多源数据判别特征学习的结构化自编码方法
项目介绍
AI项目解读
基本信息
- 批准号:61906046
- 项目类别:青年科学基金项目
- 资助金额:24.0万
- 负责人:
- 依托单位:
- 学科分类:F0605.模式识别与数据挖掘
- 结题年份:2022
- 批准年份:2019
- 项目状态:已结题
- 起止时间:2020-01-01 至2022-12-31
- 项目参与者:--
- 关键词:
项目摘要
Video image data analysis is a frontier research topic in the field of artificial intelligence and machine learning. Complex backgrounds and diverse variations make the tasks including feature extraction, fusion and matching very difficult. The characteristics of multi-source, heterogeneity and label deficiency further complicate the data analysis tasks. .This project studies structured auto-encoder methods for intelligent analysis of multi-source video image data. The main research contents and innovations include: 1) It performs structured regularizations on the latent variables in the framework of variational auto-encoder networks, and then integrates the structured auto-encoders with adversarial training strategy to improve the discriminability of network features; 2) It introduces non-Euclidean measures into the triplet loss function. It also prunes the positive and negative sample pairs reasonably to simultaneously ensure classification accuracy and reduce the computational complexity. 3) It tackles the double transfer tasks, including both identity and style attributes, and improve classification accuracy of the multi-modal image data..This project will provide new learning framework and optimization algorithms for multi-source image feature extraction and discriminant classification. It will improve the discrimination, robustness and efficiency for data analysis methods further. Preliminary results also provide a good research basis for this project.
视频图像数据分析是人工智能与机器学习领域的前沿研究课题。数据背景复杂与变化多样使得特征提取、融合与匹配都十分困难,多源异构与标签缺失的情景进一步加剧了数据分析任务的难度。.本项目研究多源视频图像数据智能分析的结构化自编码方法,主要研究内容和创新之处包括:1)在变分自编码框架下对隐变量做出结构化约束,将结构化自编码与对抗式训练结合起来,提高输出特征的判别性;2)在三元组损失函数中嵌入非欧度量并合理剪枝正负样本对,保证分类精度的同时有效减小计算复杂度与存储成本;3)针对数据呈现的多模态特点,解决风格与身份的双重迁移学习与高效率计算问题,有效提高测试样本的分类精度。.本项目将为多源视频图像数据的特征提取与判别分类问题提供新的学习框架和优化算法,进一步提高数据分析方法的判别性、稳健性和高效性。前期成果也为本项目的开展提供了良好的研究基础。
结项摘要
项目研究了多源数据的判别特征学习问题,基于度量学习原理、最优传输原理和深度网络架构建立了结构化自编码框架,提出了类别属性的隐变量学习方法、局部正则化的度量学习方法和多源数据分析的自适应深度网络模型,实现了跨域视觉语义关联性刻画与局部结构对齐。建立了最优传输与条件分布嵌入之间的理论联系,得到了基于条件分布对齐的跨域泛化误差分析结果,并提出高效率的深度学习方法,阐明了最优传输代价动态更新与判别信息迁移之间的内在关联。提出了基于生成式训练策略的多源域自适应表征学习算法簇,揭示了多源视觉数据信息融合与不变量描述的低维表征学习规律,增强了模型在跨域或异分布场景下的泛化能力。 .项目已在国际高水平期刊发表论文2篇。
项目成果
期刊论文数量(2)
专著数量(0)
科研奖励数量(0)
会议论文数量(0)
专利数量(0)
Unsupervised Domain Adaptation via Deep Conditional Adaptation Network
通过深度条件适应网络进行无监督域适应
- DOI:10.1016/j.patcog.2022.109088
- 发表时间:2022-10
- 期刊:Pattern Recognition
- 影响因子:8
- 作者:Pengfei Ge;Chuan-Xian Ren;Xiao-Lin Xu;Hong Yan
- 通讯作者:Hong Yan
数据更新时间:{{ journalArticles.updateTime }}
{{
item.title }}
{{ item.translation_title }}
- DOI:{{ item.doi || "--"}}
- 发表时间:{{ item.publish_year || "--" }}
- 期刊:{{ item.journal_name }}
- 影响因子:{{ item.factor || "--"}}
- 作者:{{ item.authors }}
- 通讯作者:{{ item.author }}
数据更新时间:{{ journalArticles.updateTime }}
{{ item.title }}
- 作者:{{ item.authors }}
数据更新时间:{{ monograph.updateTime }}
{{ item.title }}
- 作者:{{ item.authors }}
数据更新时间:{{ sciAawards.updateTime }}
{{ item.title }}
- 作者:{{ item.authors }}
数据更新时间:{{ conferencePapers.updateTime }}
{{ item.title }}
- 作者:{{ item.authors }}
数据更新时间:{{ patent.updateTime }}
其他文献
谈“肺肠相关”对承气汤及其类方临床应用的指导
- DOI:--
- 发表时间:2016
- 期刊:环球中医药
- 影响因子:--
- 作者:徐晓琳;李鹏英;王雪茜;程发峰
- 通讯作者:程发峰
p53蛋白磷酸化及其功能
- DOI:10.13376/j.cbls/2018030
- 发表时间:2018
- 期刊:生命科学
- 影响因子:--
- 作者:令吉明;王建;徐晓琳;耿云峰;王伟龙;赵春玲;田春艳
- 通讯作者:田春艳
变时滞模糊BAM神经网络的全局指数稳定性
- DOI:--
- 发表时间:--
- 期刊:合肥师范学院学报
- 影响因子:--
- 作者:陈立平;徐晓琳;王良龙
- 通讯作者:王良龙
标准生长曲线法在华南沿海老红砂石英光释光测年中的适用性
- DOI:10.7605/gdlxb.2018.03.037
- 发表时间:2018
- 期刊:古地理学报
- 影响因子:--
- 作者:程延;李志忠;靳建辉;徐晓琳;李志星;刘小菊
- 通讯作者:刘小菊
第四纪以来福建海坛岛北部软质海蚀崖演化过程研究简
- DOI:--
- 发表时间:2016
- 期刊:亚热带资源与环境学报
- 影响因子:--
- 作者:赖海成;于晓莉;程延;徐晓琳
- 通讯作者:徐晓琳
其他文献
{{
item.title }}
{{ item.translation_title }}
- DOI:{{ item.doi || "--" }}
- 发表时间:{{ item.publish_year || "--"}}
- 期刊:{{ item.journal_name }}
- 影响因子:{{ item.factor || "--" }}
- 作者:{{ item.authors }}
- 通讯作者:{{ item.author }}
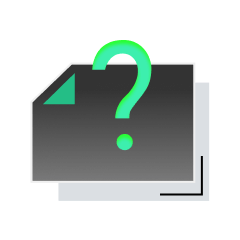
内容获取失败,请点击重试
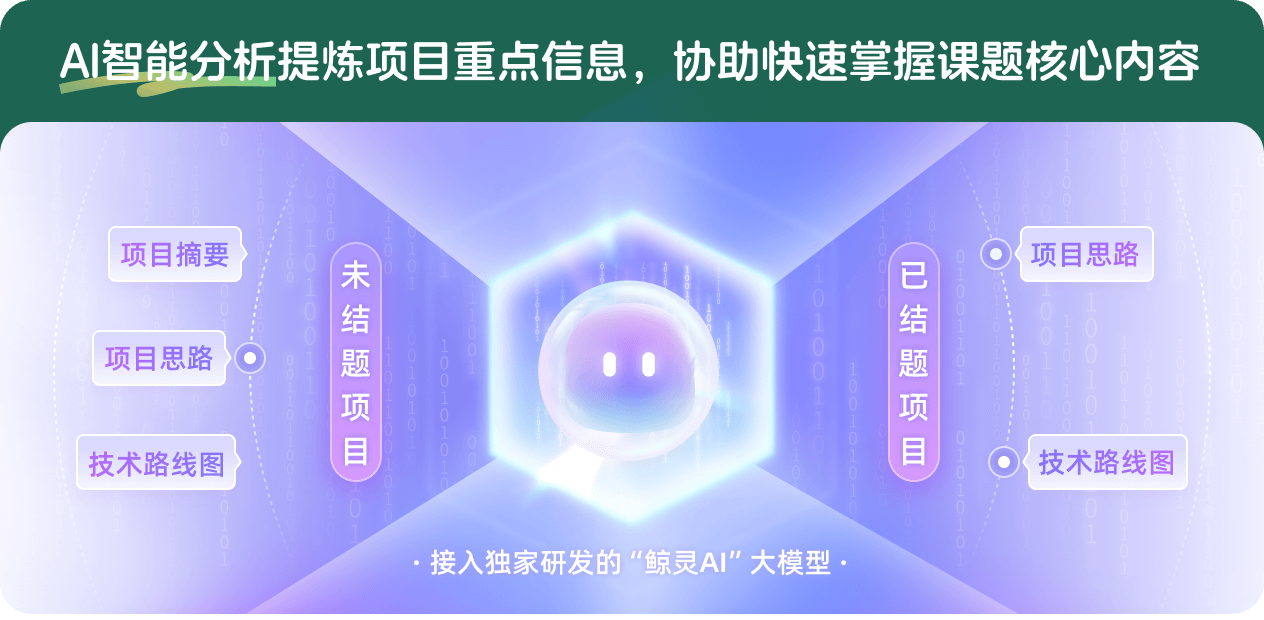
查看分析示例
此项目为已结题,我已根据课题信息分析并撰写以下内容,帮您拓宽课题思路:
AI项目摘要
AI项目思路
AI技术路线图
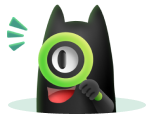
请为本次AI项目解读的内容对您的实用性打分
非常不实用
非常实用
1
2
3
4
5
6
7
8
9
10
您认为此功能如何分析更能满足您的需求,请填写您的反馈:
相似国自然基金
{{ item.name }}
- 批准号:{{ item.ratify_no }}
- 批准年份:{{ item.approval_year }}
- 资助金额:{{ item.support_num }}
- 项目类别:{{ item.project_type }}
相似海外基金
{{
item.name }}
{{ item.translate_name }}
- 批准号:{{ item.ratify_no }}
- 财政年份:{{ item.approval_year }}
- 资助金额:{{ item.support_num }}
- 项目类别:{{ item.project_type }}