弱监督视角下的网络表示学习研究
项目介绍
AI项目解读
基本信息
- 批准号:61902227
- 项目类别:青年科学基金项目
- 资助金额:25.0万
- 负责人:
- 依托单位:
- 学科分类:F0211.信息检索与社会计算
- 结题年份:2022
- 批准年份:2019
- 项目状态:已结题
- 起止时间:2020-01-01 至2022-12-31
- 项目参与者:--
- 关键词:
项目摘要
Large-scale network is of great academic and economic value in many national strategy fields and important industrial application directions. In this case, research of the network representation learning is the only way to break the bottleneck of analysis and applications in large-scale network. However, in many real scenarios, raw network data always contains plenty of information uncertainties, which could produce extremely adverse effects to the network representation learning result. Aiming at the problem of network representation learning under the influence of data uncertainties, this project proposes to conduct network representation learning research from a weekly supervised perspective. The main contents of the research include the following aspects: (1) To weaken the adverse effects of information uncertainty for representation result of network structure, we will study to establish weekly supervised learning model to use accompanying information to assist and modify the representation learning process; (2) To improve the reliability of representation result for accompanying information, we will study to fuse the weekly supervised information in network structure and construct optimization model for representation learning of accompanying information; (3) To acquire the optimal network representation that can promote network analysis performance, we will study to fuse and optimize the low dimensional representation of network structure and accompanying information based on specific analysis task. The solutions of these problems will be conducive to improve algorithm performance of representation learning and expand its application range. Besides, our research will further enrich and perfect the relative theories and methods for large-scale network analysis.
大规模网络在诸多国家战略领域和重要产业应用方向均具有重大研究和经济价值,开展网络表示学习研究是突破大规模网络分析和应用瓶颈的必经之路。在真实场景中,原始网络数据往往蕴含大量不确定性,对网络表示学习结果的可靠性和有效性产生极其不利的影响。为此,本项目针对数据不确定性影响下的网络表示学习研究问题,开展弱监督视角下的网络表示学习研究。主要研究内容包括:(1)研究如何建立网络结构的弱监督表示学习模型,利用伴随信息对表示学习过程进行补充和修正,提高其表示结果的可靠性;(2)研究如何融合网络结构中蕴含的弱监督信息,构建伴随信息表示学习的优化模型,降低数据不确定性的不利影响;(3)研究如何基于网络分析任务对网络结构和伴随信息的低维向量表示进行融合及优化,获得有利于提高目标任务性能的最优表示形式。这些问题的解决将有利于提高网络表示学习算法性能和拓展其应用范围,进一步完善大规模网络分析的相关理论和方法。
结项摘要
随着越来越多的数据以关联大数据形式存在,对网络数据进行高效分析和处理对于人们认识复杂系统内部模式及其演化规律至关重要。融合网络分析任务信息指导表示学习过程获得多模态网络数据的一致性低维表示,并对网络表示结果进行优化以提高其在目标任务约束下的有效性和稳定性,是进一步增强网络表示学习算法有效性和拓展其应用范畴的重要手段,也是从表征层面解决大规模网络分析瓶颈的关键问题。. 本项目致力于解决上述问题和挑战,以降低网络数据不确定性的不利影响为任务驱动,以提高网络表示结果可靠性和有效性为研究目标,开展弱监督视角下的网络表示学习研究,进一步拓展网络表示学习应用范围和提升大规模网络分析性能。项目取得主要研究成果如下:(1)在面向网络结构保持的弱监督网络表示学习方面,提出了融合伴随信息的网络表示学习模型、成对约束的标签传播算法、基于相似性注意力的社区发现模型;(2)在面向伴随信息的弱监督网络表示学习方面,提出了面向电商网络的异常用户检测方法、结合图自编码器与聚类的半监督表示学习方法;(3)在网络结构和伴随信息低维表示的融合及优化方面,提出了图神经网络视角下的深度聚类集成模型、基于多视图图聚类的电商异常检测方法、 深度自监督聚类集成方法。. 上述弱监督网络表示学习研究成果有助于提高网络表示学习的可靠性和有效性,对于进一步完善和丰富网络表示学习的理论与方法,拓展其应用范畴以及推动大规模网络分析技术等具有重要意义。这些成果在规模复杂场景的WIFI室内定位、脑部核磁共振图像分割、以及无人机飞行工况模式识别等领域获得成功应用,并完成成果转化。
项目成果
期刊论文数量(10)
专著数量(0)
科研奖励数量(0)
会议论文数量(0)
专利数量(4)
一种基于相似性注意力的社区发现模型
- DOI:--
- 发表时间:2022
- 期刊:山西大学学报
- 影响因子:--
- 作者:杜航原;姚倩;冯劲松;王文剑
- 通讯作者:王文剑
结合图自编码器与聚类的半监督表示学习方法
- DOI:--
- 发表时间:2022
- 期刊:计算机应用
- 影响因子:--
- 作者:杜航原;郝思聪;王文剑
- 通讯作者:王文剑
A Clustering Ensemble Framework with Integration of Data Characteristics and Structure Information: A Graph Neural Networks Approach
集成数据特征和结构信息的聚类集成框架:图神经网络方法
- DOI:10.3390/math10111834
- 发表时间:2022-05
- 期刊:Mathematics
- 影响因子:2.4
- 作者:Hangyuan Du;Wenjian Wang
- 通讯作者:Wenjian Wang
Abnormal User Detection via Multiview Graph Clustering in the Mobile e-Commerce Network
移动电子商务网络中多视图图聚类的异常用户检测
- DOI:10.1155/2022/3766810
- 发表时间:2022-08
- 期刊:Wireless Communications and Mobile Computing
- 影响因子:--
- 作者:Hangyuan Du;Duo Li;Wenjian Wang
- 通讯作者:Wenjian Wang
A Generative Clustering Ensemble Model and Its Application in IoT Data Analysis
生成聚类集成模型及其在物联网数据分析中的应用
- DOI:10.1155/2022/8081177
- 发表时间:2022-06-29
- 期刊:WIRELESS COMMUNICATIONS & MOBILE COMPUTING
- 影响因子:--
- 作者:Du,Hangyuan;Wang,Wenjian;Feng,Jinsong
- 通讯作者:Feng,Jinsong
数据更新时间:{{ journalArticles.updateTime }}
{{
item.title }}
{{ item.translation_title }}
- DOI:{{ item.doi || "--"}}
- 发表时间:{{ item.publish_year || "--" }}
- 期刊:{{ item.journal_name }}
- 影响因子:{{ item.factor || "--"}}
- 作者:{{ item.authors }}
- 通讯作者:{{ item.author }}
数据更新时间:{{ journalArticles.updateTime }}
{{ item.title }}
- 作者:{{ item.authors }}
数据更新时间:{{ monograph.updateTime }}
{{ item.title }}
- 作者:{{ item.authors }}
数据更新时间:{{ sciAawards.updateTime }}
{{ item.title }}
- 作者:{{ item.authors }}
数据更新时间:{{ conferencePapers.updateTime }}
{{ item.title }}
- 作者:{{ item.authors }}
数据更新时间:{{ patent.updateTime }}
其他文献
一种面向属性网络的重叠社区发现算法
- DOI:--
- 发表时间:2019
- 期刊:计算机应用
- 影响因子:--
- 作者:杜航原;裴希亚;王文剑
- 通讯作者:王文剑
基于模糊逻辑的SLAM数据关联方法
- DOI:--
- 发表时间:--
- 期刊:系统工程与电子技术
- 影响因子:--
- 作者:杜航原;郝燕玲;赵玉新
- 通讯作者:赵玉新
一种改进的粒子滤波算法及其在GPS/DR组合定位中的应用
- DOI:--
- 发表时间:2016
- 期刊:计算机科学
- 影响因子:--
- 作者:杜航原;王文剑;白亮
- 通讯作者:白亮
基于随机有限集的SLAM算法
- DOI:--
- 发表时间:--
- 期刊:系统工程与电子技术
- 影响因子:--
- 作者:杜航原;赵玉新;杨永鹏
- 通讯作者:杨永鹏
基于模糊自适应卡尔曼滤波的SLAM算法
- DOI:--
- 发表时间:--
- 期刊:华中科技大学学报(自然科学版)科技大学
- 影响因子:--
- 作者:杜航原;郝燕玲;赵玉新
- 通讯作者:赵玉新
其他文献
{{
item.title }}
{{ item.translation_title }}
- DOI:{{ item.doi || "--" }}
- 发表时间:{{ item.publish_year || "--"}}
- 期刊:{{ item.journal_name }}
- 影响因子:{{ item.factor || "--" }}
- 作者:{{ item.authors }}
- 通讯作者:{{ item.author }}
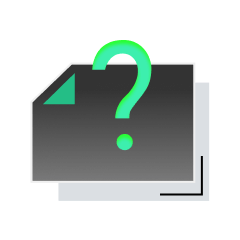
内容获取失败,请点击重试
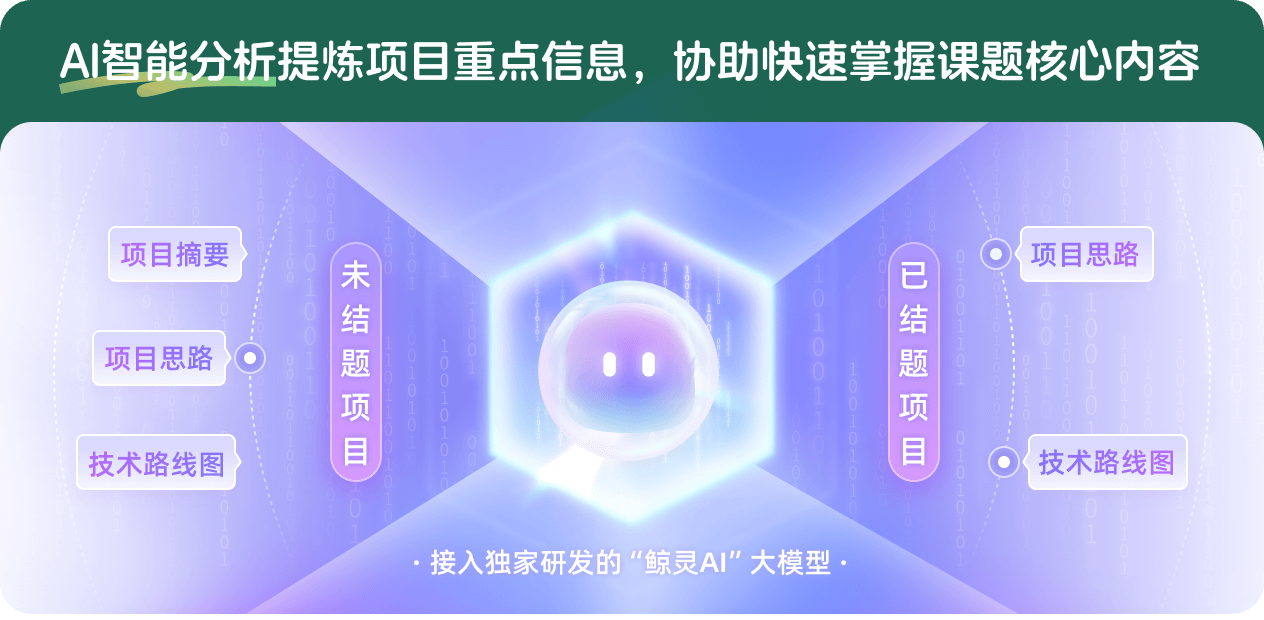
查看分析示例
此项目为已结题,我已根据课题信息分析并撰写以下内容,帮您拓宽课题思路:
AI项目摘要
AI项目思路
AI技术路线图
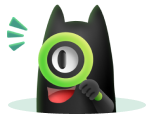
请为本次AI项目解读的内容对您的实用性打分
非常不实用
非常实用
1
2
3
4
5
6
7
8
9
10
您认为此功能如何分析更能满足您的需求,请填写您的反馈:
相似国自然基金
{{ item.name }}
- 批准号:{{ item.ratify_no }}
- 批准年份:{{ item.approval_year }}
- 资助金额:{{ item.support_num }}
- 项目类别:{{ item.project_type }}
相似海外基金
{{
item.name }}
{{ item.translate_name }}
- 批准号:{{ item.ratify_no }}
- 财政年份:{{ item.approval_year }}
- 资助金额:{{ item.support_num }}
- 项目类别:{{ item.project_type }}