}
- DOI:{{ item.doi || "--"}}
- 发表时间:{{ item.publish_year || "--" }}
- 期刊:{{ item.journal_name }}
- 影响因子:{{ item.factor || "--"}}
- 作者:{{ item.authors }}
- 通讯作者:{{ item.author }}
数据更新时间:{{ journalArticles.updateTime }}
{{ item.title }}
- 作者:{{ item.authors }}
数据更新时间:{{ monograph.updateTime }}
{{ item.title }}
- 作者:{{ item.authors }}
数据更新时间:{{ sciAawards.updateTime }}
{{ item.title }}
- 作者:{{ item.authors }}
数据更新时间:{{ conferencePapers.updateTime }}
{{ item.title }}
- 作者:{{ item.authors }}
数据更新时间:{{ patent.updateTime }}
其他文献
生物质燃烧源PM2.5在水汽条件下长大特性的研究
- DOI:--
- 发表时间:2016
- 期刊:燃料化学学报
- 影响因子:--
- 作者:徐俊超;于燕;张军;孟强;钟辉;尹艳山
- 通讯作者:尹艳山
严重烧伤患者T淋巴细胞免疫功能变化及其与患者预后的关系
- DOI:--
- 发表时间:--
- 期刊:创伤外科杂志
- 影响因子:--
- 作者:黄立锋;姚咏明;龚平;董宁;于燕;贺立新;盛志勇
- 通讯作者:盛志勇
细颗粒长大特性的直接测量
- DOI:--
- 发表时间:2016
- 期刊:东南大学学报(自然科学版)
- 影响因子:--
- 作者:徐俊超;张军;于燕;孟强;杨林军;袁竹林;尹艳山
- 通讯作者:尹艳山
分段数对生长管过饱和环境特征的影响
- DOI:--
- 发表时间:2018
- 期刊:东南大学学报 (自然科学版)
- 影响因子:--
- 作者:于燕;张军;陈广闯;徐俊超;闾荔;钟辉
- 通讯作者:钟辉
三代繁殖低硒和低硒低碘大鼠动物模型的建立
- DOI:--
- 发表时间:--
- 期刊:卫生研究
- 影响因子:--
- 作者:苏敏;张瑞娟;田东萍;郭雄;于燕;王艳
- 通讯作者:王艳
其他文献
{{
item.title }}
{{ item.translation_title }}
- DOI:{{ item.doi || "--" }}
- 发表时间:{{ item.publish_year || "--"}}
- 期刊:{{ item.journal_name }}
- 影响因子:{{ item.factor || "--" }}
- 作者:{{ item.authors }}
- 通讯作者:{{ item.author }}
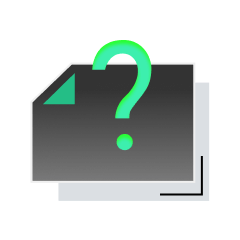
内容获取失败,请点击重试
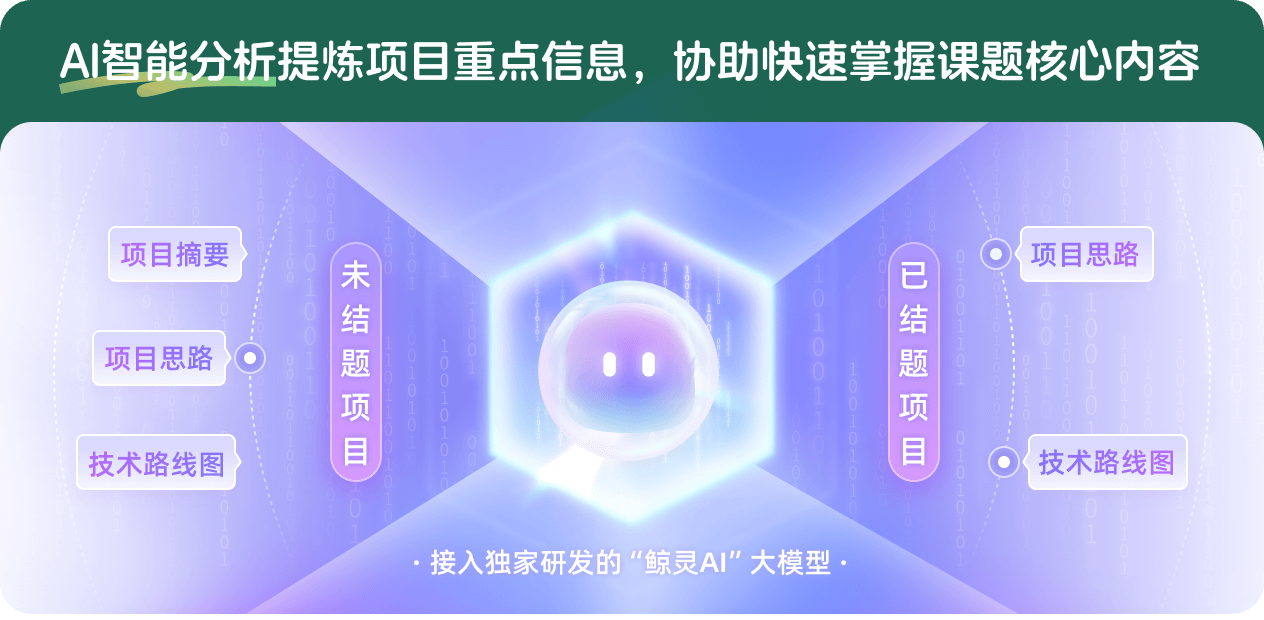
查看分析示例
此项目为已结题,我已根据课题信息分析并撰写以下内容,帮您拓宽课题思路:
AI项目摘要
AI项目思路
AI技术路线图
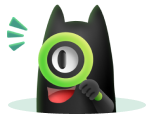
请为本次AI项目解读的内容对您的实用性打分
非常不实用
非常实用
1
2
3
4
5
6
7
8
9
10
您认为此功能如何分析更能满足您的需求,请填写您的反馈:
相似国自然基金
{{ item.name }}
- 批准号:{{ item.ratify_no }}
- 批准年份:{{ item.approval_year }}
- 资助金额:{{ item.support_num }}
- 项目类别:{{ item.project_type }}
相似海外基金
{{
item.name }}
{{ item.translate_name }}
- 批准号:{{ item.ratify_no }}
- 财政年份:{{ item.approval_year }}
- 资助金额:{{ item.support_num }}
- 项目类别:{{ item.project_type }}