有机单分子膜/二维材料杂化超晶格的表界面构筑及光电性能研究
项目介绍
AI项目解读
基本信息
- 批准号:22302073
- 项目类别:青年科学基金项目
- 资助金额:30万
- 负责人:
- 依托单位:
- 学科分类:B.化学科学部
- 结题年份:
- 批准年份:2023
- 项目状态:未结题
- 起止时间:2023至
- 项目参与者:王灿;
- 关键词:
项目摘要
结项摘要
项目成果
期刊论文数量(0)
专著数量(0)
科研奖励数量(0)
会议论文数量(0)
专利数量(0)
数据更新时间:{{ journalArticles.updateTime }}
{{
item.title }}
{{ item.translation_title }}
- DOI:{{ item.doi || "--"}}
- 发表时间:{{ item.publish_year || "--" }}
- 期刊:{{ item.journal_name }}
- 影响因子:{{ item.factor || "--"}}
- 作者:{{ item.authors }}
- 通讯作者:{{ item.author }}
数据更新时间:{{ journalArticles.updateTime }}
{{ item.title }}
- 作者:{{ item.authors }}
数据更新时间:{{ monograph.updateTime }}
{{ item.title }}
- 作者:{{ item.authors }}
数据更新时间:{{ sciAawards.updateTime }}
{{ item.title }}
- 作者:{{ item.authors }}
数据更新时间:{{ conferencePapers.updateTime }}
{{ item.title }}
- 作者:{{ item.authors }}
数据更新时间:{{ patent.updateTime }}
其他文献
我国奶牛饲养的损益点测算和竞争潜力分析
- DOI:--
- 发表时间:--
- 期刊:中国奶牛
- 影响因子:--
- 作者:王灿;郭善民;唐华仓;余航;吴一平;马恒运;赵翠萍
- 通讯作者:赵翠萍
前列腺癌恩杂鲁胺耐药细胞株的建立及相关lncRNA和mRNA的筛选
- DOI:10.13263/j.cnki.nja.2018.02.004
- 发表时间:2018
- 期刊:中华男科学杂志
- 影响因子:--
- 作者:关翰;凌志新;方芳;毛溧凯;尤宗昊;王灿;陈恕求;许斌;陈明
- 通讯作者:陈明
自由贸易协定与全球价值链嵌入:对FTA深度作用的考察
- DOI:10.13510/j.cnki.jit.2019.02.005
- 发表时间:2019
- 期刊:国际贸易问题
- 影响因子:--
- 作者:韩剑;王灿
- 通讯作者:王灿
个体时空行为的规律性与可预测性研究——以上海市居民工作日活动为例
- DOI:10.18306/dlkxjz.2021.03.007
- 发表时间:2021
- 期刊:地理科学进展
- 影响因子:--
- 作者:王德;谢栋灿;王灿;殷振轩
- 通讯作者:殷振轩
青海喇家遗址齐家文化时期黍粟农业的植硅体证据
- DOI:--
- 发表时间:2015
- 期刊:第四纪研究
- 影响因子:--
- 作者:王灿;吕厚远;张健平;叶茂林;蔡林海
- 通讯作者:蔡林海
其他文献
{{
item.title }}
{{ item.translation_title }}
- DOI:{{ item.doi || "--" }}
- 发表时间:{{ item.publish_year || "--"}}
- 期刊:{{ item.journal_name }}
- 影响因子:{{ item.factor || "--" }}
- 作者:{{ item.authors }}
- 通讯作者:{{ item.author }}
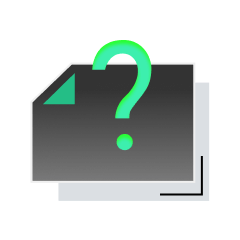
内容获取失败,请点击重试
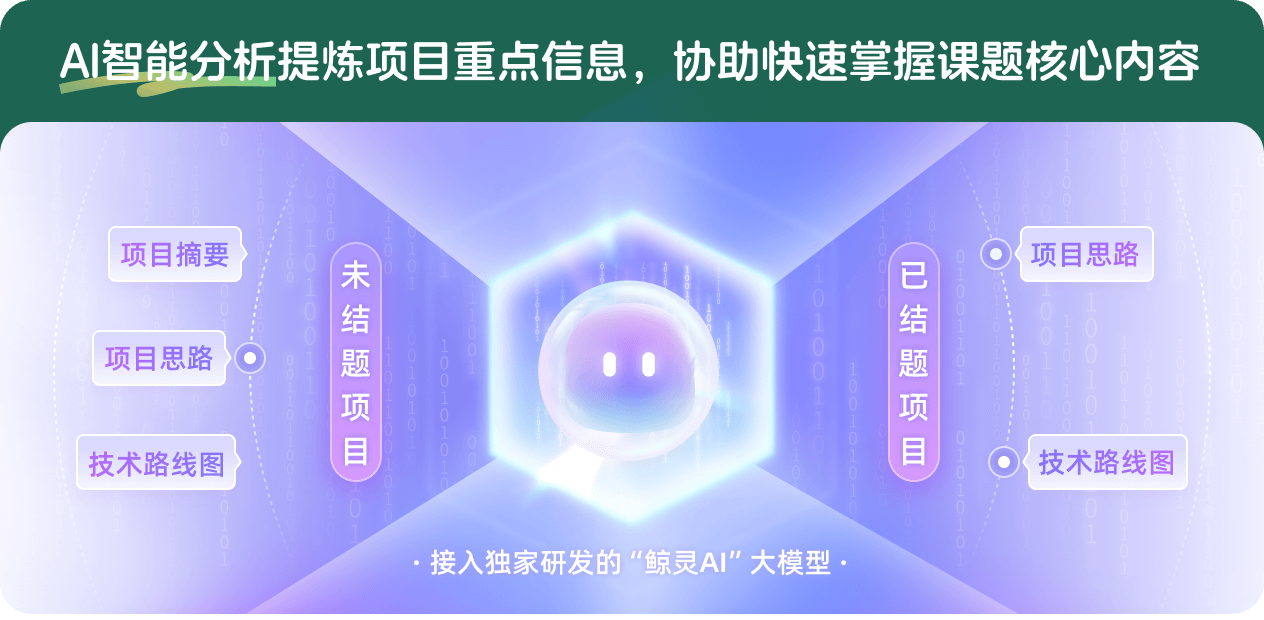
查看分析示例
此项目为未结题,我已根据课题信息分析并撰写以下内容,帮您拓宽课题思路:
AI项目摘要
AI项目思路
AI技术路线图
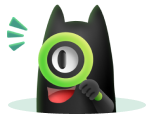
请为本次AI项目解读的内容对您的实用性打分
非常不实用
非常实用
1
2
3
4
5
6
7
8
9
10
您认为此功能如何分析更能满足您的需求,请填写您的反馈:
相似国自然基金
{{ item.name }}
- 批准号:{{ item.ratify_no }}
- 批准年份:{{ item.approval_year }}
- 资助金额:{{ item.support_num }}
- 项目类别:{{ item.project_type }}
相似海外基金
{{
item.name }}
{{ item.translate_name }}
- 批准号:{{ item.ratify_no }}
- 财政年份:{{ item.approval_year }}
- 资助金额:{{ item.support_num }}
- 项目类别:{{ item.project_type }}