脑细胞外间隙冷冻电镜高分辨率三维结构测定及建模定量分析
项目介绍
AI项目解读
基本信息
- 批准号:61901008
- 项目类别:青年科学基金项目
- 资助金额:25.5万
- 负责人:
- 依托单位:
- 学科分类:F0125.医学信息检测与处理
- 结题年份:2022
- 批准年份:2019
- 项目状态:已结题
- 起止时间:2020-01-01 至2022-12-31
- 项目参与者:--
- 关键词:
项目摘要
In recent years, the field of brain neuroscience has continuously reported the important role of extracellular space (ECS) in the physiological and pathological processes such as sleep, memory, AD and PD. ECS is a direct microenvironment from the existence of brain cells and neural networks. A variety of in vivo detection means, using diffusion measurement methods, have indirectly inferred ECS is of a nanoscale ultrastructure. Although it has long been recognized that only electron microscopy (EM) can reveal ECS directly, the limitations of conventional electron microscopy technology make the results of EM observation inconsistent or even contradictory with in vivo detection. This project will comprehensively utilize the breakthrough technologies in the field of electron microscopy in recent years, namely, cryo electron microscopy (cryoEM) sample preparation technique, Correlative light and electron microscopy (CLEM), cryo focused ion beam technology (cryoFIB) and cryo electron tomography technology (cryoET). By solving the key technical problems such as, the low resolution problem, high computation complexity problem and long time-consuming problem caused by the three-dimensional reconstruction algorithms and imaging processing approaches commonly used in cryoEM field today, the high-resolution three-dimensional ultra-structures of ECS in different brain regions will precisely be obtained, which can be good references to test and verify the accuracy of ECS structures with the in vivo methods. Based on the comparison results onabout characteristic parameters from in vivo detection with those extracted from ECS cryoEM structures, the modeling equations of in vivo measurement data can be optimized to exactly reveal the ways and rules of interstitial fluid drainage in ECS, and thus to promote its application in frontier fields such as pharmacy, tumor, neurodegenerative diseases and brain-like processes, et al.
近年脑神经科学领域连续报道脑细胞外间隙(ECS)在睡眠、记忆、AD、PD等生理病理过程中的重要作用。现有活体检测多采用扩散测量方法,间接推测出ECS纳米结构空间。目前冷冻电镜领域的突破性技术,使得直接观测揭示ECS结构成为可能,而传统电镜检测手段需要先行脱水处理等,导致ECS超微结构空间一直未被业界发现和重视。本项目将综合冷冻样品制备技术,冷冻光电关联技术,冷冻聚焦离子束技术和冷冻透射电子断层成像技术等冷冻电镜领域前沿新技术,通过克服这些技术在对脑ECS成像过程中的瓶颈得到ECS高分辨率三维空间结构,获得鼠脑不同脑区ECS空间分布,揭示ECS内间质液引流的途径与规律,关联不同成像模态跨尺度图像分析,比对活体测量方法所得ECS结构及参数,验证活体测量方法的准确性,为优化活体测量方法提供参考依据。从而为再生医学、免疫、药学、肿瘤、神经退行疾病、类脑等前沿领域的ECS研究提供标准化的测量工具。
结项摘要
脑细胞外间隙(Extracellular space,ECS)内分子转运是保持睡眠、记忆等脑活动的功能基础,但其精细结构的直接成像方法仍未建立。本项目利用目前冷冻电镜领域的突破性技术,优化改进对组织样品尤其是脑组织样品的高压冷冻及冷冻替代的样品制备方法,使用聚焦离子束扫描电镜(FIB-SEM)和冷冻电子断层透射电镜(cryoET)对优选的组织样品进行三维数据收集,在对三维电镜堆栈数据进行去噪、重构、分割、建模分析过程中,初步探索出了一套适用于解析ECS超微结构并揭示ECS内间质液引流规律的方法技术。 .重要结果具体为:1)建立了ECS三维电镜结构定量测量的完整流程;2)实现了ECS三维电镜结构半自动手工标注提取和深度学习智能分割;3)实现了ECS电镜三维断层数据重构渲染及定量分析;4)基于ECS三维超微结构进行了流体模拟和空间网络关系模拟;5)基于心肌组织探索了电镜组织样品制备新方法并实现cryoET数据收集和分析建模;6)实现了深度学习人工智能算法多用途验证及移植使用,具体为前列腺病理图片分类、手功能评估及新生儿运动发育评估。.获取的关键数据有:1)正常SD大鼠脑组织海马区、尾状核和丘脑的二维透射电镜(TEM)和扫描电镜(SEM)数据以及AutoCUTS-SEM和FIB-SEM三维数据;2)使用“Tokuyasu”冷冻切片复冻的方法对心肌组织制样获取的cryoET数据;3)用于对比参考的SD大鼠MRI成像数据;4)有关机器学习程序算法验证的前列腺病理光学显微镜图片以及手功能评估视频及新生儿运动评估视频图像等。.由于以往测量技术的限制,对脑ECS超微结构解析及其内间质液引流规律的研究一直是国际难题、业界挑战,目前尚未见有成熟方法和重要成果报道,本项目首次在国内搭建了ECS三维电镜结构定量测量的完整流程,取得了一些成果,为后续ECS相关研究提供有力支持。另外,本项目中收集的数据和已研发的程序算法可为其他领域应用提供借鉴参考或移植使用。.截至目前,已发表相关SCI、EI和Pubmed收录文章5篇,修稿SCI论文1篇,在审SCI论文1篇,申请并授权国内发明专利2项,国际会议口头报告2次,参与培养学生5人。
项目成果
期刊论文数量(5)
专著数量(0)
科研奖励数量(0)
会议论文数量(2)
专利数量(2)
冷冻电子显微镜成像中噪声的滤波方法进展
- DOI:--
- 发表时间:2021
- 期刊:北京大学学报(医学版)
- 影响因子:--
- 作者:黄新瑞;李莎;高嵩
- 通讯作者:高嵩
Original Article Synthesis of magnetic resonance images from computed tomography data using convolutional neural network with contextual loss function
使用具有上下文损失函数的卷积神经网络从 CT 数据合成 MR 图像
- DOI:10.21037/qims-21-846
- 发表时间:2022-02-23
- 期刊:QUANTITATIVE IMAGING IN MEDICINE AND SURGERY
- 影响因子:2.8
- 作者:Li,Zhaotong;Huang,Xinrui;Xia,Jun
- 通讯作者:Xia,Jun
Quantitative Measurement of Brain Extracellular Space with Three-Dimensional Electron Microscopy Imaging
三维电镜成像定量测量脑细胞外间隙
- DOI:10.1007/s11220-022-00408-z
- 发表时间:2023-01-03
- 期刊:SENSING AND IMAGING
- 影响因子:2.2
- 作者:Huang,Xinrui;Li,Kerui;Han,Hongbin
- 通讯作者:Han,Hongbin
Fusing hand-crafted and deep-learning features in a convolutional neural network model to identify prostate cancer in pathology images.
卷积神经网络模型中的深度学习特征可识别病理图像中的前列腺癌
- DOI:10.3389/fonc.2022.994950
- 发表时间:2022
- 期刊:Frontiers in oncology
- 影响因子:4.7
- 作者:
- 通讯作者:
数据更新时间:{{ journalArticles.updateTime }}
{{
item.title }}
{{ item.translation_title }}
- DOI:{{ item.doi || "--"}}
- 发表时间:{{ item.publish_year || "--" }}
- 期刊:{{ item.journal_name }}
- 影响因子:{{ item.factor || "--"}}
- 作者:{{ item.authors }}
- 通讯作者:{{ item.author }}
数据更新时间:{{ journalArticles.updateTime }}
{{ item.title }}
- 作者:{{ item.authors }}
数据更新时间:{{ monograph.updateTime }}
{{ item.title }}
- 作者:{{ item.authors }}
数据更新时间:{{ sciAawards.updateTime }}
{{ item.title }}
- 作者:{{ item.authors }}
数据更新时间:{{ conferencePapers.updateTime }}
{{ item.title }}
- 作者:{{ item.authors }}
数据更新时间:{{ patent.updateTime }}
其他文献
其他文献
{{
item.title }}
{{ item.translation_title }}
- DOI:{{ item.doi || "--" }}
- 发表时间:{{ item.publish_year || "--"}}
- 期刊:{{ item.journal_name }}
- 影响因子:{{ item.factor || "--" }}
- 作者:{{ item.authors }}
- 通讯作者:{{ item.author }}
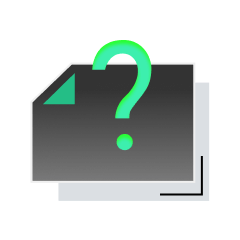
内容获取失败,请点击重试
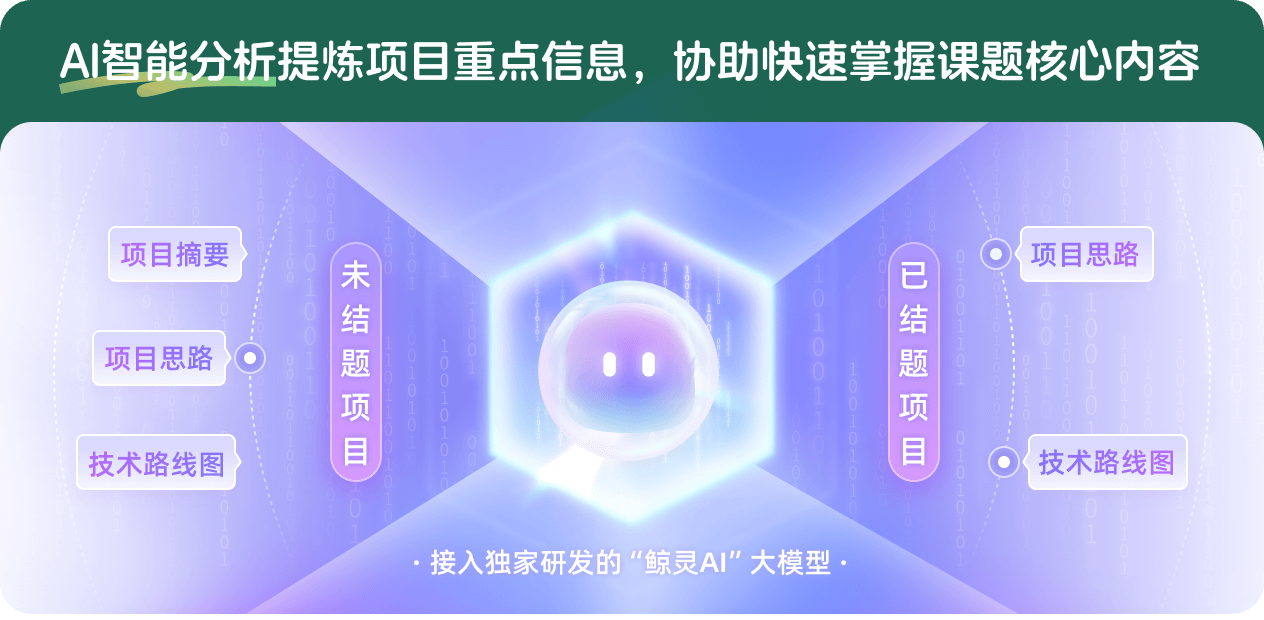
查看分析示例
此项目为已结题,我已根据课题信息分析并撰写以下内容,帮您拓宽课题思路:
AI项目摘要
AI项目思路
AI技术路线图
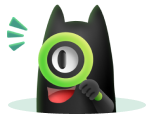
请为本次AI项目解读的内容对您的实用性打分
非常不实用
非常实用
1
2
3
4
5
6
7
8
9
10
您认为此功能如何分析更能满足您的需求,请填写您的反馈:
相似国自然基金
{{ item.name }}
- 批准号:{{ item.ratify_no }}
- 批准年份:{{ item.approval_year }}
- 资助金额:{{ item.support_num }}
- 项目类别:{{ item.project_type }}
相似海外基金
{{
item.name }}
{{ item.translate_name }}
- 批准号:{{ item.ratify_no }}
- 财政年份:{{ item.approval_year }}
- 资助金额:{{ item.support_num }}
- 项目类别:{{ item.project_type }}