基于稀疏优化的鲁棒多示例学习方法研究
项目介绍
AI项目解读
基本信息
- 批准号:61906201
- 项目类别:青年科学基金项目
- 资助金额:27.0万
- 负责人:
- 依托单位:
- 学科分类:F0603.机器学习
- 结题年份:2022
- 批准年份:2019
- 项目状态:已结题
- 起止时间:2020-01-01 至2022-12-31
- 项目参与者:--
- 关键词:
项目摘要
Multi-instance learning is one of the most newest research topics in the fields of both statistical data analysis and machine learning. This project aims at analyzing multi-instance data in the open learning environment by robust models from three aspects, i.e. in theory, methodology and application. In theoretical aspect, we will discuss the description mechanism of feature correlation of multi-instance data and the construction of the unified sparse optimization framework. In methodology, we will try to construct copula of correlated feature for multi-instance data based on the sparse and low rank constraints. Besides, by using previous constructed feature structure, we will construct the novel robust multi-instance learning methods based on sparse optimization to solve the challenges of feature and label changes of multi-instance data. Meanwhile, we will also design the specific algorithms to solve our proposed optimization problems. In applications, based on our previous results, we will dedicate to the functional annotation problem of protein coding isoforms in biomedical data mining. The above three aspects are tightly related. The researches in theory and methodology can provide useful guidance for applications. The researches in applications can provide real background for theoretical and methodological investigations. The works in this project can not only extend the research areas of traditional machine learning, but also provide meaningful guidance for the solution to problems in real applications.
多示例学习是近年来统计数据分析和机器学习中出现的一个新的研究课题。本项目以多示例学习为背景,针对开放学习环境下多示例数据特征关联结构理论、方法和应用问题开展研究。理论上主要探讨多示例数据特征相关性描述机理和稀疏优化统一框架构建问题。方法上,一方面,研究基于稀疏和低秩约束的多示例数据特征关联结构构建方法;另一方面,根据已构建的特征关联结构,研究针对标签缺失和标签变化情形的基于稀疏优化的鲁棒多示例学习方法,并基于稀疏优化框架设计其快速求解算法。应用中,在理论和方法的基础上主要研究生物医疗数据挖掘中的蛋白质编码异构体功能标注问题。这三个部分紧密相连,理论和方法研究为应用研究提供指导,应用研究为理论和方法研究提供背景。课题研究不仅能够丰富和拓展机器学习的理论和方法,同时对于解决实际中许多具体应用问题也有重要的指导意义。
结项摘要
多示例学习是是国际机器学习领域的重要研究课题之一,相关技术被广泛应用于图像分割、药物发现、生物医疗数据挖掘等领域。本项目以多示例学习为背景,针对开放学习环境下特征和标注信息不完全等问题开展了多示例数据特征关联结构理论、学习方法和实际应用等方面的研究,完成了原定研究计划,取得了诸多有价值的成果。主要包括:(1) 针对不同凸性和光滑条件的优化问题设计了重加权优化框架,并基于该框架设计了一系列快速优化算法;(2) 针对数据特征噪声干扰和缺失问题,提出了系列噪声鲁棒的特征学习方法和特征缺失的多示例学习方法;(3)针对标注信息缺失问题,提出了系列融合分布信息和单侧标记信息的多示例学习方法;(4)针对标注信息不足和数据不平衡问题,提出了系列标签信息增强方法;(5) 面向实际军事需求,开展了多项多示例学习方法在军事情报数据筛选领域的创新研究,有效性地解决了数据筛选中的一些关键问题,已发挥了显著的军事效益。项目研究期间共发表论文14篇,其中SCI检索11篇,CCF A 类期刊/会议(TPAMI、TKDE、中国科学F辑: 信息科学)4篇;B 类期刊/会议(Pattern Recognition、Frontiers of Computer Science和ICME)6篇。本项目成果已经引起国际同行的广泛关注,引用源包括IEEE TCYB, IEEE TNNLS, IEEE TAI, IEEE TETCI, Pattern Recognition, ACM MM和IJCAI等。在本项目资助下申请专利/软著6项,同时培养了多名研究生。
项目成果
期刊论文数量(12)
专著数量(0)
科研奖励数量(7)
会议论文数量(2)
专利数量(2)
Multi-view subspace learning via bidirectional sparsity
通过双向稀疏性进行多视图子空间学习
- DOI:10.1016/j.patcog.2020.107524
- 发表时间:2020-12
- 期刊:Pattern Recognition
- 影响因子:8
- 作者:Ruidong Fan;Tingjin Luo;Wenzhang Zhuge;Sheng Qiang;Chenping Hou
- 通讯作者:Chenping Hou
Joint Representation Learning and Clustering: A Framework for Grouping Partial Multiview Data
联合表示学习和聚类:对部分多视图数据进行分组的框架
- DOI:10.1109/tkde.2020.3028422
- 发表时间:2020-10
- 期刊:IEEE Transactions on Knowledge and Data Engineering
- 影响因子:8.9
- 作者:Zhuge Wenzhang;Tao Hong;Luo Tingjin;Zeng Ling-Li;Hou Chenping;Yi Dongyun
- 通讯作者:Yi Dongyun
Optimal Representative Distribution Margin Machine for Multi-instance Learning
多实例学习的最优代表分布裕度机
- DOI:10.1109/access.2020.2988764
- 发表时间:2020
- 期刊:IEEE Access
- 影响因子:3.9
- 作者:Tianxiang Luan;Tingjin Luo;Wenzhang Zhuge;Chenping Hou
- 通讯作者:Chenping Hou
基于独立自表达学习的不完全多视图聚类方法
- DOI:10.1360/ssi-2021-0070
- 发表时间:2021-09
- 期刊:中国科学: 信息科学
- 影响因子:--
- 作者:诸葛文章;范瑞东;罗廷金;陶红;侯臣平
- 通讯作者:侯臣平
Multi-View Spectral Clustering With Incomplete Graphs
不完全图的多视图谱聚类
- DOI:10.1109/access.2020.2997755
- 发表时间:2020
- 期刊:IEEE Access
- 影响因子:3.9
- 作者:Wenzhang Zhuge;Tingjin Luo;Hong Tao;Dongyun Yi;Chenping Hou
- 通讯作者:Chenping Hou
数据更新时间:{{ journalArticles.updateTime }}
{{
item.title }}
{{ item.translation_title }}
- DOI:{{ item.doi || "--"}}
- 发表时间:{{ item.publish_year || "--" }}
- 期刊:{{ item.journal_name }}
- 影响因子:{{ item.factor || "--"}}
- 作者:{{ item.authors }}
- 通讯作者:{{ item.author }}
数据更新时间:{{ journalArticles.updateTime }}
{{ item.title }}
- 作者:{{ item.authors }}
数据更新时间:{{ monograph.updateTime }}
{{ item.title }}
- 作者:{{ item.authors }}
数据更新时间:{{ sciAawards.updateTime }}
{{ item.title }}
- 作者:{{ item.authors }}
数据更新时间:{{ conferencePapers.updateTime }}
{{ item.title }}
- 作者:{{ item.authors }}
数据更新时间:{{ patent.updateTime }}
其他文献
其他文献
{{
item.title }}
{{ item.translation_title }}
- DOI:{{ item.doi || "--" }}
- 发表时间:{{ item.publish_year || "--"}}
- 期刊:{{ item.journal_name }}
- 影响因子:{{ item.factor || "--" }}
- 作者:{{ item.authors }}
- 通讯作者:{{ item.author }}
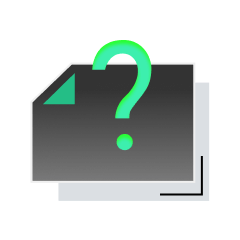
内容获取失败,请点击重试
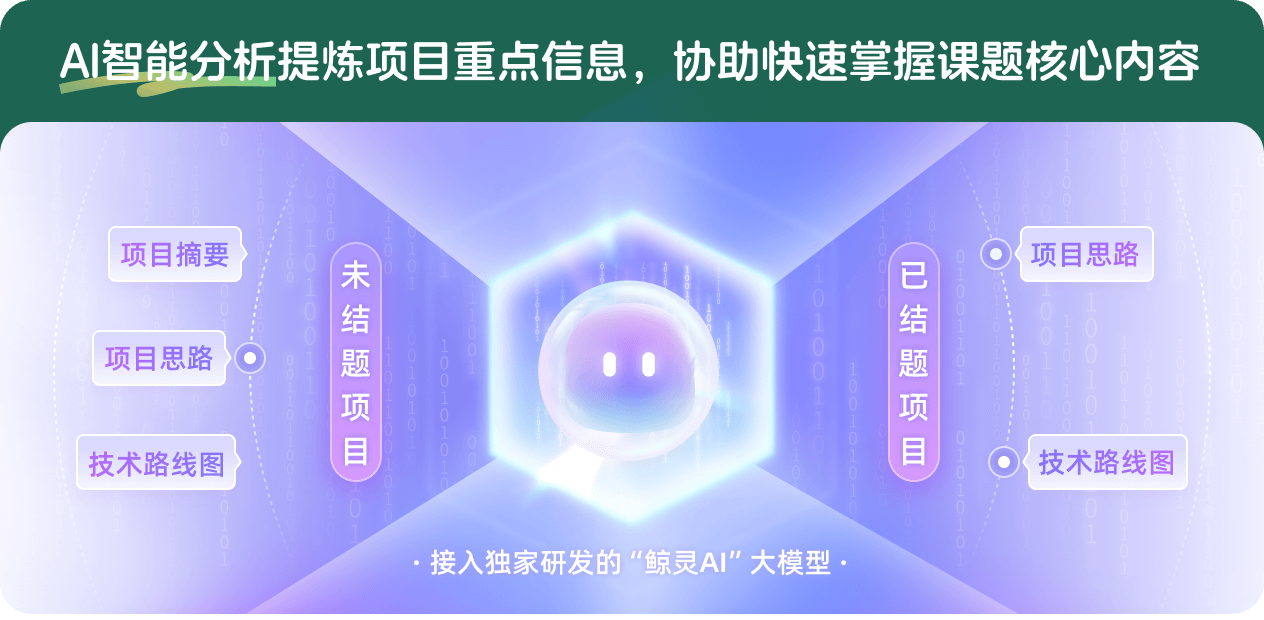
查看分析示例
此项目为已结题,我已根据课题信息分析并撰写以下内容,帮您拓宽课题思路:
AI项目摘要
AI项目思路
AI技术路线图
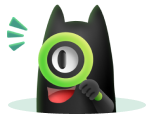
请为本次AI项目解读的内容对您的实用性打分
非常不实用
非常实用
1
2
3
4
5
6
7
8
9
10
您认为此功能如何分析更能满足您的需求,请填写您的反馈:
罗廷金的其他基金
面向开源情报分析的动态多标签学习理论与方法
- 批准号:62376281
- 批准年份:2023
- 资助金额:50 万元
- 项目类别:面上项目
相似国自然基金
{{ item.name }}
- 批准号:{{ item.ratify_no }}
- 批准年份:{{ item.approval_year }}
- 资助金额:{{ item.support_num }}
- 项目类别:{{ item.project_type }}
相似海外基金
{{
item.name }}
{{ item.translate_name }}
- 批准号:{{ item.ratify_no }}
- 财政年份:{{ item.approval_year }}
- 资助金额:{{ item.support_num }}
- 项目类别:{{ item.project_type }}