大时滞生产过程的鲁棒抗扰控制与批次运行优化
项目介绍
AI项目解读
基本信息
- 批准号:61903060
- 项目类别:青年科学基金项目
- 资助金额:25.0万
- 负责人:
- 依托单位:
- 学科分类:F0301.控制理论与技术
- 结题年份:2022
- 批准年份:2019
- 项目状态:已结题
- 起止时间:2020-01-01 至2022-12-31
- 项目参与者:--
- 关键词:
项目摘要
To cope with the problems of poor anti-disturbance control performance and inconsistence of batch running associated with manufacturing processes with large time delay, this project investigates active disturbance rejection control (ADRC) methods based on delay-free response prediction and real-time disturbance estimation, and robust anti-disturbance batch control & running optimization methods based on historical batch data and iterative learning strategies. For the presence of delay uncertainty and process dynamic uncertainties, robust anti-disturbance control methods will be established to stabilize these uncertainties, and correspondingly, sufficient conditions for holding robust stability of the closed-loop system will be analyzed. For batch processes with delay response, robust anti-disturbance iterative learning control (ILC) methods will be developed based on historical batch information, to overcome adverse effect of repeatable load disturbance, such that perfect tracking of the desired output trajectory could be realized. Moreover, non-repeatable load disturbance, delay uncertainty and time-varying process uncertainties could be stabilized. Concerning batch processes with time delay that are difficult to model the dynamic response characteristics, robust data-driven ILC methods based on measured input/output data will be explored, and meanwhile, sufficient conditions for robustly tracking the desired output trajectory will be analyzed. The project results will give some new theory and application methods of robust anti-disturbance control and batch running optimization for manufacturing processes with large time delay, and therefore have reference significance in aspects of theoretical guidance and engineering application for improving industrial process control technologies.
针对大时滞生产过程存在抗扰性能较差和批次运行质量不一致的问题,研究基于无时滞响应预估和实时扰动估计的主动抗扰控制方法、基于历史批次数据和迭代学习策略的鲁棒抗扰批次控制与运行优化方法。对于存在时滞不确定性和过程动态不确定性的情况,建立能镇定这些不确定性的鲁棒抗扰控制方法,分析闭环控制系统保证鲁棒稳定性的充分条件。针对带有时滞响应的批次生产过程,发展基于历史批次信息的鲁棒抗扰迭代学习控制方法,以克服重复性负载干扰的不利影响,实现完全跟踪期望输出轨迹,而且能镇定非重复性负载干扰、时滞不确定性以及过程时变不确定性。对于难以建模动态响应特性的时滞响应批次生产过程,研究基于过程输入和输出测量数据的鲁棒数据驱动迭代学习控制方法,论证鲁棒跟踪期望输出轨迹的充分条件。本项目成果将给出大时滞生产过程鲁棒抗扰控制与批次运行优化的一些新理论与应用方法,对提高工业过程控制技术水平具有理论指导和工程应用参考意义。
结项摘要
工业生产过程中广泛存在着由物料传送、信号处理、长距离信号传输等引起的时滞现象。此外,实际生产过程中还存在由添加原理、产物输出、以及负荷变化等引起的干扰。这些不利因素往往会导致系统性能大幅下降,甚至造成闭环控制系统不稳定。如何针对时滞系统设计抗扰控制方法是过程控制领域具有挑战性的问题。对于批次生产过程,如何克服非重复过程不确定性以及时滞响应,实现鲁棒批次运行优化,也是过程控制领域亟待解决的难题。围绕这些难题和挑战,本项目取得了一批具有原创性的研究成果,主要包括:(i) 针对存在非对称饱和约束的输出时滞温度控制系统,提出基于无时滞输出预测的反饱和主动抗扰控制方法,显著提高大时滞系统的抗扰性能;(ii) 针对存在输入时滞的工业生产过程,提出基于预估器的主动抗扰控制方法;(iii) 针对存在时变和批次变化不确定性的批次生产过程,提出基于扩张状态观测器的间接型ILC方法,提高了被控过程的跟踪和抗扰性能;(iv) 针对存在非重复性负载干扰的批次过程,提出基于静态输出反馈的有限频域两维H无穷性能规范的ILC方法;(v)针对存在输出时滞响应的批次生产过程,提出基于预测状态观测器的间接型设定点学习控制方法;(vi) 针对存在非重复性负载干扰未知非线性批次生产过程,提出基于扰动估计和补偿的数据驱动迭代学习控制方法,提高了现有数据驱动迭代学习控制方法的抗扰性能。共计发表学术论文17篇,其中7篇发表于控制领域国际重要SCI期刊,申请授权国家发明专利2项,合作出版中文著作1部。
项目成果
期刊论文数量(7)
专著数量(1)
科研奖励数量(0)
会议论文数量(10)
专利数量(2)
Predictor-based phase-lead active disturbance rejection control design for industrial processes with input delay
基于预测器的输入延迟工业过程相位超前自抗扰控制设计
- DOI:10.3389/fcteg.2022.954164
- 发表时间:2022-09
- 期刊:Frontiers in Control Engineering
- 影响因子:--
- 作者:Xiaomeng Li;Shoulin Hao;Tao Liu;Bin Yan;Yongzhi Zhou
- 通讯作者:Yongzhi Zhou
Anti-windup ADRC design for temperature control systems with output delay against asymmetric input constraint
针对不对称输入约束具有输出延迟的温度控制系统的抗饱和 ADRC 设计
- DOI:10.1016/j.isatra.2023.01.001
- 发表时间:2023
- 期刊:ISA Transactions
- 影响因子:7.3
- 作者:Shoulin Hao;Tao Liu;Xinpeng Geng;Youqing Wang
- 通讯作者:Youqing Wang
Extended state observer based indirect-type ILC for single-input single-output batch processes with time- and batch-varying uncertainties
基于扩展状态观察器的间接型ILC,用于具有时变和批次不确定性的单输入单输出批处理
- DOI:10.1016/j.automatica.2019.108673
- 发表时间:2020-02
- 期刊:Automatica
- 影响因子:6.4
- 作者:Shoulin Hao;Tao Liu;Eric Rogers
- 通讯作者:Eric Rogers
Output feedback based robust iterative learning control via a heuristic approach for batch processes with time-varying state delays and uncertainties
通过具有时变状态延迟和不确定性的批处理的启发式方法,基于输出反馈的鲁棒迭代学习控制
- DOI:--
- 发表时间:2022
- 期刊:Journal of Process Control
- 影响因子:4.2
- 作者:Junyu Wei;Hongfeng Tao;Shoulin Hao;Wojciech Paszke;Krzysztof Galkowski
- 通讯作者:Krzysztof Galkowski
Predictive state observer based set-point learning control for batch manufacturing processes with delay response
基于预测状态观测器的设定点学习控制,用于具有延迟响应的批量制造过程
- DOI:10.1109/tie.2023.3245217
- 发表时间:--
- 期刊:IEEE Transactions on Industrial Electronics
- 影响因子:7.7
- 作者:Tao Liu;Shoulin Hao;Youqing Wang;Jing Na
- 通讯作者:Jing Na
数据更新时间:{{ journalArticles.updateTime }}
{{
item.title }}
{{ item.translation_title }}
- DOI:{{ item.doi || "--"}}
- 发表时间:{{ item.publish_year || "--" }}
- 期刊:{{ item.journal_name }}
- 影响因子:{{ item.factor || "--"}}
- 作者:{{ item.authors }}
- 通讯作者:{{ item.author }}
数据更新时间:{{ journalArticles.updateTime }}
{{ item.title }}
- 作者:{{ item.authors }}
数据更新时间:{{ monograph.updateTime }}
{{ item.title }}
- 作者:{{ item.authors }}
数据更新时间:{{ sciAawards.updateTime }}
{{ item.title }}
- 作者:{{ item.authors }}
数据更新时间:{{ conferencePapers.updateTime }}
{{ item.title }}
- 作者:{{ item.authors }}
数据更新时间:{{ patent.updateTime }}
其他文献
其他文献
{{
item.title }}
{{ item.translation_title }}
- DOI:{{ item.doi || "--" }}
- 发表时间:{{ item.publish_year || "--"}}
- 期刊:{{ item.journal_name }}
- 影响因子:{{ item.factor || "--" }}
- 作者:{{ item.authors }}
- 通讯作者:{{ item.author }}
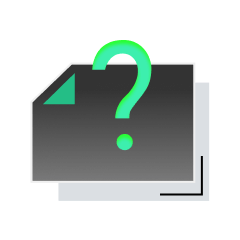
内容获取失败,请点击重试
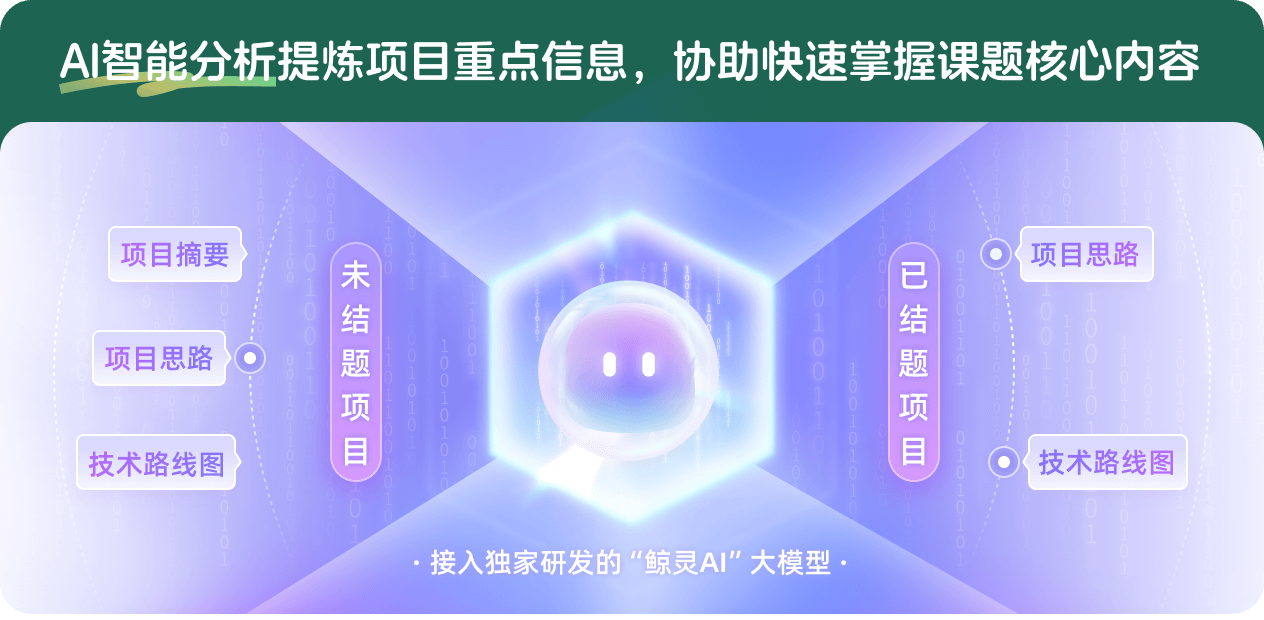
查看分析示例
此项目为已结题,我已根据课题信息分析并撰写以下内容,帮您拓宽课题思路:
AI项目摘要
AI项目思路
AI技术路线图
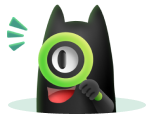
请为本次AI项目解读的内容对您的实用性打分
非常不实用
非常实用
1
2
3
4
5
6
7
8
9
10
您认为此功能如何分析更能满足您的需求,请填写您的反馈:
相似国自然基金
{{ item.name }}
- 批准号:{{ item.ratify_no }}
- 批准年份:{{ item.approval_year }}
- 资助金额:{{ item.support_num }}
- 项目类别:{{ item.project_type }}
相似海外基金
{{
item.name }}
{{ item.translate_name }}
- 批准号:{{ item.ratify_no }}
- 财政年份:{{ item.approval_year }}
- 资助金额:{{ item.support_num }}
- 项目类别:{{ item.project_type }}