基于形式化方法的实时系统互操作性测试研究
项目介绍
AI项目解读
基本信息
- 批准号:60572082
- 项目类别:面上项目
- 资助金额:20.0万
- 负责人:
- 依托单位:
- 学科分类:F0104.通信网络
- 结题年份:2008
- 批准年份:2005
- 项目状态:已结题
- 起止时间:2006-01-01 至2008-12-31
- 项目参与者:王之梁; 施新刚; 刘岩; 喻星; 李天;
- 关键词:
项目摘要
在未来的大型分布式系统和计算机通信系统中,实时系统是其中最重要最基本的组成元素。实时系统之间的通信问题是保证分布式系统和通讯系统正常运行最基本最重要的问题。对于实时系统的互操作性测试研究是了解实时系统通信问题最直接最有效的方法,但对它的研究仅仅处于起步阶段。因此,对于实时系统的互操作性测试研究不仅重要而且必需。.本项目的研究目标是利用形式化方法研究什么是实时系统互操作性测试、如何实现、如何提供服务以及什么是实时系统互操作性测试的关键问题这四个科学问题。.整个理论框架的出发点是实时系统的形式化描述模型,在此基础上,研究以下几个相关的关键技术:测试的理论基础,测试架构和测试方法,测试生成技术及测试覆盖评价,测试集描述法,基于该测试集描述法的测试执行技术。
结项摘要
项目成果
期刊论文列表
专著列表
科研奖励列表
会议论文列表
专利列表
数据更新时间:{{ journalArticles.updateTime }}
{{
item.title }}
{{ item.translation_title }}
- DOI:{{ item.doi || "--"}}
- 发表时间:{{ item.publish_year || "--" }}
- 期刊:{{ item.journal_name }}
- 影响因子:{{ item.factor || "--"}}
- 作者:{{ item.authors }}
- 通讯作者:{{ item.author }}
数据更新时间:{{ journalArticles.updateTime }}
{{ item.title }}
- 作者:{{ item.authors }}
数据更新时间:{{ monograph.updateTime }}
{{ item.title }}
- 作者:{{ item.authors }}
数据更新时间:{{ sciAawards.updateTime }}
{{ item.title }}
- 作者:{{ item.authors }}
数据更新时间:{{ conferencePapers.updateTime }}
{{ item.title }}
- 作者:{{ item.authors }}
数据更新时间:{{ patent.updateTime }}
其他文献
春季黄、渤海沉积物中CH4和N2O的垂向分布特征研究
- DOI:10.13745/j.esf.sf.2021.9.12
- 发表时间:2021
- 期刊:地学前缘
- 影响因子:--
- 作者:李思琦;陈烨;尹霞;臧昆鹏;甄毓
- 通讯作者:甄毓
基于遗传算法的混合软件定义网络路由节能算法
- DOI:--
- 发表时间:2020
- 期刊:计算机科学
- 影响因子:--
- 作者:张举;王浩;罗舒婷;耿海军;尹霞
- 通讯作者:尹霞
刺老苞根皮黄酮对骨折模型大鼠骨质代谢的影响研究
- DOI:--
- 发表时间:--
- 期刊:上海中医药杂志
- 影响因子:--
- 作者:裴凌鹏;郑玲玲;尹霞;白岩;李志勇
- 通讯作者:李志勇
非水相酶促合成不同链长的对香豆酸酯及其自由基清除能力
- DOI:10.13982/j.mfst.1673-9078.2021.7.0217
- 发表时间:2021
- 期刊:现代食品科技
- 影响因子:--
- 作者:尹霞;辛璇;李晓凤
- 通讯作者:李晓凤
基于段路由的单节点故障路由保护算法
- DOI:--
- 发表时间:2018
- 期刊:清华大学学报(自然科学版)
- 影响因子:--
- 作者:耿海军;刘洁琦;尹霞
- 通讯作者:尹霞
其他文献
{{
item.title }}
{{ item.translation_title }}
- DOI:{{ item.doi || "--" }}
- 发表时间:{{ item.publish_year || "--"}}
- 期刊:{{ item.journal_name }}
- 影响因子:{{ item.factor || "--" }}
- 作者:{{ item.authors }}
- 通讯作者:{{ item.author }}
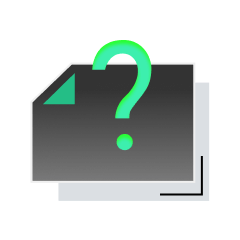
内容获取失败,请点击重试
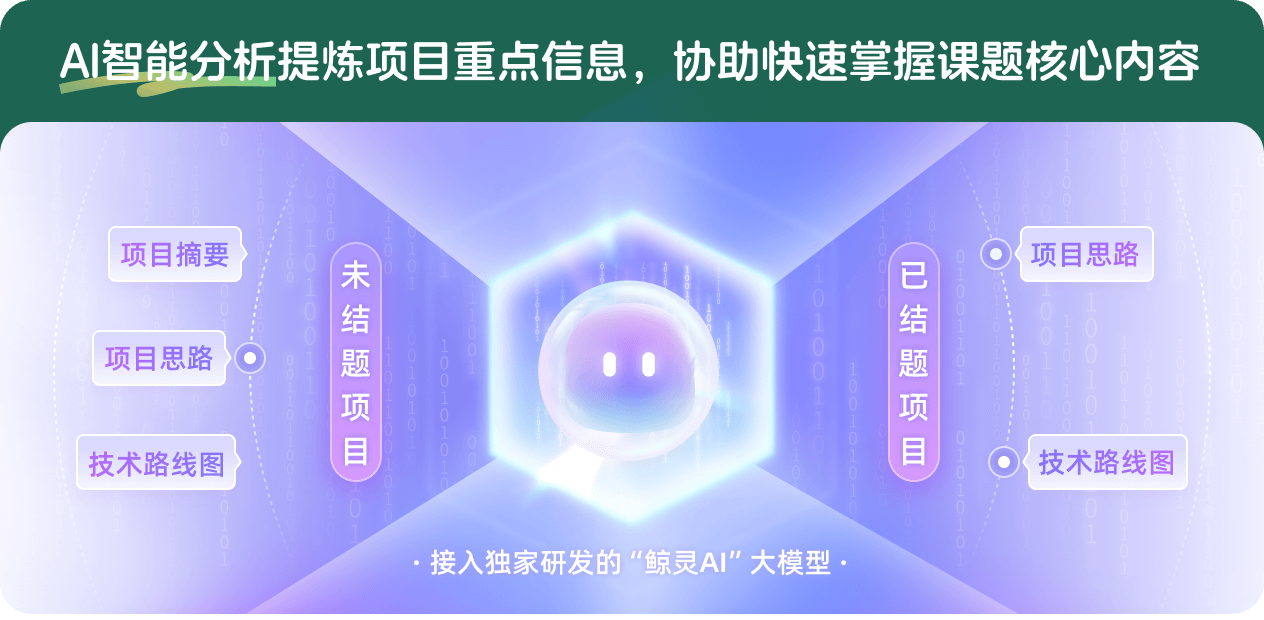
查看分析示例
此项目为已结题,我已根据课题信息分析并撰写以下内容,帮您拓宽课题思路:
AI项目摘要
AI项目思路
AI技术路线图
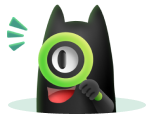
请为本次AI项目解读的内容对您的实用性打分
非常不实用
非常实用
1
2
3
4
5
6
7
8
9
10
您认为此功能如何分析更能满足您的需求,请填写您的反馈:
尹霞的其他基金
下一代互联网IPv6协议的测试理论研究
- 批准号:60102009
- 批准年份:2001
- 资助金额:4.0 万元
- 项目类别:青年科学基金项目
相似国自然基金
{{ item.name }}
- 批准号:{{ item.ratify_no }}
- 批准年份:{{ item.approval_year }}
- 资助金额:{{ item.support_num }}
- 项目类别:{{ item.project_type }}
相似海外基金
{{
item.name }}
{{ item.translate_name }}
- 批准号:{{ item.ratify_no }}
- 财政年份:{{ item.approval_year }}
- 资助金额:{{ item.support_num }}
- 项目类别:{{ item.project_type }}