面向多源高维数据流的在线特征选择与分类方法研究
项目介绍
AI项目解读
基本信息
- 批准号:61673152
- 项目类别:面上项目
- 资助金额:61.0万
- 负责人:
- 依托单位:
- 学科分类:F0605.模式识别与数据挖掘
- 结题年份:2020
- 批准年份:2016
- 项目状态:已结题
- 起止时间:2017-01-01 至2020-12-31
- 项目参与者:李培培; 李磊; 胡骏; 周鹏; 朱毅; 吕俊伟; 何俊宏; 储光;
- 关键词:
项目摘要
Classification on data streams has been widely applied in the real-world applications, such as the network monitoring and sensor network, to name a few. However, the real-world data streams such as traffic network monitoring data present new characteristics as being multi-sourcing, high-dimensional and sparse, high-volume, high-velocity and multivariate. It is hence a challenge for traditional approaches of feature selection and classification due to the poor performance in the time overhead and the accuracy. Therefore, in this proposal, we focus on the study of online feature selection and classification approaches for high-dimensional data stream from mutli-data sources. More specifically, in terms of the study in the formalization of the feature vector and the data fusion oriented to the multi-source and high-dimensional data streams, we address the study in the theory and methods of feature ranking based on online filtering feature selection techniques, and in the online model updating, cost evalution and model evaluation based on the embeded feature selection techniques. In addition, considering the impact from the concept drift and evolution caused by the distribution changes of feature rankings and labels, we further study the detection and prediction methods of concept drift and evolution, and propose the corresponding evaluation measures. We aim to sovle the isses in high dimensional data streams from multi-data srouces, and meanwhile we want to provide a new approach to the classification of high dimensional data streams in real-world applications. Lastly, we apply our approaches into labeling traffic congestion states and detecting the drift and evolution of congestion events from the traffic monitoring data streams, and design a prototype classification system for high dimensional data streams to validate the effectiveness of our appraoches.
数据流分类在网络监控、传感器网络等实际领域有着广泛应用。然而,实际数据流如交通网络监控数据具有多源性、高维稀疏、海量快速、多变等特点,使得传统的特征选择与分类方法难以满足时间与精度要求,因此,本课题拟开展面向多源高维数据流的在线特征选择与分类方法研究。在多源海量数据流的特征空间形式化表示与数据融合的研究工作基础上,重点开展基于过滤式在线特征选择策略的特征排序理论与方法研究,以及基于嵌入式特征选择策略的在线分类模型的构建、更新、代价评估与模型评估等方面的研究。此外,考虑由于特征排序分布或类分布变化引发的概念漂移与演化问题对分类模型的影响,研究高维数据流环境下的概念漂移与演化的检测与预测方法及其评价标准,为实际应用领域中多源高维数据流的分类提供新的解决途径;基于上述研究,设计并实现面向交通网络监测数据流的交通拥堵状态分类与拥堵事件漂移与演化检测的数据流分类原型系统,以此验证理论与方法的有效性。
结项摘要
实际应用领域产生大量的数据流,它们呈现出多源、海量、快速、无限等特点,更显著的特点是特征高维稀疏、隐含语义信息低质、概念漂移等。因此,针对多源数据流开展分类任务的研究,以寻求适应多源数据流的在线模型、解决特征高维稀疏、语义低质、概念漂移等问题,具有重要研究意义和实际应用价值。目前我们主要从以下七个方面开展工作,包括:多源海量数据的获取与实体识别研究、海量数据的特征表示方法研究、流特征选择方法研究、多源数据的因果发现方法研究、数据流概念模型与概念漂移检测模型研究、海量数据分类方法及其应用研究,上述研究成果为解决多源数据流中的多源数据获取与表示、特征高维稀疏、概念漂移等难题提供了更多的理论依据和更有效的技术途径。同时,相关研究成果在实际应用领域如:复杂网络社团预测、煤与瓦斯突出预测及新闻网页与专利推荐等进行了探索,为多源数据流的特征选择与在线分类方法的研究贡献了新的理论、机制和方法,创造了实际应用价值。研究工作按照预定计划,取得的研究成果总结如下:. (1) 理论方法上强调创新:在国内外重要学术刊物和会议上发表高质量论文40篇,其中,已发表SCI检索期刊论文23篇(其中IEEE/ACM Trans. 论文4篇)、EI会议论文4篇、国内核心期刊论文13篇(其中,中国科学: 信息科学(中文版)1篇、情报学报1篇、模式识别与人工智能1篇);. (2) 基础研究可持续性:在此项目研究工作的基础上,课题主持人成功延续获批1 项国家基金面上项目(No. 62076085)、课题参与人李培培与李磊成功获批2项国家基金面上项目(No. 61976077与No. 62076087)、课题参与人周鹏与朱毅成功获批2项国家基金青年基金项目(No. 61906056与No. 61906060); . (3) 技术方法在实际应用领域的探索与应用,提高其社会效益:获得授权专利8项;. (4) 人才培养:培养博士生7名、硕士生10名。
项目成果
期刊论文数量(36)
专著数量(0)
科研奖励数量(0)
会议论文数量(4)
专利数量(8)
Wasserstein GAN based on Autoencoder with back-translation for cross-lingual embedding mappings
基于自动编码器的 Wasserstein GAN,具有跨语言嵌入映射的反向翻译
- DOI:10.1016/j.patrec.2019.11.033
- 发表时间:2020
- 期刊:Pattern Recognition Letters
- 影响因子:5.1
- 作者:Zhang Yuhong;Li Yuling;Zhu Yi;Hu Xuegang
- 通讯作者:Hu Xuegang
Learning Markov Blankets From Multiple Interventional Data Sets
从多个介入数据集中学习马尔可夫毯子
- DOI:10.1109/tnnls.2019.2927636
- 发表时间:2020-06
- 期刊:IEEE Transactions on Neural Networks and Learning Systems
- 影响因子:10.4
- 作者:Yu Kui;Liu Lin;Li Jiuyong
- 通讯作者:Li Jiuyong
BAMB: A Balanced Markov Blanket Discovery Approach to Feature Selection
BAMB:用于特征选择的平衡马尔可夫毯子发现方法
- DOI:10.1145/3335676
- 发表时间:2019
- 期刊:ACM Transactions on Intelligent Systems and Technology
- 影响因子:5
- 作者:Zhaolong Ling;Kui Yu;Hao Wang;Lin Liu;Wei Ding;Xindong Wu
- 通讯作者:Xindong Wu
Document-specific keyphrase candidate search and ranking
特定于文档的关键词候选搜索和排名
- DOI:10.1016/j.eswa.2017.12.031
- 发表时间:2018-05
- 期刊:Expert Systems with Applications
- 影响因子:8.5
- 作者:Wang Qingren;Sheng Victor S;Wu Xindong
- 通讯作者:Wu Xindong
An Effective Confidence-Based Early Classification of Time Series
一种有效的基于置信度的时间序列早期分类
- DOI:10.1109/access.2019.2929644
- 发表时间:2019-01-01
- 期刊:IEEE ACCESS
- 影响因子:3.9
- 作者:Lv, Junwei;Hu, Xuegang;Li, Peipei
- 通讯作者:Li, Peipei
数据更新时间:{{ journalArticles.updateTime }}
{{
item.title }}
{{ item.translation_title }}
- DOI:{{ item.doi || "--"}}
- 发表时间:{{ item.publish_year || "--" }}
- 期刊:{{ item.journal_name }}
- 影响因子:{{ item.factor || "--"}}
- 作者:{{ item.authors }}
- 通讯作者:{{ item.author }}
数据更新时间:{{ journalArticles.updateTime }}
{{ item.title }}
- 作者:{{ item.authors }}
数据更新时间:{{ monograph.updateTime }}
{{ item.title }}
- 作者:{{ item.authors }}
数据更新时间:{{ sciAawards.updateTime }}
{{ item.title }}
- 作者:{{ item.authors }}
数据更新时间:{{ conferencePapers.updateTime }}
{{ item.title }}
- 作者:{{ item.authors }}
数据更新时间:{{ patent.updateTime }}
其他文献
字符分布特征对带有通配符串匹配问题的影响
- DOI:--
- 发表时间:2015
- 期刊:计算机应用研究
- 影响因子:--
- 作者:王海平;郭丹;项泰宁;胡学钢
- 通讯作者:胡学钢
一种面向高维数据的迭代式Lasso特征选择方法
- DOI:--
- 发表时间:--
- 期刊:计算机应用研究
- 影响因子:--
- 作者:施万锋;胡学钢;俞奎;SHI Wan-feng,HU Xue-gang,YU Kui(School of Computer
- 通讯作者:SHI Wan-feng,HU Xue-gang,YU Kui(School of Computer
面向评论信息的跨领域词汇情感倾向判别方法
- DOI:--
- 发表时间:--
- 期刊:计算机科学
- 影响因子:--
- 作者:吴斐;张玉红;胡学钢
- 通讯作者:胡学钢
基于自身特征扩展的短文本分类方法
- DOI:--
- 发表时间:2016
- 期刊:计算机应用研究
- 影响因子:--
- 作者:胡学钢;杨超群;张玉红
- 通讯作者:张玉红
基于同义实体识别的 Web 信息集成
- DOI:--
- 发表时间:2015
- 期刊:计算机系统应用
- 影响因子:--
- 作者:徐喆昊;吴共庆;胡学钢
- 通讯作者:胡学钢
其他文献
{{
item.title }}
{{ item.translation_title }}
- DOI:{{ item.doi || "--" }}
- 发表时间:{{ item.publish_year || "--"}}
- 期刊:{{ item.journal_name }}
- 影响因子:{{ item.factor || "--" }}
- 作者:{{ item.authors }}
- 通讯作者:{{ item.author }}
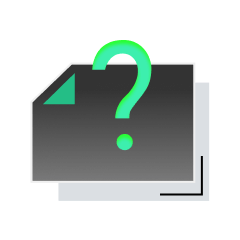
内容获取失败,请点击重试
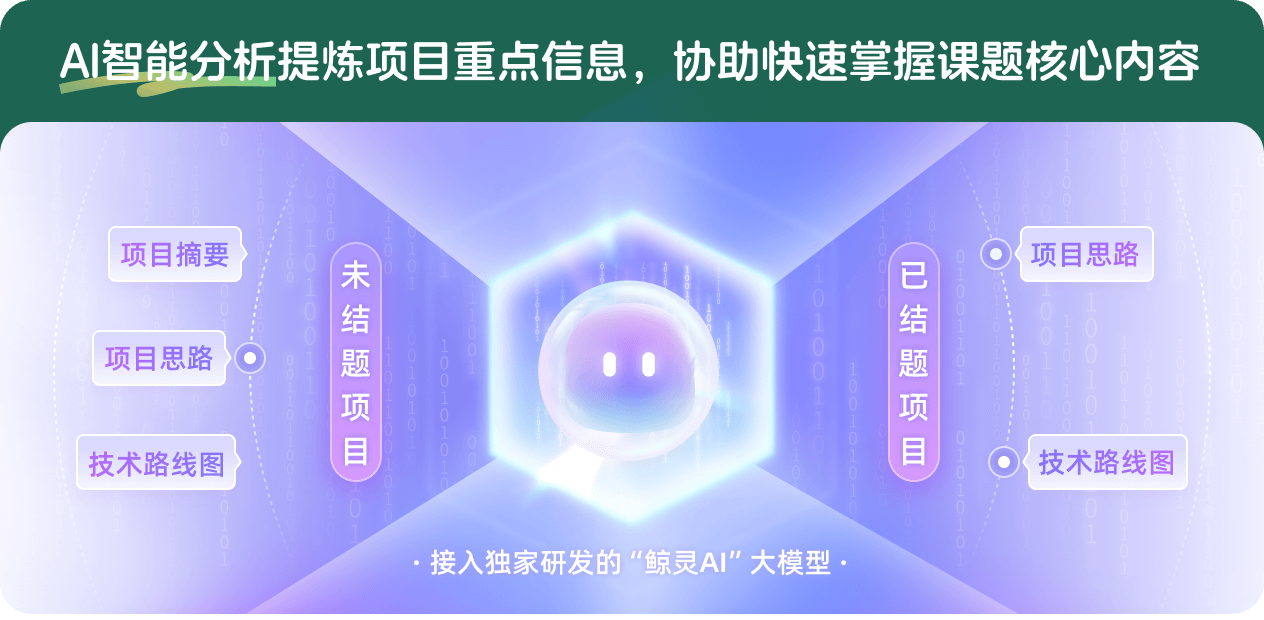
查看分析示例
此项目为已结题,我已根据课题信息分析并撰写以下内容,帮您拓宽课题思路:
AI项目摘要
AI项目思路
AI技术路线图
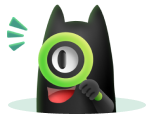
请为本次AI项目解读的内容对您的实用性打分
非常不实用
非常实用
1
2
3
4
5
6
7
8
9
10
您认为此功能如何分析更能满足您的需求,请填写您的反馈:
胡学钢的其他基金
多源数据流的半监督分类方法研究
- 批准号:62076085
- 批准年份:2020
- 资助金额:59 万元
- 项目类别:面上项目
基于协同训练策略的不完全标记数据流分类问题研究
- 批准号:61273292
- 批准年份:2012
- 资助金额:80.0 万元
- 项目类别:面上项目
基于特征发现的数据流概念漂移问题研究
- 批准号:60975034
- 批准年份:2009
- 资助金额:32.0 万元
- 项目类别:面上项目
相似国自然基金
{{ item.name }}
- 批准号:{{ item.ratify_no }}
- 批准年份:{{ item.approval_year }}
- 资助金额:{{ item.support_num }}
- 项目类别:{{ item.project_type }}
相似海外基金
{{
item.name }}
{{ item.translate_name }}
- 批准号:{{ item.ratify_no }}
- 财政年份:{{ item.approval_year }}
- 资助金额:{{ item.support_num }}
- 项目类别:{{ item.project_type }}