面向中医数据库的方剂配伍规律智能挖掘模型研究
项目介绍
AI项目解读
基本信息
- 批准号:61802035
- 项目类别:青年科学基金项目
- 资助金额:24.0万
- 负责人:
- 依托单位:
- 学科分类:F0202.系统软件、数据库与工业软件
- 结题年份:2021
- 批准年份:2018
- 项目状态:已结题
- 起止时间:2019-01-01 至2021-12-31
- 项目参与者:乔少杰; 熊熙; 王亚强; 张永清; 李斌勇; 刘定祥; 丁鹏; 李艾鲜; 张笑天;
- 关键词:
项目摘要
By taking into full consideration the characteristics of multidimensional TCM (traditional Chinese medicine) data, this project focuses on the research of mining the compatibility of Chinese prescriptions. We adopt large-scale data preprocessing, complex networks, and association rule mining approaches to analyze TCM databases. In order to design a general schema, we model the factors affecting the compatibility of herbs, disclose the relationship and compound rules of herbs, explore the methods and strategies of discovering the compatibility of compound recipes by using intelligent mining techniques, and propose new concepts, specific theories and models, which can help set up the methodology on establishing the evaluation system for selecting compound recipes. The difficulties and essential problems in this project contain: (1) proposing multidimensional TCM data organization model, preprocessing TCM data from the aspects including data normalization, missing data filling, and noise processing; (2) constructing complex network model of prescriptions, designing core herb group identification algorithm based on modularity, and partitioning overlapping herbs by the membership function; (3) proposing a negative frequent pattern tree, measuring the association of herbs by mutual information, proposing an association rule mining algorithm based on the positive and negative support, handling the problem of over-frequent by using the maximum support, and improving the efficiency of mining frequent items by designing a support count matrix. This project can greatly improve the effectiveness and efficiency of knowledge discovery in large-scale TCM databases by using intelligence mining techniques.
本项目抓住中药多维数据特征,以方剂配伍规律挖掘问题为背景,将中医数据库作为研究对象,以大规模数据预处理、复杂网络、关联规则挖掘为主要研究手段,从模型通用性入手,对影响药物配伍关系的因素建模,揭示药物关联与组合的规律性,探索智能挖掘技术在方剂配伍规律发现中的方法意义和应对策略,提出药物配伍规律分析新概念、特色理论和模型,为中药复方筛选评价体系的建立进行理论和技术上的准备。研究难点和关键问题包括:(1)提出多维中药数据组织模型,从药物规范化、缺失数据填充、噪声处理等方面对中药数据预处理;(2)构建复杂药物网络模型,设计基于模块度的核心药物群识别算法,利用隶属度函数划分重叠药物;(3)提出负项频繁模式树,采用互信息度量药物关联性,提出基于正负双支持度的关联规则挖掘算法,利用最大支持度解决过频繁问题,设计支持度计数矩阵提高频繁项挖掘效率。利用智能挖掘技术切实提高大规模中药数据挖掘的有效性和效率。
结项摘要
本项目充分考虑中药多维数据特征,以方剂配伍规律挖掘问题为背景,将中医数据库作为研究对象,以大规模数据预处理、复杂网络、关联规则挖掘为主要研究手段,从模型通用性入手,对影响药物配伍关系的因素建模,揭示药物关联与组合的规律性,探索智能挖掘技术在方剂配伍规律发现中的方法意义和应对策略,提出药物配伍规律分析新概念、特色理论和模型,为中药复方筛选评价体系的建立进行理论和技术上的准备。主要研究内容及重要成果包括:(1)提出多维中药数据组织模型对多维中药数据概念分层,从药物规范化、缺失数据填充、噪声处理等方面对中药数据预处理;(2)提出了一种基于改进人工蜂群算法的参数优化方法,并将其用于药物聚类。该方法以轮廓系数指标作为蜂群算法的优化目标函数,使用改进的人工蜂群算法对DP算法的截断距离、局部密度值和距离δ进行寻优处理,找到最优的聚类中心。实验结果表明:相比于k-means,k-medoids,DBSCAN等传统聚类算法,所提方法较传统聚类算法能够有效地提高聚类质量,在轮廓系数、聚类纯度、聚类准确率、聚类召回率、F-Mesure等5个评价指标均有较大提高,在聚类熵评价指标上明显降低。所提算法对药物进行多维矢量聚类,能够较好地将药性相似的药物聚为一类,为进一步的中医药处方配伍规律挖掘给出了客观依据。(3)提出负项频繁模式树,采用互信息度量药物关联性,提出基于正负双支持度的关联规则挖掘算法,利用最大支持度解决过频繁问题,设计支持度计数矩阵提高频繁项挖掘效率。基于上述技术设计并开发了一个中医药学数据挖掘系统,已经应用于中医院等相关单位,辅助中医师临床诊断和开具方剂。
项目成果
期刊论文数量(13)
专著数量(0)
科研奖励数量(1)
会议论文数量(0)
专利数量(2)
移动社交网络快速社区并行发现算法
- DOI:--
- 发表时间:2020
- 期刊:重庆理工大学学报(自然科学)
- 影响因子:--
- 作者:韩楠;乔少杰;元昌安;黄萍;魏军林;彭京;周凯
- 通讯作者:周凯
Identification of DNA-protein binding sites by bootstrap multiple convolutional neural networks on sequence information
通过序列信息引导多个卷积神经网络识别 DNA-蛋白质结合位点
- DOI:10.1016/j.engappai.2019.01.003
- 发表时间:2019
- 期刊:Engineering Applications of Artificial Intelligence
- 影响因子:8
- 作者:Zhang Yongqing;Qiao Shaojie;Ji Shengjie;Han Nan;Liu Dingxiang;Zhou Jiliu
- 通讯作者:Zhou Jiliu
NetSRE: Link predictability measuring and regulating
NetSRE:链路可预测性测量和调节
- DOI:10.1016/j.knosys.2020.105800
- 发表时间:2020
- 期刊:Knowledge-Based Systems
- 影响因子:8.8
- 作者:Xian Xingping;Wu Tao;Qiao Shaojie;Wang Xi-Zhao;Wang Wei;Liu Yanbing
- 通讯作者:Liu Yanbing
不平衡分类的数据采样方法综述
- DOI:--
- 发表时间:2019
- 期刊:重庆理工大学学报(自然科学)
- 影响因子:--
- 作者:刘定祥;乔少杰;张永清;韩楠;魏军林;张榕珂;黄萍
- 通讯作者:黄萍
SOTB: Semi-Supervised Oversampling Approach Based on Trigonal Barycenter Theory
SOTB:基于三角重心理论的半监督过采样方法
- DOI:10.1109/access.2020.2980157
- 发表时间:2020
- 期刊:IEEE Access
- 影响因子:3.9
- 作者:Liu Dingxiang;Qiao Shaojie;Han Nan;Wu Tao;Mao Rui;Zhang Yongqing;Yuan Chang-An;Xiao Yueqiang
- 通讯作者:Xiao Yueqiang
数据更新时间:{{ journalArticles.updateTime }}
{{
item.title }}
{{ item.translation_title }}
- DOI:{{ item.doi || "--"}}
- 发表时间:{{ item.publish_year || "--" }}
- 期刊:{{ item.journal_name }}
- 影响因子:{{ item.factor || "--"}}
- 作者:{{ item.authors }}
- 通讯作者:{{ item.author }}
数据更新时间:{{ journalArticles.updateTime }}
{{ item.title }}
- 作者:{{ item.authors }}
数据更新时间:{{ monograph.updateTime }}
{{ item.title }}
- 作者:{{ item.authors }}
数据更新时间:{{ sciAawards.updateTime }}
{{ item.title }}
- 作者:{{ item.authors }}
数据更新时间:{{ conferencePapers.updateTime }}
{{ item.title }}
- 作者:{{ item.authors }}
数据更新时间:{{ patent.updateTime }}
其他文献
复杂网络大数据中重叠社区检测算法
- DOI:10.13328/j.cnki.jos.005155
- 发表时间:2016
- 期刊:软件学报
- 影响因子:--
- 作者:乔少杰;韩楠;张凯峰;邹磊;王宏志;Louis Alberto GUTIERREZ
- 通讯作者:Louis Alberto GUTIERREZ
一种基于样本空间的类别不平衡数据采样方法
- DOI:--
- 发表时间:--
- 期刊:自动化学报
- 影响因子:--
- 作者:张永清;卢荣钊;乔少杰;韩楠;GUTIERREZ Louis Alberto;周激流
- 通讯作者:周激流
基于前缀投影技术的大规模轨迹预测模型
- DOI:10.13328/j.cnki.jos.005340
- 发表时间:2017
- 期刊:软件学报
- 影响因子:--
- 作者:乔少杰;韩楠;李天瑞;李荣华;李斌勇;王晓腾;Louis Alberto GUTIERREZ
- 通讯作者:Louis Alberto GUTIERREZ
span style=color:#000000;background-color:#EFF1EF;云计算环境下基于代表点增量层次密度聚类的微博事件检测及跟踪/span
- DOI:--
- 发表时间:2013
- 期刊:计算机应用
- 影响因子:--
- 作者:冯永;韩楠;贾东风
- 通讯作者:贾东风
面向LBSN的k-medoids聚类算法
- DOI:--
- 发表时间:2017
- 期刊:中国科学技术大学学报
- 影响因子:--
- 作者:罗维佳;乔少杰;韩楠;元昌安;闭应洲;舒红平
- 通讯作者:舒红平
其他文献
{{
item.title }}
{{ item.translation_title }}
- DOI:{{ item.doi || "--" }}
- 发表时间:{{ item.publish_year || "--"}}
- 期刊:{{ item.journal_name }}
- 影响因子:{{ item.factor || "--" }}
- 作者:{{ item.authors }}
- 通讯作者:{{ item.author }}
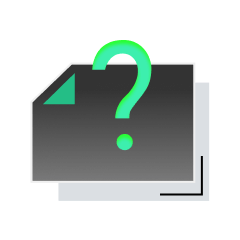
内容获取失败,请点击重试
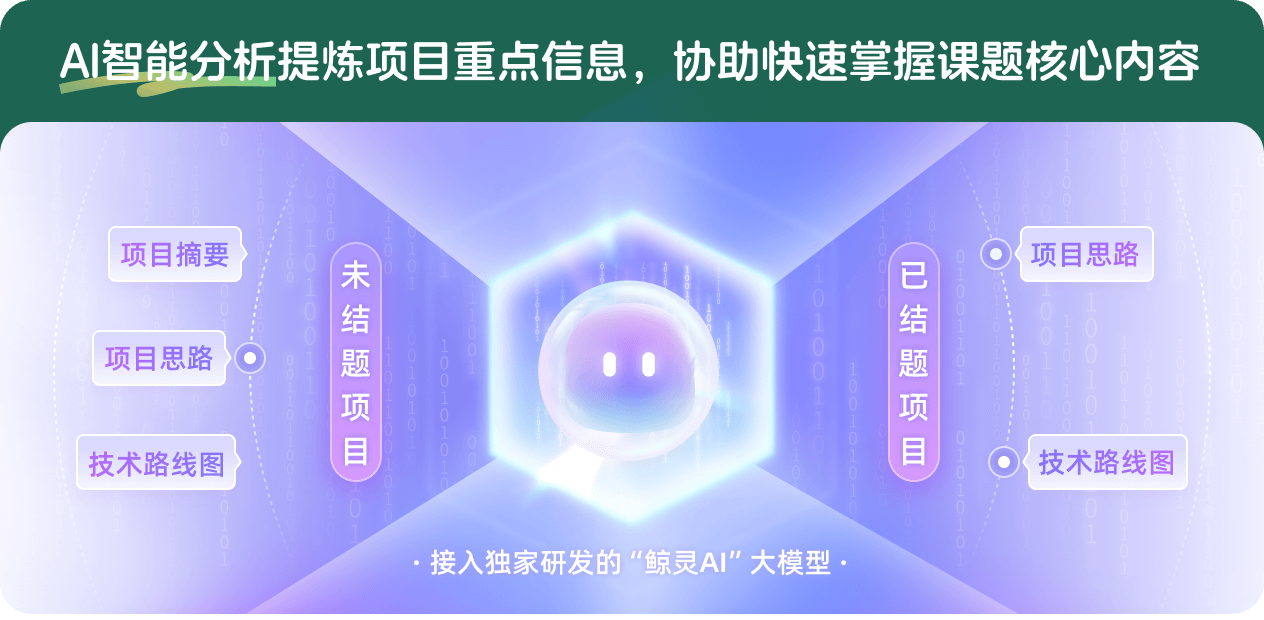
查看分析示例
此项目为已结题,我已根据课题信息分析并撰写以下内容,帮您拓宽课题思路:
AI项目摘要
AI项目思路
AI技术路线图
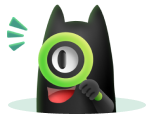
请为本次AI项目解读的内容对您的实用性打分
非常不实用
非常实用
1
2
3
4
5
6
7
8
9
10
您认为此功能如何分析更能满足您的需求,请填写您的反馈:
相似国自然基金
{{ item.name }}
- 批准号:{{ item.ratify_no }}
- 批准年份:{{ item.approval_year }}
- 资助金额:{{ item.support_num }}
- 项目类别:{{ item.project_type }}
相似海外基金
{{
item.name }}
{{ item.translate_name }}
- 批准号:{{ item.ratify_no }}
- 财政年份:{{ item.approval_year }}
- 资助金额:{{ item.support_num }}
- 项目类别:{{ item.project_type }}