基于压缩感知的核磁共振成像问题驱动的应用数学研究
项目介绍
AI项目解读
基本信息
- 批准号:11571325
- 项目类别:面上项目
- 资助金额:55.0万
- 负责人:
- 依托单位:
- 学科分类:A0504.微分方程数值解
- 结题年份:2019
- 批准年份:2015
- 项目状态:已结题
- 起止时间:2016-01-01 至2019-12-31
- 项目参与者:常谦顺; 王武; 朱先进; 张彬; 于欣妍; 刘晓曼; 李浩; 牛秀秀;
- 关键词:
项目摘要
This project is based on National Program for Medium- and Long-Term Scientific and Technological Development and 12th Five-Year Plan for the Development of the National Natural Science Fund of China. It is a research on the mathematical problems from practical applications, and also a basic research on the key Compressive Sensing MRI (CS-MRI)-driven problems for high-performance computing and data processing. The contents of this project are the three core issues in compressive sensing MRI, i.e. the sparse representation for MR images,the K-Space sampling optimal design and nonlinear reconstruction of MR images. The research of these issues is used to improve the speed of magnetic resonance imaging. The object of this project is to solve the challenging problems in CS-MRI, and to promote the application and development of applied mathematics in the field of medical imaging. The first innovative point of this project is to present adaptive dictionary sparse and global regularization sparse representation methods in MR images transforms. The second innovative point is to design feasible K-Space sampling modes in order to exploit the performance of MR equipment. The third innovative point is to establish fast, efficient, stable and general nonlinear reconstruction methods of MR images. The fourth innovative point is to provide the more total study about applied mathematics driven by CS-MRI problems.
本项目是依据《国家中长期科学与技术发展规划纲要》和国家自然科学基金“十二五”发展规划,从实际应用中凝练出的应用数学问题研究,是基于压缩感知的核磁共振成像(Compressive Sensing Magnetic Resonance Imaging,CS-MRI)问题驱动的高性能计算和数据处理的关键问题研究。具体的研究内容是面向核磁共振成像时间长的实际问题,就基于压缩感知的核磁共振成像中的三个核心问题:MR图像的稀疏表示、K-Space抽样最优设计和MR图像的非线性重建进行应用数学研究,解决核磁共振成像时间慢这一挑战性问题,推动应用数学在医学成像领域中的应用和发展。本项目的创新之处在于给出MR图像的自适应字典稀疏和全局正则化稀疏表示;设计能够发挥MR硬件效能的实际可行的K-Space抽样模式;构造快速、有效、稳定和普适的MR图像非线性重建方法;给出较全面的CS-MRI问题驱动的应用数学研究。
结项摘要
给出了改进的L1/L2联合正则化图像复原模型及其求解的数值方法,证明了求解方法的收敛性。发展了新的图像复原的非线性扩散模型,证明了该模型弱解的存在性,给出了求解模型的加性分裂方法。研究出用于图像去噪的增广拉格朗日乘子方法与对称Red-Black 高斯-赛德尔方法的组合方法和相应的数学模型及其数值求解方法。研究出一种基于Tikhonov正则化的图像盲复原方法。系统整理了傅里叶变换的图像复原算法、基于共轭梯度法的图像复原、基于全变差的图像正则化复原算法、Bregman算法、Bregman分裂算法、基于偏微分方程的图像复原算法、变指数函数空间的图像复原算法、图像去雾和基于深度神经网络的单视觉实时避障算法等系列研究成果。研究出一种能够从再压缩图像中对迹特征进行重采样的卷积神经网络(Convolutional Neural Networks, CNN)学习方法,这一学习方法可用于图像篡改的辨识。
项目成果
期刊论文数量(5)
专著数量(1)
科研奖励数量(0)
会议论文数量(1)
专利数量(0)
Fast Algorithm for Image Denosing with Different Boundary Conditions
不同边界条件下图像去噪的快速算法
- DOI:--
- 发表时间:2017
- 期刊:Journal of the Franklin Institute
- 影响因子:--
- 作者:Xiaole Zhang;Yuying Shi;zhifengoang@163.com;Yonggui Zhu
- 通讯作者:Yonggui Zhu
A Fast Linearised Augmented Lagrangian Method for a Mean Curvature Based Model
基于平均曲率模型的快速线性增广拉格朗日方法
- DOI:10.4208/eajam.010817.160218
- 发表时间:2018-06
- 期刊:East Asian Journal on Applied Mathematics
- 影响因子:1.2
- 作者:Jun Zhang;Chengzhi Deng;Yuying Shi;Shengqian Wang;Yonggui Zhu
- 通讯作者:Yonggui Zhu
Resampling detection of recompressed images via dual-stream convolutional neural network
通过双流卷积神经网络对重压缩图像进行重采样检测
- DOI:10.3934/mbe.2019253
- 发表时间:2019
- 期刊:Mathematical Biosciences and Engineering
- 影响因子:2.6
- 作者:Cao Gang;Zhou Antao;Huang Xianglin;Song Gege;Yang Lifang;Zhu Yonggui
- 通讯作者:Zhu Yonggui
数据更新时间:{{ journalArticles.updateTime }}
{{
item.title }}
{{ item.translation_title }}
- DOI:{{ item.doi || "--"}}
- 发表时间:{{ item.publish_year || "--" }}
- 期刊:{{ item.journal_name }}
- 影响因子:{{ item.factor || "--"}}
- 作者:{{ item.authors }}
- 通讯作者:{{ item.author }}
数据更新时间:{{ journalArticles.updateTime }}
{{ item.title }}
- 作者:{{ item.authors }}
数据更新时间:{{ monograph.updateTime }}
{{ item.title }}
- 作者:{{ item.authors }}
数据更新时间:{{ sciAawards.updateTime }}
{{ item.title }}
- 作者:{{ item.authors }}
数据更新时间:{{ conferencePapers.updateTime }}
{{ item.title }}
- 作者:{{ item.authors }}
数据更新时间:{{ patent.updateTime }}
其他文献
其他文献
{{
item.title }}
{{ item.translation_title }}
- DOI:{{ item.doi || "--" }}
- 发表时间:{{ item.publish_year || "--"}}
- 期刊:{{ item.journal_name }}
- 影响因子:{{ item.factor || "--" }}
- 作者:{{ item.authors }}
- 通讯作者:{{ item.author }}
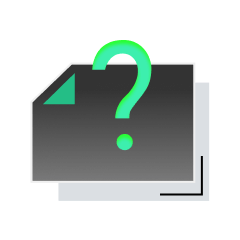
内容获取失败,请点击重试
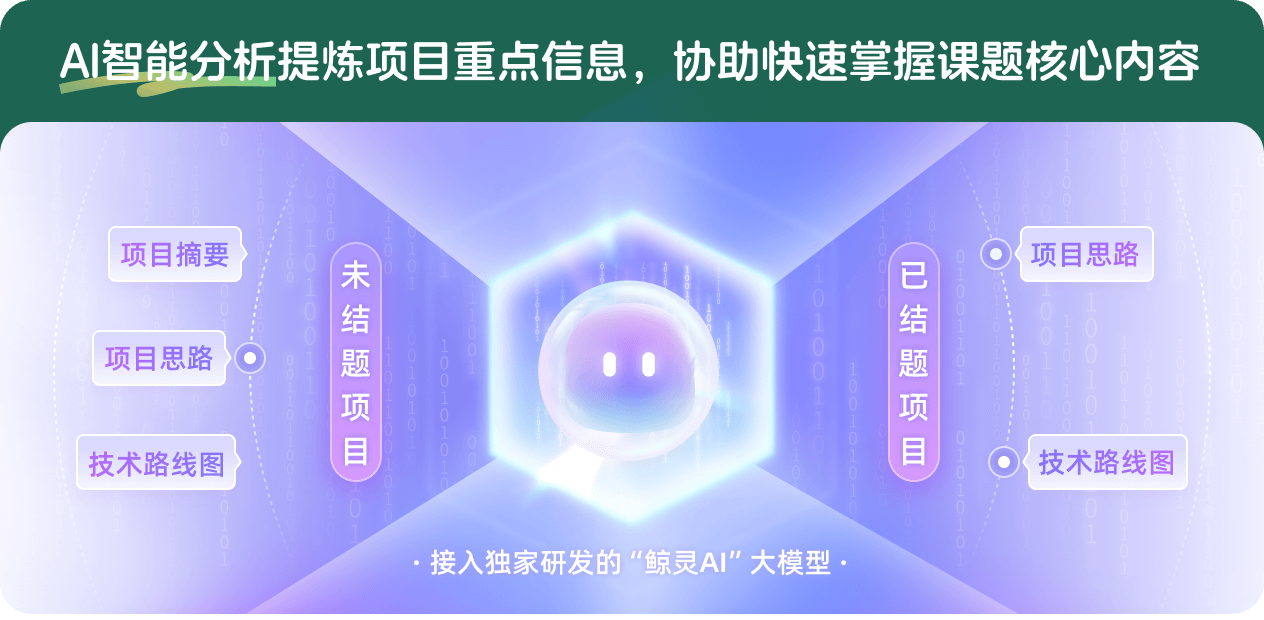
查看分析示例
此项目为已结题,我已根据课题信息分析并撰写以下内容,帮您拓宽课题思路:
AI项目摘要
AI项目思路
AI技术路线图
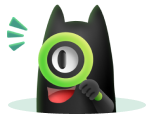
请为本次AI项目解读的内容对您的实用性打分
非常不实用
非常实用
1
2
3
4
5
6
7
8
9
10
您认为此功能如何分析更能满足您的需求,请填写您的反馈:
相似国自然基金
{{ item.name }}
- 批准号:{{ item.ratify_no }}
- 批准年份:{{ item.approval_year }}
- 资助金额:{{ item.support_num }}
- 项目类别:{{ item.project_type }}
相似海外基金
{{
item.name }}
{{ item.translate_name }}
- 批准号:{{ item.ratify_no }}
- 财政年份:{{ item.approval_year }}
- 资助金额:{{ item.support_num }}
- 项目类别:{{ item.project_type }}