张量最优化算法及其在基因表达数据中的应用
项目介绍
AI项目解读
基本信息
- 批准号:11301436
- 项目类别:青年科学基金项目
- 资助金额:22.0万
- 负责人:
- 依托单位:
- 学科分类:A0405.连续优化
- 结题年份:2016
- 批准年份:2013
- 项目状态:已结题
- 起止时间:2014-01-01 至2016-12-31
- 项目参与者:张树中; 吴小惠; 曾勇; 陈珍珍; 唐芸;
- 关键词:
项目摘要
Much research attention has been attracted by using tensor as a tool to analyze and study real problems. It has applications in a broad range of areas, including computer vision, data mining, signal processing, neuroscience, graphical analysis, biomedical engineering, quantum mechanics, etc. Tensor decomposition and approximation is one of the important analyzing methods there. Tensor calculus is a new branch of applied mathematics, which has close relationship with polynomial optimization and tensor optimization problems. The project focuses on establishing the optimization models of tensor decomposition and approximation problems, revealing the inherent nature of complex structured tensors, and analyzing gene expression data in bioinformatics, while the optimization problems involved are all NP-hard in general. Up till now, the algorithms with convergence and efficiency for solving tensor decomposition and approximation problems are rare, and the practical applications found in the area of bioinformatics are still lack. Based on these, this project aspires to provide some easy-to-implement and efficient algorithms with convergence guaranteed, design new tensor optimization models for solving real problems, and build the bridge between tensor optimization and polynomial optimization problems, which will bring both profound theoretical significance and extensive application prospects, and at the same time enrich theories and algorithms of some related issues, and then promote the development of related disciplines.
以张量为工具来分析和研究现实中的问题越来越受到关注,其应用领域非常广泛,包括计算机视觉、数据挖掘、信号处理、神经系统科学、图形分析,生物医学工程、量子力学等等,张量分解与逼近问题是重要的分析依据之一。张量计算是应用数学中一个新的分支,它与多项式优化、张量优化等问题紧密相联。本项目研究的重点在于构造张量分解与逼近的优化模型、揭示张量复杂结构下蕴含的性质、应用于生物信息学中基因表达数据的分析,其中所涉及的优化问题都为NP困难的。目前国内外研究在求解张量分解与逼近问题中具备收敛性且高效性的算法尚不多见,在新兴学科生物信息学方面的实际应用缺乏。本项目立志于给出操作性强、运行时间快且有收敛性保证的算法,设计新的张量优化模型以解决实际中的问题,搭建张量优化与多项式优化之间的桥梁,这一研究既有理论上的深刻性又有应用前景的广泛性,同时充实相关问题的理论与算法,促进相关学科的发展。
结项摘要
以张量为工具来分析和研究现实中的问题越来越受到关注,其应用领域非常广泛,包括计算机视觉、数据挖掘、信号处理、神经系统科学、图形分析,生物医学工程、量子力学等等,张量分解与逼近问题是重要的分析依据之一。张量计算是应用数学中一个新的分支,它与多项式优化、张量优化等问题紧密相联。目前国内外研究在求解张量分解与逼近问题中具备收敛性且高效性的算法尚不多见,在新兴学科生物信息学方面的实际应用缺乏。为此,本项目研究的重点在于:张量最佳低秩逼近中的优化模型、揭示张量复杂结构下蕴含的性质、应用基因表达数据的分析,其中所涉及的优化问题都为NP困难的。经过三年的研究工作,本项目在这三个主要研究内容下取得的重要成果为:1)Tucker分解算法的收敛性保证;基于Tucker分解的新模型的构建、求解与应用,已将模型成功应用到基因表达数据、图像处理等问题;2)新的三类张量的概念的提出(co-quadratic nonnegativity, M-quasiconvexity, co-quadratic M-quasiconvexity)以及相关理论性分析,搭建张量优化与多项式优化之间的桥梁,并证明了:如果非负对称张量是M-quasiconvex的,那么在任意约束条件下,此张量对应齐次多项式最大值问题等价于其张量松弛模型的最大值问题(这一理论上的保证为这一类特殊的多项式优化模型提供了寻找驻点的更为简单的方法),已成功解决张量最大特征值问题、电路设计中的双二次分配问题、投资组合问题等。本项目的部分研究成果已被葡萄牙学者应用到事件检测中。在接下来的研究中,我们将以张量为研究工具,解决多智能体预测状态表示以及热点推荐系统中的问题。这一研究既有理论上的深刻性又有应用前景的广泛性,同时充实相关问题的理论与算法,促进相关学科的发展。
项目成果
期刊论文数量(3)
专著数量(0)
科研奖励数量(0)
会议论文数量(1)
专利数量(0)
On Optimal Low Rank Tucker Approximation for Tensors: the Case for An Adjustable Core Size
关于张量的最优低阶塔克近似:可调整核心尺寸的情况
- DOI:10.1007/s10898-014-0231-x
- 发表时间:2014-08
- 期刊:Journal of Global Optimization
- 影响因子:1.8
- 作者:Bilian Chen;Zhening Li;Shuzhong Zhang
- 通讯作者:Shuzhong Zhang
Dilemma of Personal POI Recommendation in Location-based Social Networks
基于位置的社交网络中个人POI推荐的困境
- DOI:--
- 发表时间:--
- 期刊:Lecture Notes in Computer Science
- 影响因子:--
- 作者:Shenbao Yu;Bilian Chen;Jing Tang
- 通讯作者:Jing Tang
数据更新时间:{{ journalArticles.updateTime }}
{{
item.title }}
{{ item.translation_title }}
- DOI:{{ item.doi || "--"}}
- 发表时间:{{ item.publish_year || "--" }}
- 期刊:{{ item.journal_name }}
- 影响因子:{{ item.factor || "--"}}
- 作者:{{ item.authors }}
- 通讯作者:{{ item.author }}
数据更新时间:{{ journalArticles.updateTime }}
{{ item.title }}
- 作者:{{ item.authors }}
数据更新时间:{{ monograph.updateTime }}
{{ item.title }}
- 作者:{{ item.authors }}
数据更新时间:{{ sciAawards.updateTime }}
{{ item.title }}
- 作者:{{ item.authors }}
数据更新时间:{{ conferencePapers.updateTime }}
{{ item.title }}
- 作者:{{ item.authors }}
数据更新时间:{{ patent.updateTime }}
其他文献
关于非线性方程组 Householder 迭代法的一个注记
- DOI:--
- 发表时间:--
- 期刊:Applied Mathematics and Computation
- 影响因子:4
- 作者:陈碧连;马昌凤
- 通讯作者:马昌凤
其他文献
{{
item.title }}
{{ item.translation_title }}
- DOI:{{ item.doi || "--" }}
- 发表时间:{{ item.publish_year || "--"}}
- 期刊:{{ item.journal_name }}
- 影响因子:{{ item.factor || "--" }}
- 作者:{{ item.authors }}
- 通讯作者:{{ item.author }}
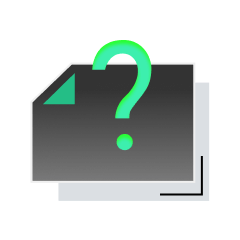
内容获取失败,请点击重试
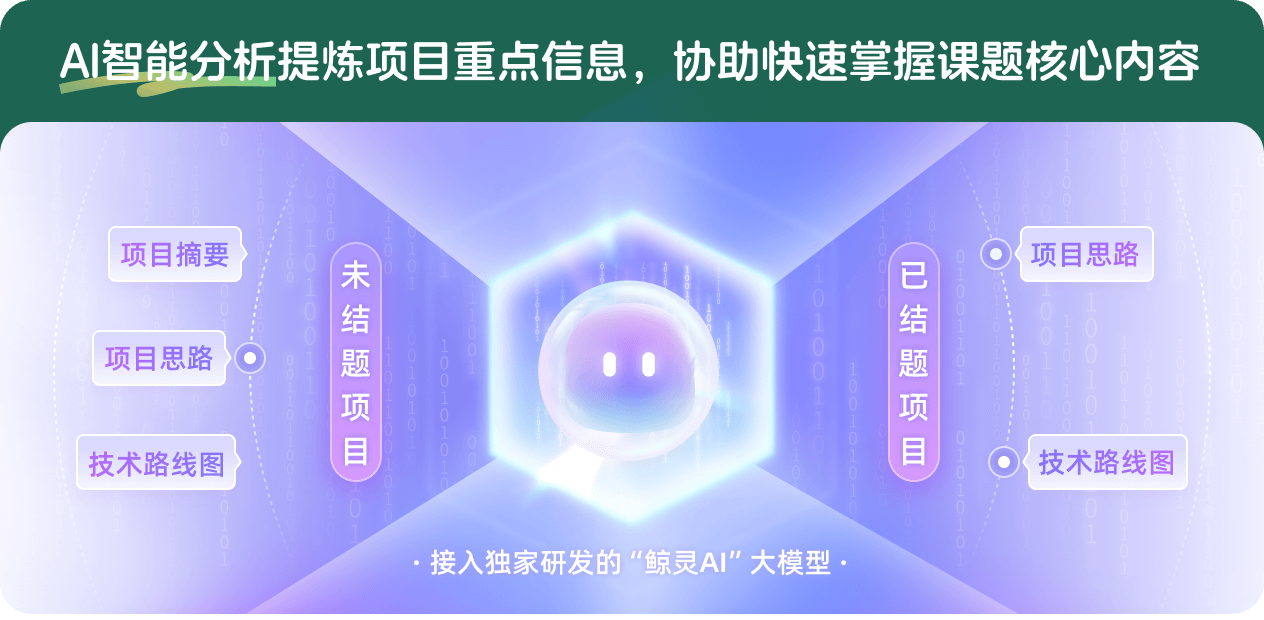
查看分析示例
此项目为已结题,我已根据课题信息分析并撰写以下内容,帮您拓宽课题思路:
AI项目摘要
AI项目思路
AI技术路线图
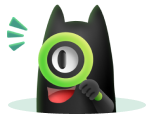
请为本次AI项目解读的内容对您的实用性打分
非常不实用
非常实用
1
2
3
4
5
6
7
8
9
10
您认为此功能如何分析更能满足您的需求,请填写您的反馈:
陈碧连的其他基金
面向高维稀疏数据的特征交互选择:张量优化理论与算法
- 批准号:12371515
- 批准年份:2023
- 资助金额:43.5 万元
- 项目类别:面上项目
相似国自然基金
{{ item.name }}
- 批准号:{{ item.ratify_no }}
- 批准年份:{{ item.approval_year }}
- 资助金额:{{ item.support_num }}
- 项目类别:{{ item.project_type }}
相似海外基金
{{
item.name }}
{{ item.translate_name }}
- 批准号:{{ item.ratify_no }}
- 财政年份:{{ item.approval_year }}
- 资助金额:{{ item.support_num }}
- 项目类别:{{ item.project_type }}