克罗内克积协方差和相关系数模型的估计——偏均值法和二次型法
项目介绍
AI项目解读
基本信息
- 批准号:71903034
- 项目类别:青年科学基金项目
- 资助金额:19.0万
- 负责人:
- 依托单位:
- 学科分类:G0301.计量经济与经济统计
- 结题年份:2022
- 批准年份:2019
- 项目状态:已结题
- 起止时间:2020-01-01 至2022-12-31
- 项目参与者:--
- 关键词:
项目摘要
Covariance (and correlation) matrices measure how different objects are related; they are crucial in many fields. Against the backdrop of big data, the matrix dimension n relative to the sample size T can no longer be ignored. When n/T≤1 but not close to 0, we call it the large dimension; when n/T>1, we call it the high dimension. Traditional methods to estimate covariance matrices cannot be applied in the large/high dimension. Our working paper Hafner, Linton and Tang (2018) proposed a Kronecker product model to estimate covariance matrices, and provided methods to estimate the model in the large dimension. This grant application stands on the shoulder of Hafner, Linton and Tang (2018) to answer the question - How to estimate the Kronecker product model in the high dimension? As the theoretical properties of the methods of Hafner, Linton and Tang (2018) are unknown in the high dimension, this grant application proposes partial means and quadratic form methods to estimate the Kronecker product model in the high dimension. We will study their high-dimensional properties and use them to construct a high-dimensional Lagrange multiplier test. Last, we estimate the covariance matrix of Chinese stock returns, form portfolios, and investigate the performances.
协方差(和相关系数)矩阵度量个体之间的相关性,在很多领域中很重要。在大数据背景下,矩阵维度n相较之样本容量T是不可忽略的。当n/T≤1但不接近0,称为大维;当n/T>1,称为高维。传统估计协方差矩阵的方法在大/高维下并不适用。如何在大/高维下估计协方差矩阵一直是学者们致力研究的问题。我们在论文Hafner, Linton and Tang (2018)(简称HLT18)里对协方差矩阵提出克罗内克积模型,并给出在大维下估计模型的方法。本项目以HLT18为基石,进一步回答如何在高维下估计克罗内克积模型。由于HLT18的方法的高维性质尚属未知,本项目提出偏均值和二次型两种全新的估计法来完成高维下克罗内克积模型的估计。本项目研究这两种估计法的高维性质,并用它们构建高维拉格朗日检验。最后,我们估计沪深股票收益的协方差矩阵,构建资产组合,检验其量化投资的效果。
结项摘要
协方差矩阵在诸多领域中都很重要。假设协方差矩阵的维度为n,样本容量为T。实证应用中的n,相对于T,是比较大的。如果n/T不接近0,但小于1,我们称为大维;如果n/T>1,我们称为高维。项目负责人2020年发表在Journal of Econometrics上的论文(简称文1)对协方差矩阵提出克罗内克积模型,是领域内第一篇在大维下考虑克罗内克积模型的研究。在大维下,我们证明了所提出的估计量的收敛速度和渐近正态性。..但文1并没有回答如何在高维下估计克罗内克积模型,该项目填补了这一空白。该项目提出一个新的估计法,二次型法,来完成高维下克罗内克积模型的估计。二次型估计量对样本协方差矩阵的元素取平均,在一个弱相关的条件下,我们得到了一个文1得不到的收敛速度。另外,我们考虑了关于高维数据均值的假设检验。在原假设和局部备择假设下,我们得到了拉格朗日和Wald检验的极限分布。在随机模拟实验里,在克罗内克积模型成立和不成立两种情况下,我们的估计量性能都挺好。..该项目的核心成果于2022年发表在国内外权威期刊Econometric Theory (SCIE, SSCI)上。因为项目负责人在克罗内克积领域上的积累,项目负责人撰写了一篇评论发表在国内外顶级期刊Journal of the American Statistical Association (SCIE)上。在应用前景方面,任何需要估计协方差矩阵的领域都可以考虑使用克罗内克积模型,并用二次型估计量来估计。例如,我们可以用克罗内克积模型估计海量股票的日收益率的协方差矩阵,由此来构建最小方差资产组合。另外,以本项目为依托,项目负责人培养了6名研究生(3名专硕,3名工商管理硕士),他们已经顺利毕业。
项目成果
期刊论文数量(2)
专著数量(0)
科研奖励数量(0)
会议论文数量(0)
专利数量(0)
Comment on “Factor Models for High-Dimensional Tensor Time Series” by Rong Chen, Dan Yang, and Cun-Hui Zhang
对陈榕、杨丹和张存辉的“高维张量时间序列的因子模型”的评论
- DOI:10.1080/01621459.2021.2018328
- 发表时间:2022-01
- 期刊:Journal of the American Statistical Association
- 影响因子:3.7
- 作者:Oliver B. Linton;Haihan Tang
- 通讯作者:Haihan Tang
数据更新时间:{{ journalArticles.updateTime }}
{{
item.title }}
{{ item.translation_title }}
- DOI:{{ item.doi || "--"}}
- 发表时间:{{ item.publish_year || "--" }}
- 期刊:{{ item.journal_name }}
- 影响因子:{{ item.factor || "--"}}
- 作者:{{ item.authors }}
- 通讯作者:{{ item.author }}
数据更新时间:{{ journalArticles.updateTime }}
{{ item.title }}
- 作者:{{ item.authors }}
数据更新时间:{{ monograph.updateTime }}
{{ item.title }}
- 作者:{{ item.authors }}
数据更新时间:{{ sciAawards.updateTime }}
{{ item.title }}
- 作者:{{ item.authors }}
数据更新时间:{{ conferencePapers.updateTime }}
{{ item.title }}
- 作者:{{ item.authors }}
数据更新时间:{{ patent.updateTime }}
其他文献
其他文献
{{
item.title }}
{{ item.translation_title }}
- DOI:{{ item.doi || "--" }}
- 发表时间:{{ item.publish_year || "--"}}
- 期刊:{{ item.journal_name }}
- 影响因子:{{ item.factor || "--" }}
- 作者:{{ item.authors }}
- 通讯作者:{{ item.author }}
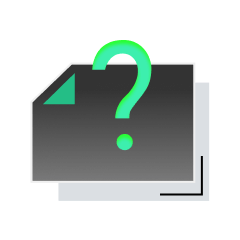
内容获取失败,请点击重试
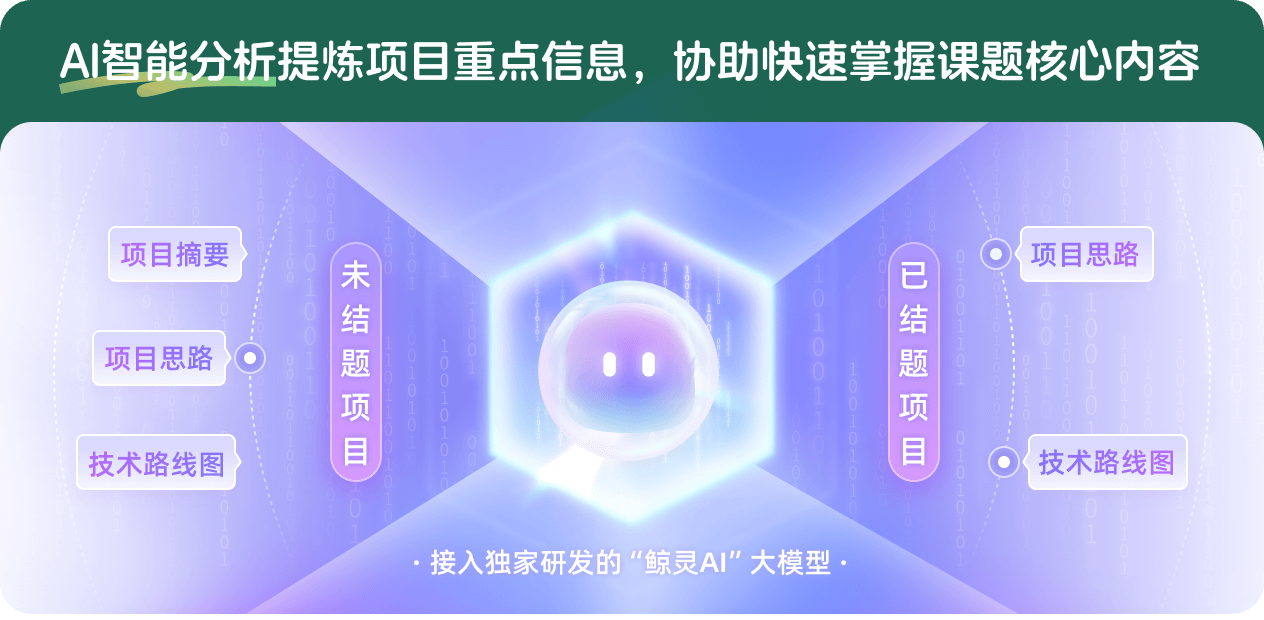
查看分析示例
此项目为已结题,我已根据课题信息分析并撰写以下内容,帮您拓宽课题思路:
AI项目摘要
AI项目思路
AI技术路线图
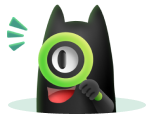
请为本次AI项目解读的内容对您的实用性打分
非常不实用
非常实用
1
2
3
4
5
6
7
8
9
10
您认为此功能如何分析更能满足您的需求,请填写您的反馈:
相似国自然基金
{{ item.name }}
- 批准号:{{ item.ratify_no }}
- 批准年份:{{ item.approval_year }}
- 资助金额:{{ item.support_num }}
- 项目类别:{{ item.project_type }}
相似海外基金
{{
item.name }}
{{ item.translate_name }}
- 批准号:{{ item.ratify_no }}
- 财政年份:{{ item.approval_year }}
- 资助金额:{{ item.support_num }}
- 项目类别:{{ item.project_type }}