基于证据链的可推理机器阅读理解关键技术研究
项目介绍
AI项目解读
基本信息
- 批准号:61906217
- 项目类别:青年科学基金项目
- 资助金额:24.0万
- 负责人:
- 依托单位:
- 学科分类:F0606.自然语言处理
- 结题年份:2022
- 批准年份:2019
- 项目状态:已结题
- 起止时间:2020-01-01 至2022-12-31
- 项目参与者:--
- 关键词:
项目摘要
Machine comprehension is one of the hot research topics in natural language processing. This task focuses on the research for machine's understanding on the semantics in natural language. It requires the machine to be able to generate the corresponding answers according to the input questions with regards to a given document. Traditional methods often choose the answer by computing the similarity between question-answer pair and the document. Due to the lack of the fine-grained inference process, these methods have minimal capability to link related supports distributed across multiple sentences for deducing the answers. We observe that such linked relations are mainly embodied in the text semantic structure, includes sequential, syntactic, logical and rhetoric structures, etc. Thus, we in this project intend to study the methods for constructing the evidence chain by leveraging the text semantic structure, and using the evidence chain to improve the machine's deeply reasoning ability on the text meanings. The main researches on this project include: (1) we investigate a model to effectively represent the text semantic structure; (2) we employ an approach to interpret the question details, so as to fully grasp the intentions of the questioners; (3) we propose an end-to-end inferential network to construct evidence chain by simultaneously capturing the text semantic structure and question details, and explore the terminated mechanism as well as the accelerated training technique on the network. Finally, we intend to achieve breakthroughs on this research topic for publishing papers with cutting edge results.
机器阅读理解是自然语言处理领域的研究热点之一。该任务侧重于机器对于自然语言语义理解的研究,它要求机器能够根据输入的问题在给定的文档中解答出对应的答案。传统的方法通常是计算问题答案对与文档之间的相似度来进行答案选取。由于缺少细粒度的推理过程,这些方法未能有效地关联分散在不同句子之间的相关内容来推理出答案。我们发现这些关联信息主要体现在文本的语义结构中,包括次序、语法、逻辑和修辞等结构。本项目拟研究利用文本语义结构建立证据链的方法,并基于证据链来提高机器对于文本深层次的语义推理能力。主要研究内容包括:(1)拟研究能有效地表示文本语义结构的模型;(2)拟构建模型解析问题细节来充分地把握提问者本意;(3)拟提出可推理的神经网络通过结合语义结构和问题细节来建立证据链,并研究对应的推理终止机制和加速训练方法。最后,拟在本课题上取得突破以发表具有国际领先水平的研究成果。
结项摘要
本项目主要研究面向语言智能的机器阅读理解和推理技术。该课题在信息检索、智慧教育等应用中具有广泛的商业价值。由于语言存在歧义性、语义模糊性和上下文依赖性,传统基于确定性原理设计的机器很难直接对抽象的非确定性语义建模,存在“表征浅层、推理低阶和资源稀缺”等挑战。项目围绕着这些难点展开了系统的研究,在本项目资助下,研发出一批关键技术,包括提出了基于语义结构的表征方法,解决了知识发现和表示难题;提出了基于协同复合的关联推理技术,揭示了知识归纳和关联推理的规律,大幅提升了对话问答的质量;还提出了基于知识引导的推理生成方法,缓解了稀缺标注资源的过度依赖难题;这些工作于 IEEE Transactions 和中国计算机学会推荐的 A 类等重要期刊和国际会议上发表论文11篇,包括 IEEE TKDE 等国际知名期刊及 ACL、WWW、ICDE、AAAI、IJCAI等国际著名会议;曾多次获得广东省计算机学会优秀论文一等奖,还获得2021年广东省计算机学会科学奖;超额完成了原计划书中预期目标。在知识产权方面,项目组申请了 8件发明专利,其中有 2 件已经授权。这推动成果的产业化应用,譬如本项目的技术可以用于智慧教育实现面向考试的机器自动命题。此外,在人才培养方面,培养6 名在读研究生。这些成果还在中国计算机大会,全国知识图谱与语义计算大会等国内影响力很大的学术会议上做专题报告,具有相当的影响力。这些工作有力地推动了语言智能的发展。
项目成果
期刊论文数量(2)
专著数量(0)
科研奖励数量(3)
会议论文数量(9)
专利数量(8)
Multi-hop Reasoning Question Generation and Its Application
多跳推理题生成及其应用
- DOI:10.1140/epjp/s13360-021-01478-w
- 发表时间:2021
- 期刊:IEEE Transactions on Knowledge and Data Engineering (TKDE)
- 影响因子:--
- 作者:Jianxing Yu;Qinliang Su;Xiaojun Quan;Jian Yin
- 通讯作者:Jian Yin
Adaptive Cross-lingual Question Generation with Minimal Resources
用最少的资源生成自适应跨语言问题
- DOI:10.1093/comjnl/bxab106
- 发表时间:2021
- 期刊:The Computer Journal
- 影响因子:--
- 作者:Jianxing Yu;Shiqi Wang;Jian Yin
- 通讯作者:Jian Yin
数据更新时间:{{ journalArticles.updateTime }}
{{
item.title }}
{{ item.translation_title }}
- DOI:{{ item.doi || "--"}}
- 发表时间:{{ item.publish_year || "--" }}
- 期刊:{{ item.journal_name }}
- 影响因子:{{ item.factor || "--"}}
- 作者:{{ item.authors }}
- 通讯作者:{{ item.author }}
数据更新时间:{{ journalArticles.updateTime }}
{{ item.title }}
- 作者:{{ item.authors }}
数据更新时间:{{ monograph.updateTime }}
{{ item.title }}
- 作者:{{ item.authors }}
数据更新时间:{{ sciAawards.updateTime }}
{{ item.title }}
- 作者:{{ item.authors }}
数据更新时间:{{ conferencePapers.updateTime }}
{{ item.title }}
- 作者:{{ item.authors }}
数据更新时间:{{ patent.updateTime }}
其他文献
新型冠状病毒肺炎不同年龄组患者临床特征分析
- DOI:--
- 发表时间:2021
- 期刊:疾病监测
- 影响因子:--
- 作者:耿梦杰;任翔;余建兴;蒋荣猛;李中杰;冯子健;王丽萍;何广学
- 通讯作者:何广学
大数据治理的全景式框架
- DOI:--
- 发表时间:2020
- 期刊:大数据
- 影响因子:--
- 作者:印鉴;朱怀杰;余建兴;邱爽
- 通讯作者:邱爽
其他文献
{{
item.title }}
{{ item.translation_title }}
- DOI:{{ item.doi || "--" }}
- 发表时间:{{ item.publish_year || "--"}}
- 期刊:{{ item.journal_name }}
- 影响因子:{{ item.factor || "--" }}
- 作者:{{ item.authors }}
- 通讯作者:{{ item.author }}
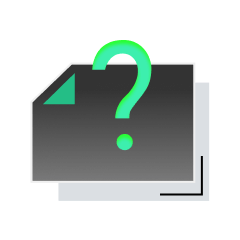
内容获取失败,请点击重试
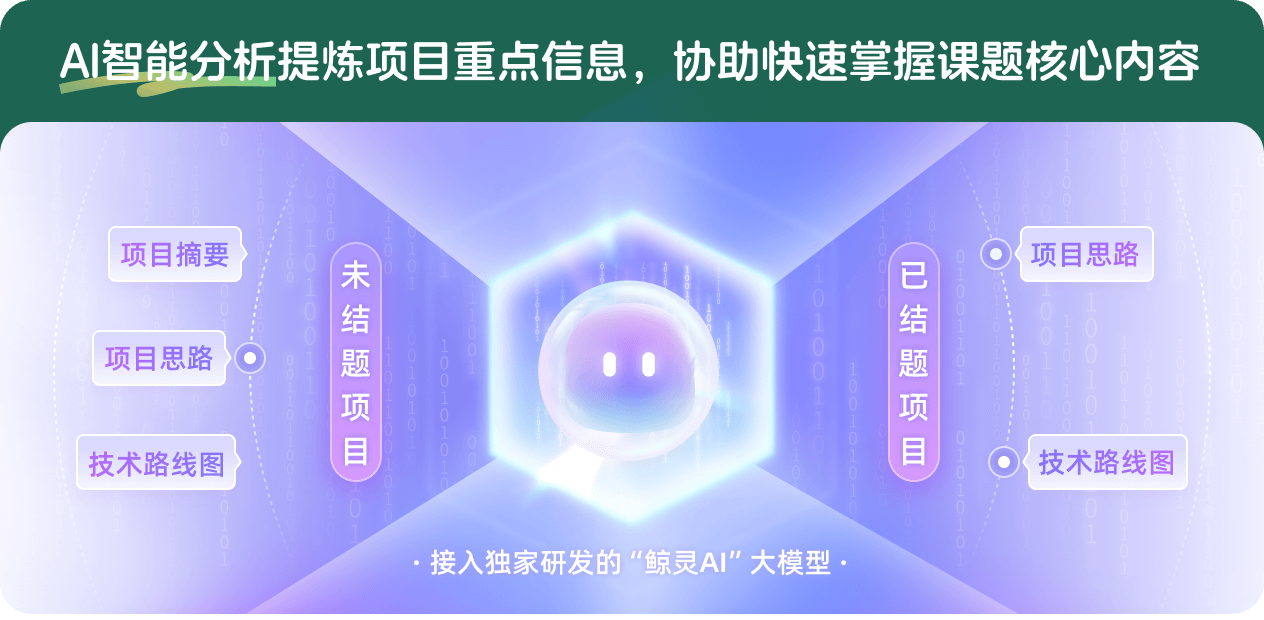
查看分析示例
此项目为已结题,我已根据课题信息分析并撰写以下内容,帮您拓宽课题思路:
AI项目摘要
AI项目思路
AI技术路线图
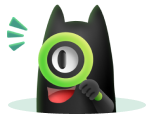
请为本次AI项目解读的内容对您的实用性打分
非常不实用
非常实用
1
2
3
4
5
6
7
8
9
10
您认为此功能如何分析更能满足您的需求,请填写您的反馈:
余建兴的其他基金
基于跨模态推理的机器阅读理解技术研究
- 批准号:
- 批准年份:2022
- 资助金额:53 万元
- 项目类别:面上项目
相似国自然基金
{{ item.name }}
- 批准号:{{ item.ratify_no }}
- 批准年份:{{ item.approval_year }}
- 资助金额:{{ item.support_num }}
- 项目类别:{{ item.project_type }}
相似海外基金
{{
item.name }}
{{ item.translate_name }}
- 批准号:{{ item.ratify_no }}
- 财政年份:{{ item.approval_year }}
- 资助金额:{{ item.support_num }}
- 项目类别:{{ item.project_type }}