基于复合分位数回归和最大秩相关想法的ROC回归曲线估计
项目介绍
AI项目解读
基本信息
- 批准号:11301031
- 项目类别:青年科学基金项目
- 资助金额:22.0万
- 负责人:
- 依托单位:
- 学科分类:A0402.统计推断与统计计算
- 结题年份:2016
- 批准年份:2013
- 项目状态:已结题
- 起止时间:2014-01-01 至2016-12-31
- 项目参与者:梁宝生; 邱燕萍;
- 关键词:
项目摘要
The covariate-specific receiver operating characteristic curve or ROC regression is frequently used to evaluate the classification accuracy of a diagnostic test when it is associated with certain covariates. Indirect modeling of ROC regression is widely discussed in the literature. This method first builds a disease-specific regression model between test results and covariates as well as disease status, and estimates model parameters based on quasilikelihood method or local polynomial regression; then it estimates the targeted ROC curve based on nonparametric or kernel smoothing estimates of the disease-specific survival function using fitted residuals obtained previously. Considering the limitations of this method (e.g.: depending heavily on properties of the random errors, and is sensitive to outliers), we propose in this project a new series of methods for ROC regression based on the ideas of composite quantile regression and maximum rank correlation. We discuss these methods both in the absense or presence of missing data at random. These include missing test result, missing covariates, missing gold standard, or a mixture of them. To facilitate the use and comparison of different regression methods, we also propose a new formulation for a class of traditional location-scale model. Under the new framework,the ROC regression curve is much simpler than it was under the old framework. Furthermore, it is more convenient to compare the asymptotic variances among the different methods. Due to the complexity of the estimated ROC curve expression, we shall establish the asymptotic results of the proposed estimators, based on M-estimation theories and empirical process method. We shall conduct extensive numerical simulations to compare the finite sample performances of different methods. We shall also apply the different procedures in a real example from the National Alzheimer's Coordinating Center.
受试者操作特征(ROC)回归曲线广泛用于评估连续型诊断检验的分类精度。文献上常使用间接建模方法估计ROC回归曲线:首先对诊断检验结果关于协变量和疾病状态建立位置刻度模型,并通过拟似然或局部多项式回归估计模型参数;然后基于获得的拟合残差使用非参或核光滑方法估计目标ROC曲线。这类方法对模型随机误差分布有很强的依赖性,且对观测数据中的异常值特别敏感。本课题中,我们拟使用复合分位数及最大秩相关等技巧估计ROC回归曲线。我们分别考虑数据完全观测和含随机缺失两种情况。为便于使用上述方法,我们针对一类参数位置刻度模型提出一种新的模型描述方法。在新的模型描述框架下,目标ROC回归曲线形式更加简洁,且更便于理论上比较各种估计的渐近方差。考虑到ROC曲线估计表达式的复杂性,我们拟借助M-估计理论及经验过程知识证明估计量的渐近正态性。我们拟通过大量数值模拟及老年痴呆症方面的实际数据考察各类方法的有限样本表现。
结项摘要
受试者操作特征(ROC)回归曲线是评估诊断检验分类精度的一种基本工具。本项目中,我们分别使用复合分位数回归、加权秩回归等技巧估计ROC回归曲线。我们分别考虑数据完全观测和含随机缺失两种情况。为便于使用上述估计方法, 我们对一类参数位置刻度模型提出了一种新的模型描述方法。在新的模型描述框架下,目标ROC回归曲线形式更加简洁,且更便于理论上比较各种估计的渐近性质。我们借助M-估计理论及经验过程知识证明了估计量的渐近性质,并通过大量数值模拟及老年痴呆症方面的实际数据考察了所提方法的有限样本表现。本项目提出的“位置刻度模型的重参数化”,将对基于不同方法位置刻度模型参数估计效率的渐近比较提供统一框架。
项目成果
期刊论文数量(2)
专著数量(0)
科研奖励数量(0)
会议论文数量(0)
专利数量(0)
多元生存数据加法模型的广义矩估计
- DOI:--
- 发表时间:2016
- 期刊:Scandinavian Journal of Statistics
- 影响因子:1
- 作者:Li; Hui;Duan; Xiaogang;Yin; Guosheng
- 通讯作者:Guosheng
二分类自变量随机缺失时回归模型的最小二乘与经验似然融合估计
- DOI:--
- 发表时间:2016
- 期刊:Science China Mathematics
- 影响因子:--
- 作者:段小刚;王植
- 通讯作者:王植
数据更新时间:{{ journalArticles.updateTime }}
{{
item.title }}
{{ item.translation_title }}
- DOI:{{ item.doi || "--"}}
- 发表时间:{{ item.publish_year || "--" }}
- 期刊:{{ item.journal_name }}
- 影响因子:{{ item.factor || "--"}}
- 作者:{{ item.authors }}
- 通讯作者:{{ item.author }}
数据更新时间:{{ journalArticles.updateTime }}
{{ item.title }}
- 作者:{{ item.authors }}
数据更新时间:{{ monograph.updateTime }}
{{ item.title }}
- 作者:{{ item.authors }}
数据更新时间:{{ sciAawards.updateTime }}
{{ item.title }}
- 作者:{{ item.authors }}
数据更新时间:{{ conferencePapers.updateTime }}
{{ item.title }}
- 作者:{{ item.authors }}
数据更新时间:{{ patent.updateTime }}
其他文献
Quantile Regression under Local Misspecification
局部错误指定下的分位数回归
- DOI:10.1007/s10255-020-0973-9
- 发表时间:2020
- 期刊:Acta Mathematicae Applicatae Sinica, English Series
- 影响因子:--
- 作者:段小刚;王启华
- 通讯作者:王启华
基于Copula函数的北京强降水频率及危险性分析
- DOI:--
- 发表时间:2016
- 期刊:气象学报
- 影响因子:--
- 作者:曹伟华;梁旭东;赵晗萍;段小刚;张自银
- 通讯作者:张自银
HBeAg转阴与HBV基因型分布和YMDD变异位点的相关性分析
- DOI:--
- 发表时间:2014
- 期刊:检验医学与临床
- 影响因子:--
- 作者:王艳艳;曲远青;段小刚;吴丽娟
- 通讯作者:吴丽娟
其他文献
{{
item.title }}
{{ item.translation_title }}
- DOI:{{ item.doi || "--" }}
- 发表时间:{{ item.publish_year || "--"}}
- 期刊:{{ item.journal_name }}
- 影响因子:{{ item.factor || "--" }}
- 作者:{{ item.authors }}
- 通讯作者:{{ item.author }}
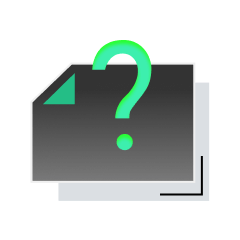
内容获取失败,请点击重试
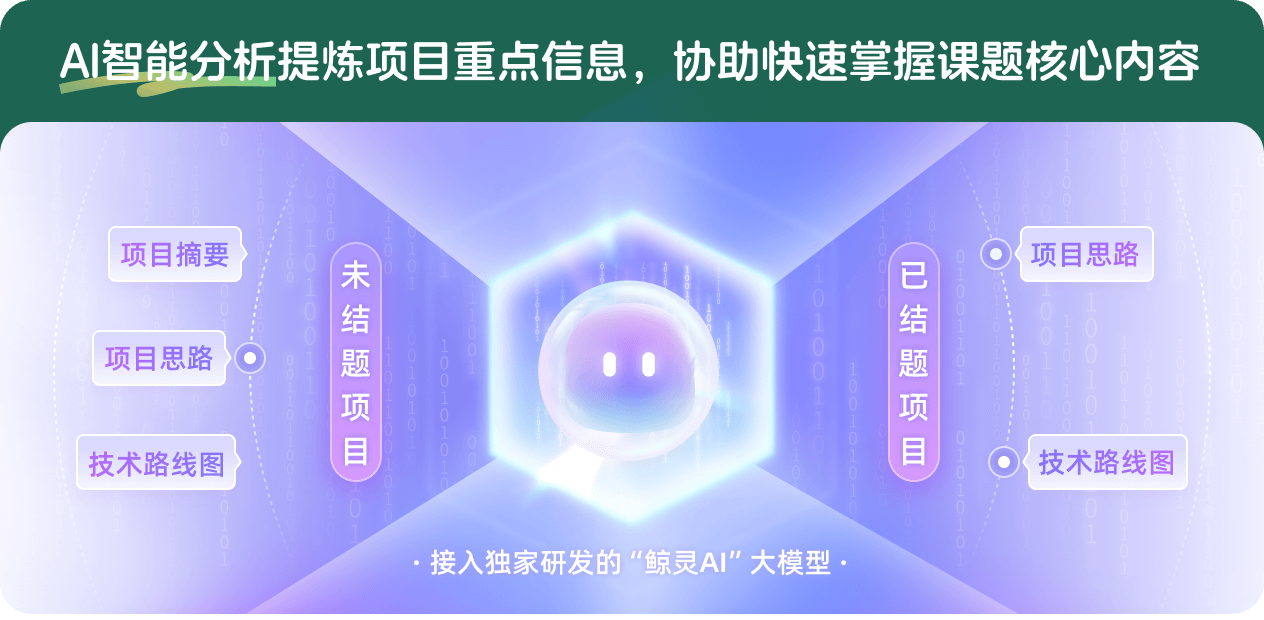
查看分析示例
此项目为已结题,我已根据课题信息分析并撰写以下内容,帮您拓宽课题思路:
AI项目摘要
AI项目思路
AI技术路线图
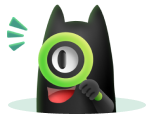
请为本次AI项目解读的内容对您的实用性打分
非常不实用
非常实用
1
2
3
4
5
6
7
8
9
10
您认为此功能如何分析更能满足您的需求,请填写您的反馈:
段小刚的其他基金
基于再拟合的多模型融合推断:理论及应用
- 批准号:11771049
- 批准年份:2017
- 资助金额:48.0 万元
- 项目类别:面上项目
相似国自然基金
{{ item.name }}
- 批准号:{{ item.ratify_no }}
- 批准年份:{{ item.approval_year }}
- 资助金额:{{ item.support_num }}
- 项目类别:{{ item.project_type }}
相似海外基金
{{
item.name }}
{{ item.translate_name }}
- 批准号:{{ item.ratify_no }}
- 财政年份:{{ item.approval_year }}
- 资助金额:{{ item.support_num }}
- 项目类别:{{ item.project_type }}