面向阿尔兹海默病诊断的多模态特征提取方法
项目介绍
AI项目解读
基本信息
- 批准号:61866009
- 项目类别:地区科学基金项目
- 资助金额:38.0万
- 负责人:
- 依托单位:
- 学科分类:F0604.机器感知与机器视觉
- 结题年份:2022
- 批准年份:2018
- 项目状态:已结题
- 起止时间:2019-01-01 至2022-12-31
- 项目参与者:蓝如师; 王子民; 李灵巧; 黄源; 潘细朋; 马超; 姬欢欢; 王文颢;
- 关键词:
项目摘要
Computer-aided diagnosis of Alzheimer's disease (AD) via multi-Modality medical imaging is the hot research topic and frontiers of intelligent brain imaging analysis. However, it is a typical high dimensional and small sample machine learning problem, and the effective use of the complementary information on each modality is not solved well. This project makes a deep research on the above challenge and proposes a novel multi-modality image feature extraction method. First, we explore the effect of gray scale standardization on diagnosis of AD by restricting weight on the segmented voxels in the album model, and making full use of the information of the atlas while completing the segmentation and gray standardization of the image to be segmented. The method of joint brain structure segmentation and gray standardization is proposed based on the atlas model by the effect of gray standardization on image segmentation. Then, deep convolution network classification is studied based on the multi-linear model aiming at the problem of high-dimensional small samples. The target-level representation of the image is obtained by using a regularized multilinear regression model. We use the similarity of voxel on partial area to give the different weight on every voxel. At the same time, the similarity between the neighborhoods of each block is assigned different weights to obtain the target-level representation of the image as the input of the deep network and complete the image dimensionality reduction. The multi-linear model is used to comprehensively consider the complementary information of each modality, and then the classification of AD images is completed. Based on the above research, multi-instance learning is expended on AD diagnostic. T-test is used to sort the discrimination ability and generate the marker points of each modal image. Based on the marker points, different sizes blocks are used to calculate texture and multi-scale feature representation of landmarks. The feature representation of each marker point is seen as an example then we complete the multiple example representation of the image. To make full use of the image structural information and reduce the loss of structural information in images from 3D to 2D models, we try to explore the three-dimensional convolution network method. Preliminary experimental results show that the classification results of the proposed method are better than the existing methods. The project is of great significance for the improvement of the clinical diagnostic techniques of AD and the development of intelligent brain imaging computing.
基于多模态医学影像的阿尔兹海默症(AD)的计算机辅助诊断是脑影像智能计算领域的研究热点和前沿。但该问题是一个典型高维度小样本的机器学习问题,且各模态的互补信息的有效利用没有得到很好的解决。本项目围绕上述问题展开深入研究,提出新型的多模态图像特征提取方法:(1)研究图像灰度标准化对AD诊断的影响,通过在图册模型中对待分割的体素进行加权约束,在完成分割的同时,充分利用图册的信息,完成待分割图像的灰度标准化;综合考虑灰度标准化对图像分割的影响,提出基于图册表观模型的联合脑结构分割与灰度标准化方法。(2)针对高维度小样本的问题,研究基于多线性模型的深度卷积网络分类方法:利用正则化的多线性回归模型获得图像的目标级表示,通过考虑目标图像每一个体素点对应的局部区域的相似度,对每一个体素点都赋予一个不同的权重;同时考虑每个块邻域之间的相似度,赋予不同的权重获得图像的目标级表示,作为深度网络的输入,完成图像降维。同时使用多线性模型综合考虑各个模态的互补信息,进而完成AD图像的分类。在此基础上,研究基于多示例学习的AD 诊断方法:利用T-test 方法,通过对像素点判别能力的排序,生成每种模态图像的标志点;以标志点为中心,取不同大小的块为基础,计算纹理等特征,计算标志点的多尺度特征表示;每个标志点的特征表示作为一个示例,完成图像的多示例表示。最后,探索三维的卷积神经网络方法,充分利用图像的结构信息,降低图像在从三维到二维模型中的结构信息的丢失。初步的实验结果表明,本项目提出方法的分类结果优于现有方法。本项目的研究内容对于AD临床诊断技术水平的提高、脑影像智能计算的发展都具有重要意义。
结项摘要
基于多模态医学影像的阿尔兹海默症(AD)的计算机辅助诊断是脑影像智能计算领域的研究热点和前沿。但该问题是一个典型高维度小样本的机器学习问题,且各模态的互补信息的有效利用没有得到很好的解决。本项目围绕上述问题展开深入研究,提出新型的多模态图像特征提取方法:(1)研究图像灰度标准化对AD诊断的影响,通过在图册模型中对待分割的体素进行加权约束,在完成分割的同时,充分利用图册的信息,完成待分割图像的灰度标准化;综合考虑灰度标准化对图像分割的影响,提出基于图册表观模型的联合脑结构分割与灰度标准化方法。(2)针对高维度小样本的问题,研究基于多线性模型的深度卷积网络分类方法:利用正则化的多线性回归模型获得图像的目标级表示,通过考虑目标图像每一个体素点对应的局部区域的相似度,对每一个体素点都赋予一个不同的权重;同时考虑每个块邻域之间的相似度,赋予不同的权重获得图像的目标级表示,作为深度网络的输入,完成图像降维。同时使用多线性模型综合考虑各个模态的互补信息,进而完成AD图像的分类。在此基础上,研究基于多示例学习的AD 诊断方法:利用T-test 方法,通过对像素点判别能力的排序,生成每种模态图像的标志点;以标志点为中心,取不同大小的块为基础,计算纹理等特征,计算标志点的多尺度特征表示;每个标志点的特征表示作为一个示例,完成图像的多示例表示。最后,探索三维的卷积神经网络方法,充分利用图像的结构信息,降低图像在从三维到二维模型中的结构信息的丢失。初步的实验结果表明,本项目提出方法的分类结果优于现有方法。本项目的研究内容对于AD临床诊断技术水平的提高、脑影像智能计算的发展都具有重要意义。
项目成果
期刊论文数量(13)
专著数量(0)
科研奖励数量(0)
会议论文数量(1)
专利数量(0)
An Accurate Nuclei Segmentation Algorithm in Pathological Image Based on Deep Semantic Network
一种基于深度语义网络的病理图像细胞核精确分割算法
- DOI:10.1109/access.2019.2934486
- 发表时间:2019-01-01
- 期刊:IEEE ACCESS
- 影响因子:3.9
- 作者:Pan, Xipeng;Li, Lingqiao;Yang, Huihua
- 通讯作者:Yang, Huihua
Attention-inception-based U-Net for retinal vessel segmentation with advanced residual
基于注意力感知的 U-Net,用于具有高级残差的视网膜血管分割
- DOI:10.1016/j.compeleceng.2021.107670
- 发表时间:2022-01-06
- 期刊:COMPUTERS & ELECTRICAL ENGINEERING
- 影响因子:4.3
- 作者:Wang, Huadeng;Xu, Guang;Luo, Xiaonan
- 通讯作者:Luo, Xiaonan
Multi-scale pathology image texture signature is a prognostic factor for resectable lung adenocarcinoma: a multi-center, retrospective study.
多尺度病理图像纹理特征是可切除肺腺癌的预后因素:一项多中心回顾性研究
- DOI:10.1186/s12967-022-03777-x
- 发表时间:2022-12-14
- 期刊:JOURNAL OF TRANSLATIONAL MEDICINE
- 影响因子:7.4
- 作者:Wang, Yumeng;Pan, Xipeng;Lin, Huan;Han, Chu;An, Yajun;Qiu, Bingjiang;Feng, Zhengyun;Huang, Xiaomei;Xu, Zeyan;Shi, Zhenwei;Chen, Xin;Li, Bingbing;Yan, Lixu;Lu, Cheng;Li, Zhenhui;Cui, Yanfen;Liu, Zaiyi;Liu, Zhenbing
- 通讯作者:Liu, Zhenbing
A Novel Ray-Casting Algorithm Using Dynamic Adaptive Sampling
一种使用动态自适应采样的新型光线投射算法
- DOI:10.1155/2020/8822624
- 发表时间:2020-10-14
- 期刊:WIRELESS COMMUNICATIONS & MOBILE COMPUTING
- 影响因子:--
- 作者:Wang,Huadeng;Xu,Guang;Luo,Xiaonan
- 通讯作者:Luo,Xiaonan
Multi-task generative adversarial learning for nuclei segmentation with dual attention and recurrent convolution
具有双重注意和循环卷积的细胞核分割的多任务生成对抗学习
- DOI:10.1016/j.bspc.2022.103558
- 发表时间:2022
- 期刊:Biomedical Signal Processing and Control
- 影响因子:5.1
- 作者:Huadeng Wang;Guang Xu;Xipeng Pan;Zhenbing Liu;Rushi Lan;Xiaonan Luo
- 通讯作者:Xiaonan Luo
数据更新时间:{{ journalArticles.updateTime }}
{{
item.title }}
{{ item.translation_title }}
- DOI:{{ item.doi || "--"}}
- 发表时间:{{ item.publish_year || "--" }}
- 期刊:{{ item.journal_name }}
- 影响因子:{{ item.factor || "--"}}
- 作者:{{ item.authors }}
- 通讯作者:{{ item.author }}
数据更新时间:{{ journalArticles.updateTime }}
{{ item.title }}
- 作者:{{ item.authors }}
数据更新时间:{{ monograph.updateTime }}
{{ item.title }}
- 作者:{{ item.authors }}
数据更新时间:{{ sciAawards.updateTime }}
{{ item.title }}
- 作者:{{ item.authors }}
数据更新时间:{{ conferencePapers.updateTime }}
{{ item.title }}
- 作者:{{ item.authors }}
数据更新时间:{{ patent.updateTime }}
其他文献
求解多目标优化问题的改进布谷鸟搜索算法
- DOI:--
- 发表时间:2015
- 期刊:浙江大学学报 (工学版)
- 影响因子:--
- 作者:杨辉华;谢谱模;张晓凤;马 巍;刘振丙
- 通讯作者:刘振丙
结合卷积神经网络和超像素聚类的细胞图像分割方法
- DOI:--
- 发表时间:2017
- 期刊:计算机应 用研究
- 影响因子:--
- 作者:杨金鑫;杨辉华;李灵巧;潘细鹏;刘振丙;周洁茜
- 通讯作者:周洁茜
基于多尺度残差神经网络的阿尔茨海默病诊断分类
- DOI:--
- 发表时间:2018
- 期刊:山东大学学报
- 影响因子:--
- 作者:刘振丙;方旭升;杨辉华;蓝如师
- 通讯作者:蓝如师
分割灰度不均匀图像水平集算法的 CUDA 实现
- DOI:--
- 发表时间:2017
- 期刊:计算机仿真
- 影响因子:--
- 作者:卓永;杨辉华;高春洋;刘振丙
- 通讯作者:刘振丙
一种新的最大间隔分类器的求解算法及其在测井岩性识别中的应用
- DOI:--
- 发表时间:2012
- 期刊:石油天然气学报
- 影响因子:--
- 作者:罗明璋;张翔;刘振丙
- 通讯作者:刘振丙
其他文献
{{
item.title }}
{{ item.translation_title }}
- DOI:{{ item.doi || "--" }}
- 发表时间:{{ item.publish_year || "--"}}
- 期刊:{{ item.journal_name }}
- 影响因子:{{ item.factor || "--" }}
- 作者:{{ item.authors }}
- 通讯作者:{{ item.author }}
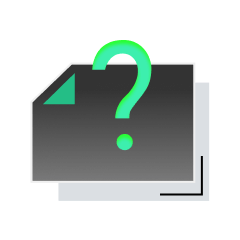
内容获取失败,请点击重试
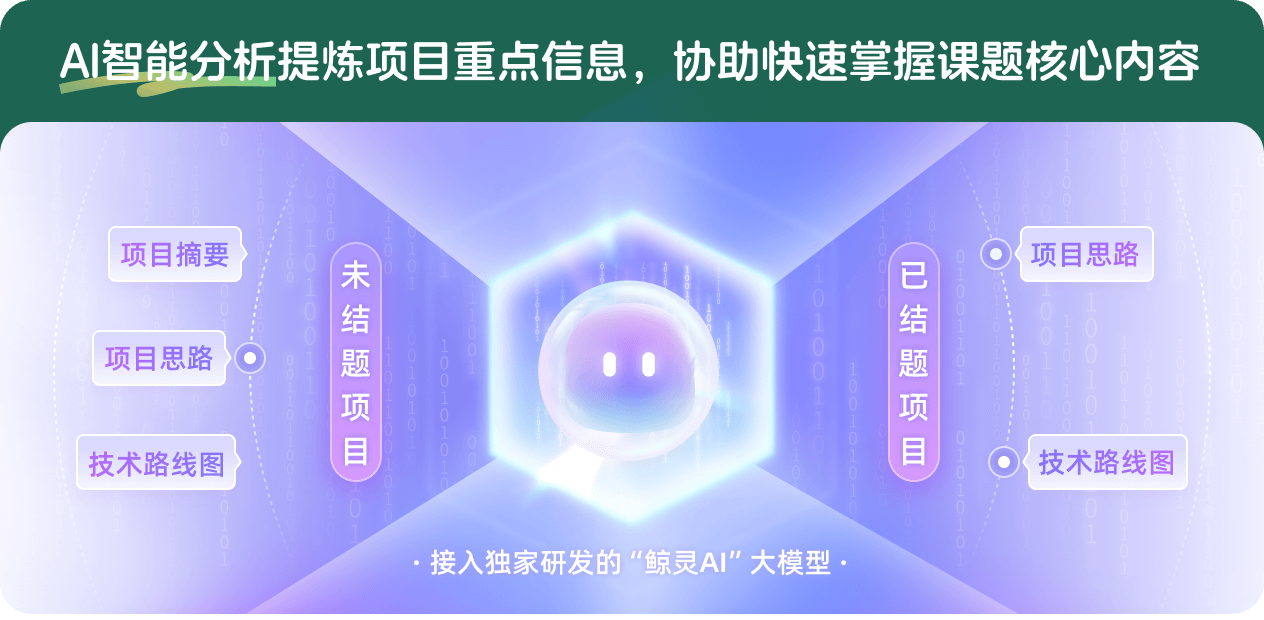
查看分析示例
此项目为已结题,我已根据课题信息分析并撰写以下内容,帮您拓宽课题思路:
AI项目摘要
AI项目思路
AI技术路线图
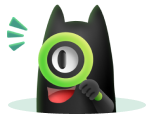
请为本次AI项目解读的内容对您的实用性打分
非常不实用
非常实用
1
2
3
4
5
6
7
8
9
10
您认为此功能如何分析更能满足您的需求,请填写您的反馈:
刘振丙的其他基金
基于联邦学习的多模态医学图像预测早期非小细胞肺癌术后复发风险的研究
- 批准号:
- 批准年份:2022
- 资助金额:52 万元
- 项目类别:面上项目
基于联邦学习的多模态医学图像预测早期非小细胞肺癌术后复发风险的研究
- 批准号:82272075
- 批准年份:2022
- 资助金额:52.00 万元
- 项目类别:面上项目
代价敏感的稀疏学习与距离度量学习方法研究
- 批准号:61562013
- 批准年份:2015
- 资助金额:38.0 万元
- 项目类别:地区科学基金项目
基于尺度化凸壳的最大间隔学习算法研究
- 批准号:61105004
- 批准年份:2011
- 资助金额:22.0 万元
- 项目类别:青年科学基金项目
相似国自然基金
{{ item.name }}
- 批准号:{{ item.ratify_no }}
- 批准年份:{{ item.approval_year }}
- 资助金额:{{ item.support_num }}
- 项目类别:{{ item.project_type }}
相似海外基金
{{
item.name }}
{{ item.translate_name }}
- 批准号:{{ item.ratify_no }}
- 财政年份:{{ item.approval_year }}
- 资助金额:{{ item.support_num }}
- 项目类别:{{ item.project_type }}