基于深度迁移网络的多模高光谱图像分类方法研究
项目介绍
AI项目解读
基本信息
- 批准号:61801078
- 项目类别:青年科学基金项目
- 资助金额:23.0万
- 负责人:
- 依托单位:
- 学科分类:F0113.信息获取与处理
- 结题年份:2021
- 批准年份:2018
- 项目状态:已结题
- 起止时间:2019-01-01 至2021-12-31
- 项目参与者:郝应光; 耿杰; 付安岩; 周晓君; 程杨; 林宇轩;
- 关键词:
项目摘要
Hyperspectral image classification is crucial for remote sensing based earth observation tasks. Its performance directly impacts the application and development of remote sensing. However, due to the redundancy of hyperspectral images and the insufficient of training samples, current classification methods are damaged by the over-fitting and dimensionality disaster problems. Moreover, due to the feature drift and the task inconsistency of multimode images, existing methods cannot transfer to multimode data. To solve these problems, this proposal studies the above issues from information mining, utilization and transferring. Firstly, in order to mine effective information from redundant data using a small training set, this proposal proposes a dual-channel deep deconvolution network to learn and optimize the spectral and spatial information of the hyperspectral image. Secondly, in order to efficiently utilize the insufficient training samples, this proposal proposes a semisupervised classification method based on the metric learning to perform classification. Finally, in order to transfer the proposed method in multimode data, this proposal explores solutions based on deep transfer networks, and proposes a domain adaptive method based on parallel transfer networks and a zero-time learning method based on multi-level transfer networks to complete classification of multimodal images. This project will realize accurate, stable, and continuous multimode data classification, the achievements and results will enrich and promote the research of hyperspectral image analysis, and provide theory and technique support for the applications of remote sensing in many fields.
高光谱图像分类是遥感对地观测中的关键问题,其精度直接决定着遥感技术的应用与发展。但是,受数据冗余大且训练样本不足的影响,现有分类方法受到过拟合与维数灾难等问题的困扰;同时,受多模图像特征漂移与任务不一致的影响,现有方法难以实现跨数据迁移。针对此,本课题拟从信息的挖掘、利用、以及迁移三个角度展开研究。首先,针对如何在训练样本不足时从冗余的高光谱图像中挖掘有效信息的问题,提出双通道反卷积网络以学习并优化空谱特征;其次,针对如何高效利用有限训练样本的问题,提出基于度量学习的半监督分类方法以实现源图像分类;最后,针对如何实现分类方法的多模数据迁移问题,探索基于深度迁移网络的解决方案,提出基于并行迁移网络的领域自适应方法与基于分级迁移网络的零次学习方法以实现多模图像分类。本课题将实现精准、稳定、持续的多模数据分类,其研究成果将丰富和完善高光谱图像分析方法,为诸多领域的遥感应用提供理论与技术的支撑。
结项摘要
高光谱图像分类是遥感对地观测中的关键问题,其精度直接决定着遥感技术的应用与发展。但是,受数据冗余大且训练样本不足的影响,现有分类方法受到过拟合与维数灾难等问题的困扰;同时,受多模图像特征漂移与任务不一致的影响,现有方法难以实现跨数据迁移。针对此,以实现多模高光谱图像分类为目标,课题组提出了基于双阶段关系学习网络的小样本分类方法解决样本不足问题,基于任务引导学习网络的小样本高光谱图像分类方法解决样本数量不均衡问题,基于领域自适应的高光谱图像分类方法实现跨数据分类,基于生成对抗网络的高光谱分类方法实现无监督跨数据分类,基于参数预测网络的高光谱图像特征提取方法有效学习高光谱图像特征,知识引导型高光谱图像分类方法实现高效的高光谱分类。此外,将提出的方法在无线感知、SAR图像目标识别与分类上、以及变化监测上进行了交叉应用。成果以论文形式公开发表,累计论文17篇,其中,SCI论文13篇,IEEE汇刊11篇,申请国家发明专利及软著12项,已获批国家发明专利2项,软著5项,项目的成果远超预期成果。
项目成果
期刊论文数量(13)
专著数量(0)
科研奖励数量(1)
会议论文数量(4)
专利数量(2)
Cross-Scenario Device-Free Activity Recognition Based on Deep Adversarial Networks
基于深度对抗网络的跨场景无设备活动识别
- DOI:10.1109/tvt.2020.2977973
- 发表时间:2020-05-01
- 期刊:IEEE TRANSACTIONS ON VEHICULAR TECHNOLOGY
- 影响因子:6.8
- 作者:Wang, Jie;Zhao, Yunong;Wang, Hongyu
- 通讯作者:Wang, Hongyu
Cross-data set hyperspectral image classification based on deep domain adaptation
基于深域自适应的跨数据集高光谱图像分类
- DOI:10.1109/tgrs.2019.2931730
- 发表时间:2020
- 期刊:IEEE Transactions on Geoscience and Remote Sensing
- 影响因子:8.2
- 作者:Xiaorui Ma;Xuerong Mou;Jie Wang;Xiaokai Liu;Hongyu Wang;Baocai Yin
- 通讯作者:Baocai Yin
Multiscale and Dense Ship Detection in SAR Images Based on Key-Point Estimation and Attention Mechanism
基于关键点估计和注意力机制的SAR图像多尺度密集船舶检测
- DOI:10.1109/tgrs.2022.3141407
- 发表时间:2022-01-01
- 期刊:IEEE TRANSACTIONS ON GEOSCIENCE AND REMOTE SENSING
- 影响因子:8.2
- 作者:Ma, Xiaorui;Hou, Shilong;Wang, Hongyu
- 通讯作者:Wang, Hongyu
Hyperspectral Image Classification Based on Two-phase Relation Learning Network
基于两阶段关系学习网络的高光谱图像分类
- DOI:10.1109/tgrs.2019.2934218
- 发表时间:2019
- 期刊:IEEE Transactions on Geoscience and Remote Sensing
- 影响因子:8.2
- 作者:Xiaorui Ma;Sheng Ji;Jie Wang;Jie Geng;Hongyu Wang
- 通讯作者:Hongyu Wang
Robust AUV Visual Loop Closure Detection Based on Variational Auto-Encoder Network
基于变分自编码器网络的鲁棒AUV视觉闭环检测
- DOI:10.1109/tii.2022.3145860
- 发表时间:--
- 期刊:IEEE Transactions on Industrial Informatics
- 影响因子:12.3
- 作者:Yangyang Wang;Xiaorui Ma;Jie Wang;Shilong Hou;Ju Dai;Dongbing Gu;Hongyu Wamg
- 通讯作者:Hongyu Wamg
数据更新时间:{{ journalArticles.updateTime }}
{{
item.title }}
{{ item.translation_title }}
- DOI:{{ item.doi || "--"}}
- 发表时间:{{ item.publish_year || "--" }}
- 期刊:{{ item.journal_name }}
- 影响因子:{{ item.factor || "--"}}
- 作者:{{ item.authors }}
- 通讯作者:{{ item.author }}
数据更新时间:{{ journalArticles.updateTime }}
{{ item.title }}
- 作者:{{ item.authors }}
数据更新时间:{{ monograph.updateTime }}
{{ item.title }}
- 作者:{{ item.authors }}
数据更新时间:{{ sciAawards.updateTime }}
{{ item.title }}
- 作者:{{ item.authors }}
数据更新时间:{{ conferencePapers.updateTime }}
{{ item.title }}
- 作者:{{ item.authors }}
数据更新时间:{{ patent.updateTime }}
其他文献
其他文献
{{
item.title }}
{{ item.translation_title }}
- DOI:{{ item.doi || "--" }}
- 发表时间:{{ item.publish_year || "--"}}
- 期刊:{{ item.journal_name }}
- 影响因子:{{ item.factor || "--" }}
- 作者:{{ item.authors }}
- 通讯作者:{{ item.author }}
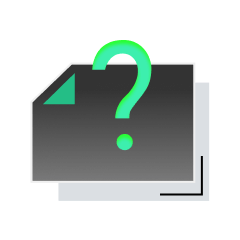
内容获取失败,请点击重试
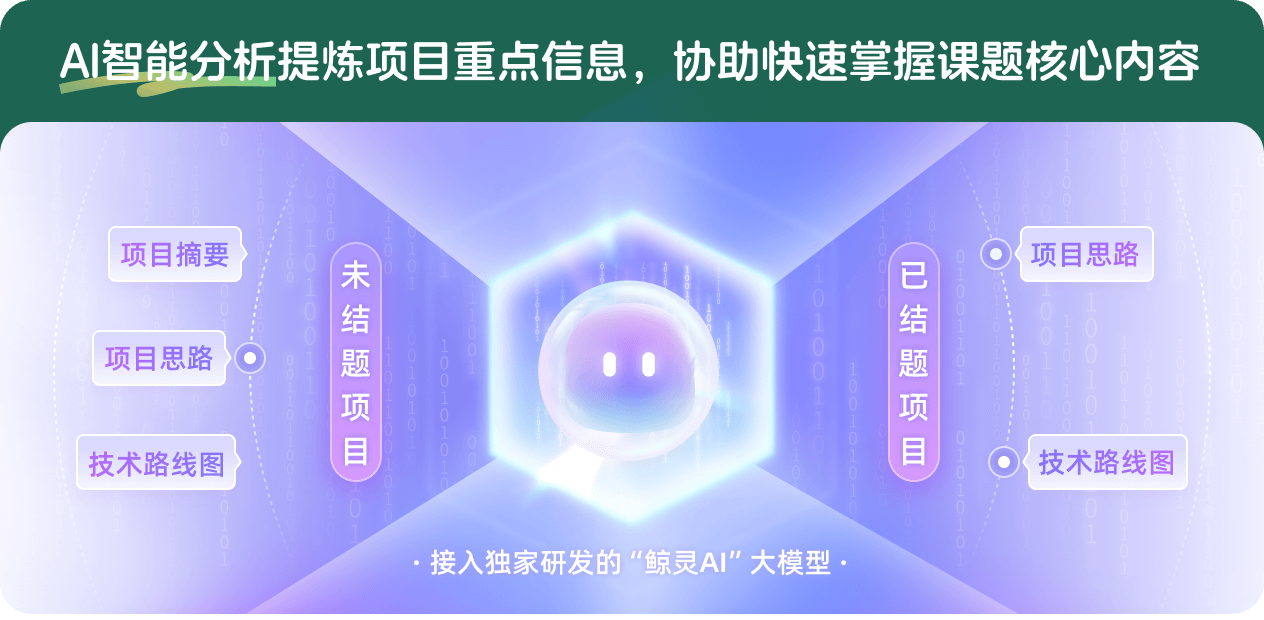
查看分析示例
此项目为已结题,我已根据课题信息分析并撰写以下内容,帮您拓宽课题思路:
AI项目摘要
AI项目思路
AI技术路线图
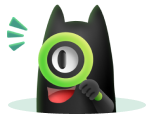
请为本次AI项目解读的内容对您的实用性打分
非常不实用
非常实用
1
2
3
4
5
6
7
8
9
10
您认为此功能如何分析更能满足您的需求,请填写您的反馈:
马晓瑞的其他基金
基于信息挖掘与共享的跨数据高光谱图像分类方法研究
- 批准号:
- 批准年份:2022
- 资助金额:54 万元
- 项目类别:面上项目
基于信息挖掘与共享的跨数据高光谱图像分类方法研究
- 批准号:62271102
- 批准年份:2022
- 资助金额:54.00 万元
- 项目类别:面上项目
相似国自然基金
{{ item.name }}
- 批准号:{{ item.ratify_no }}
- 批准年份:{{ item.approval_year }}
- 资助金额:{{ item.support_num }}
- 项目类别:{{ item.project_type }}
相似海外基金
{{
item.name }}
{{ item.translate_name }}
- 批准号:{{ item.ratify_no }}
- 财政年份:{{ item.approval_year }}
- 资助金额:{{ item.support_num }}
- 项目类别:{{ item.project_type }}