基于变量选择与训练群体优化的植物基因组选择方法研究
项目介绍
AI项目解读
基本信息
- 批准号:31701164
- 项目类别:青年科学基金项目
- 资助金额:25.0万
- 负责人:
- 依托单位:
- 学科分类:C0608.生物数据资源与分析方法
- 结题年份:2020
- 批准年份:2017
- 项目状态:已结题
- 起止时间:2018-01-01 至2020-12-31
- 项目参与者:李兰芝; 陈渊; 曾莹; 张雪丽; 蔡训辉;
- 关键词:
项目摘要
Explore and develop accurate and efficient novel method for breeding value estimation of genomic selection (GS) is one of the key content in breeding and genetic improvement of animals and plants. Furthermore, variable selection and optimization of training population are important means to improve the accuracy of GS. This project incorporates several concepts including BMSF a nonlinear screening method of high-dimensional features, ChiMIC served as a measure of nonlinear correlation, strategy of redundancy apportion, and haplotype block, to construct a variable selection method with characteristics that can discover nonlinear correlation between quantitative traits and single nucleotide polymorphisms (SNP) markers, eliminate redundancy among SNPs effectively, consider joint effect for multiple markers with minor effect acting on traits, and depict linkage disequilibrium. Based on subset of haploblocks, we construct a method for optimization of training population with feature of personalized prediction by using population structure analysis and semivariance in geostatistics which can reflect spatial variability and structure. Couple the two novel methods with support vector regression and then apply to genomic selection for quantitative traits of model plant Arabidopsis, important grain crop wheat, rice, and maize. Finally, comprehensive comparison will be conducted between our methods and reference models. This project implements the optimization from double directions for rows and columns of GS datasets. The accuracy of genomic selection expects to be improved significantly. The breeding progress and germplasm innovation would be accelerated under the guidance of methods proposed in this project.
探索和发展准确、高效的全基因组选择(GS)育种值估计新方法是动植物育种和遗传改良的关键内容之一,而变量选择与训练群体优化是提高GS准确性的重要手段。本项目结合高维特征非线性筛选方法BMSF、非线性关联测度指标ChiMIC、冗余分摊策略以及单倍型域理念,构建可检测各单核苷酸多态性(SNP)标记与数量性状间的非线性关联、能有效剔除SNP之间的冗余信息、考虑多个微效标记对性状的联合效应及标记间连锁不平衡程度的变量选择方法。基于单倍型域子集,利用群体结构分析与地统计学中能反映空间变异和结构的半变异函数构建具有个性化预测功能的训练群体优化方法。结合新方法与支持向量回归模型并用于拟南芥、小麦、水稻和玉米的数量性状全基因组选择,最后与多种模型综合参比。该项目从非线性视角结合数量性状的遗传学背景以实现GS数据行、列两个方向的优化,有望显著提高基因组选择的准确性,为加速育种进展与种质创新提供方法学指导。
结项摘要
农业大数据时代,如何从组学层面快速、深度挖掘影响植物表型的复杂遗传位点、基因以及调控网络,是植物科学、作物育种领域关注的焦点。全基因组选择遗传育种需要在训练群体上建立植物表型与基因组尺度的遗传变异(通常使用单核苷酸多态性,即SNP)位点之间的统计模型,并对验证群体的个体表型实施预测,选出理想个体以指导育种。全基因组范围的SNP位点中必然存在大量冗余、与表型无关位点,这要求设计统计模型时必须考虑特征选择。本项目自实施以来,在高维特征选择算法创新方面,已开发2种新算法用于影响植物生长环境的醇/芳香类有机化合物的定量构效关系研究。新算法在4个数据集上均选出了比参比方法更精炼的、具有明确生物学意义的分子描述符,基于保留描述符的回归模型优于其他参比模型。新算法结合单倍型策略与训练群体优化方法在水稻、拟南芥的基因组预测上已获得初步结果。本项目发展的算法可有效挖掘因变量与高维自变量之间的统计规律,在加速计算机辅助药物设计、提高基因组预测精度进而缩短育种周期等数量性状建模方面,有望获得广泛应用。
项目成果
期刊论文数量(2)
专著数量(0)
科研奖励数量(0)
会议论文数量(0)
专利数量(0)
Chi-MIC-share: a new feature selection algorithm for quantitative structure-activity relationship models.
Chi-MIC-share:定量构效关系模型的新特征选择算法
- DOI:10.1039/d0ra00061b
- 发表时间:2020-05-26
- 期刊:RSC advances
- 影响因子:3.9
- 作者:
- 通讯作者:
Maximal Information Coefficient and Support Vector Regression Based Nonlinear Feature Selection and QSAR Modeling on Toxicity of Alcohol Compounds to Tadpoles of Rana temporaria
基于最大信息系数和支持向量回归的非线性特征选择和QSAR建模醇类化合物对林蛙蝌蚪的毒性
- DOI:10.21577/0103-5053.20180176
- 发表时间:2018
- 期刊:Journal of the Brazilian Chemical Society
- 影响因子:1.4
- 作者:Wang Lifeng;Xing Pengwei;Wang Cong;Zhou Xiaomao;Dai Zhijun;Bai Lianyang
- 通讯作者:Bai Lianyang
数据更新时间:{{ journalArticles.updateTime }}
{{
item.title }}
{{ item.translation_title }}
- DOI:{{ item.doi || "--"}}
- 发表时间:{{ item.publish_year || "--" }}
- 期刊:{{ item.journal_name }}
- 影响因子:{{ item.factor || "--"}}
- 作者:{{ item.authors }}
- 通讯作者:{{ item.author }}
数据更新时间:{{ journalArticles.updateTime }}
{{ item.title }}
- 作者:{{ item.authors }}
数据更新时间:{{ monograph.updateTime }}
{{ item.title }}
- 作者:{{ item.authors }}
数据更新时间:{{ sciAawards.updateTime }}
{{ item.title }}
- 作者:{{ item.authors }}
数据更新时间:{{ conferencePapers.updateTime }}
{{ item.title }}
- 作者:{{ item.authors }}
数据更新时间:{{ patent.updateTime }}
其他文献
基于序列特征筛选与支持向量回归预测蛋白质折叠速率
- DOI:--
- 发表时间:2014
- 期刊:Acta Physico - Chimica Sinica
- 影响因子:--
- 作者:代志军;陈渊;王志明;袁哲明
- 通讯作者:袁哲明
乳腺癌组织SATB1基因表达与临床病理特征的关系
- DOI:--
- 发表时间:2015
- 期刊:中华肿瘤防治杂志
- 影响因子:--
- 作者:靳耀锋;薛兴欢;代志军;姜建涛
- 通讯作者:姜建涛
miR-125a和miR-206在乳腺癌中的表达及临床意义
- DOI:--
- 发表时间:2014
- 期刊:贵阳医学院学报
- 影响因子:--
- 作者:赵阳;代志军;王西京;马清涌
- 通讯作者:马清涌
磷酸化 Caspase-8 在非小细胞肺癌中的表达及其临床意义
- DOI:--
- 发表时间:2014
- 期刊:南方医科大学学报
- 影响因子:--
- 作者:代志军;张寅斌;王西京;赵阳
- 通讯作者:赵阳
MR 非增强序列的联合应用对乳腺癌的诊断价值的探讨
- DOI:--
- 发表时间:--
- 期刊:实用放射学杂志
- 影响因子:--
- 作者:赵暹;陈欣;刘瑜;郭佑民;代志军;康华峰;刘燕
- 通讯作者:刘燕
其他文献
{{
item.title }}
{{ item.translation_title }}
- DOI:{{ item.doi || "--" }}
- 发表时间:{{ item.publish_year || "--"}}
- 期刊:{{ item.journal_name }}
- 影响因子:{{ item.factor || "--" }}
- 作者:{{ item.authors }}
- 通讯作者:{{ item.author }}
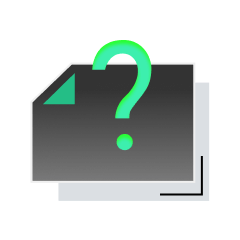
内容获取失败,请点击重试
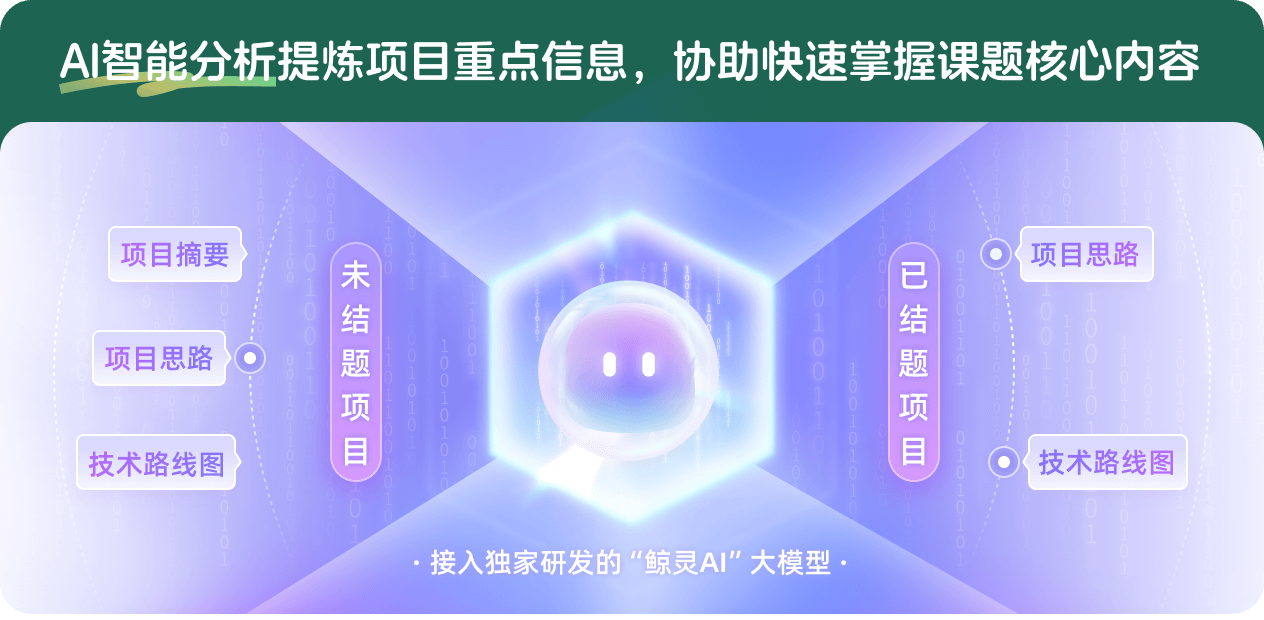
查看分析示例
此项目为已结题,我已根据课题信息分析并撰写以下内容,帮您拓宽课题思路:
AI项目摘要
AI项目思路
AI技术路线图
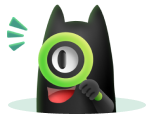
请为本次AI项目解读的内容对您的实用性打分
非常不实用
非常实用
1
2
3
4
5
6
7
8
9
10
您认为此功能如何分析更能满足您的需求,请填写您的反馈:
相似国自然基金
{{ item.name }}
- 批准号:{{ item.ratify_no }}
- 批准年份:{{ item.approval_year }}
- 资助金额:{{ item.support_num }}
- 项目类别:{{ item.project_type }}
相似海外基金
{{
item.name }}
{{ item.translate_name }}
- 批准号:{{ item.ratify_no }}
- 财政年份:{{ item.approval_year }}
- 资助金额:{{ item.support_num }}
- 项目类别:{{ item.project_type }}