Multi-modal data integration to identify kinase substrates
多模式数据集成识别激酶底物
基本信息
- 批准号:10659156
- 负责人:
- 金额:$ 50.7万
- 依托单位:
- 依托单位国家:美国
- 项目类别:
- 财政年份:2022
- 资助国家:美国
- 起止时间:2022-07-05 至 2024-06-30
- 项目状态:已结题
- 来源:
- 关键词:
项目摘要
PROJECT SUMMARY
Kinases are involved in a variety of physiological functions, such as signal transduction, transcription,
development, and cell cycle regulation. Thus, dysregulation of protein kinases is associated with a range of
diseases, including cancer, metabolic diseases, and central nervous system disorders. More than 60 drugs
targeting kinases have been approved by the FDA, making them one of the most druggable protein families.
Despite their biomedical importance, a large group of human protein kinases remains highly understudied. These
proteins, often referred to as “dark kinases”, including by the Illuminating the Druggable Genome (IDG), have
limited knowledge of their substrate(s), which ultimately determine their cellular function. To address this
challenge, we will develop a novel computational framework to predict kinase-substrate interactions by
combining biologically relevant multi-modal data sources with cutting-edge machine learning methodologies.
Specifically, we will first derive features that quantify potential interactions between kinases and substrates from
diverse data sources, such as protein structure and dynamics, gene expression profiles, protein-protein and
protein-small molecule interaction networks, and evolutionary information (Aim 1). We will then develop
predictors of kinase-substrate interactions using an powerful machine learning methodology named Ensemble
Integration (EI; Aim 2). EI is based on the concept of heterogeneous ensembles that can aggregate an
unrestricted number and variety of base predictors derived from the above diverse data sources, and can benefit
from both the consensus and the diversity among these predictors. Due to its flexibility, EI is able to produce
more accurate predictions from multi-modal datasets than other established data integration methodologies, as
is expected for our project as well. Finally, we will evaluate the kinase-substrate interactions predicted by the EI-
based predictive model developed in Aim 2 using both computational and experimental methods (Aim 3). We
will also share the experimentally validated interactions, the most confident predictions from the EI model, and
all the data and software generated during this project through our KinaMetrix web server, as well as other public
data and software repositories. At its culmination, this project will produce novel and validated computational
methods and software to predict substrates of kinases, validated and high-confidence kinase-substrate
interactions for IDG dark kinases, and a public web server (KinaMetrix) to share these products. We expect that
these products will be highly useful for the study of dark kinases, especially in the IDG effort, as well as to better
understand kinase function and improve their utilization in drug development efforts. Our approach is also
expected to be generally applicable to other druggable protein families, such as ion channels and GPCRs.
项目摘要
激酶参与多种物理功能,例如信号转导,转录,
开发和细胞周期调节。那就是蛋白激酶的失调与一系列
包括癌症,代谢疾病和中枢神经系统疾病在内的疾病。超过60种药物
靶向激酶已得到FDA的批准,使其成为最可吸毒的蛋白质家族之一。
尽管具有生物医学的重要性,但大量的人类蛋白激酶仍然高度理解。这些
蛋白质通常称为“黑暗激酶”,包括通过照明的可毒基因组(IDG)具有
对其底物的了解有限,最终决定了其细胞功能。解决这个问题
挑战,我们将开发一个新颖的计算框架,以通过
将生物学相关的多模式数据源与尖端的机器学习方法相结合。
具体而言,我们将首先得出能够量化激酶与底物之间潜在相互作用的特征
多种数据源,例如蛋白质结构和动力学,基因表达谱,蛋白质 - 蛋白质和
蛋白质 - 小分子相互作用网络和进化信息(AIM 1)。然后我们将发展
使用功能强大的机器学习方法的集合的激酶 - 底物相互作用的预测指标
集成(EI; AIM 2)。 EI基于可以汇总的异质合奏的概念
从上述潜水员数据来源得出的不受限制数量和种类的基本预测因素,并且可以受益
从这些预测指标之间的共识和多样性中。由于其灵活性,EI能够产生
与其他已建立的数据集成方法相比,来自多模式数据集的更准确的预测
我们的项目也期望。最后,我们将评估EI所预测的激酶 - 基底相互作用
在AIM 2中使用计算和实验方法开发的基于基于的预测模型(AIM 3)。我们
还将共享经过实验验证的互动,EI模型中最自信的预测以及
通过我们的Kinametrix Web服务器以及其他公众,该项目期间生成的所有数据和软件
数据和软件存储库。在其高潮时,该项目将产生新颖且经过验证的计算
预测激酶底物的方法和软件
IDG深色激酶和公共Web服务器(Kinametrix)共享这些产品的交互。我们期望这一点
这些产品对于研究深色激酶的研究将非常有用,尤其是在IDG工作中,以及更好
了解激酶功能并改善其在药物开发工作中的利用。我们的方法也是
预计通常适用于其他可吸毒蛋白质家族,例如离子通道和GPCR。
项目成果
期刊论文数量(0)
专著数量(0)
科研奖励数量(0)
会议论文数量(0)
专利数量(0)
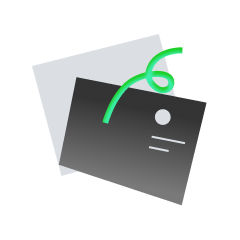
暂无数据
数据更新时间:2024-06-01
Gaurav Pandey的其他基金
Multi-modal data integration to identify kinase substrates
多模式数据集成识别激酶底物
- 批准号:1045194110451941
- 财政年份:2022
- 资助金额:$ 50.7万$ 50.7万
- 项目类别:
Integrating genomic and clinical data to predict disease phenotypes using heterogeneous ensembles
使用异质集合整合基因组和临床数据来预测疾病表型
- 批准号:1021876610218766
- 财政年份:2021
- 资助金额:$ 50.7万$ 50.7万
- 项目类别:
Integrating genomic and clinical data to predict disease phenotypes using heterogeneous ensembles
使用异质集合整合基因组和临床数据来预测疾病表型
- 批准号:1058982710589827
- 财政年份:2021
- 资助金额:$ 50.7万$ 50.7万
- 项目类别:
Integrating genomic and clinical data to predict disease phenotypes using heterogeneous ensembles
使用异质集合整合基因组和临床数据来预测疾病表型
- 批准号:1040975510409755
- 财政年份:2021
- 资助金额:$ 50.7万$ 50.7万
- 项目类别:
Boosting the Translational Impact of Scientific Competitions by Ensemble Learning
通过集成学习提升科学竞赛的转化影响
- 批准号:88646798864679
- 财政年份:2015
- 资助金额:$ 50.7万$ 50.7万
- 项目类别:
相似国自然基金
拟南芥中EIN2蛋白调控mRNA翻译并激活乙烯信号的生化机制研究
- 批准号:31870254
- 批准年份:2018
- 资助金额:60.0 万元
- 项目类别:面上项目
大米蛋白/阿魏酸的结合机制对复合物的抗氧化及模拟胃肠消化性能的调控研究
- 批准号:31760433
- 批准年份:2017
- 资助金额:38.0 万元
- 项目类别:地区科学基金项目
拟南芥fimbrin5调控花粉管生长的细胞学基础和生化机制分析
- 批准号:31671390
- 批准年份:2016
- 资助金额:60.0 万元
- 项目类别:面上项目
拟南芥微丝解聚因子第三亚家族成员生理生化功能研究
- 批准号:31670180
- 批准年份:2016
- 资助金额:65.0 万元
- 项目类别:面上项目
结合神经分类的分子超光谱成像生化指标定量分析研究
- 批准号:61240006
- 批准年份:2012
- 资助金额:10.0 万元
- 项目类别:专项基金项目
相似海外基金
DNA repair pathway coordination during damage processing
损伤处理过程中 DNA 修复途径的协调
- 批准号:1074847910748479
- 财政年份:2024
- 资助金额:$ 50.7万$ 50.7万
- 项目类别:
Endothelial Cell Reprogramming in Familial Intracranial Aneurysm
家族性颅内动脉瘤的内皮细胞重编程
- 批准号:1059540410595404
- 财政年份:2023
- 资助金额:$ 50.7万$ 50.7万
- 项目类别:
Selective targeting of matrix metalloproteinases for developing preterm labor therapeutics
选择性靶向基质金属蛋白酶用于开发早产疗法
- 批准号:1050978610509786
- 财政年份:2023
- 资助金额:$ 50.7万$ 50.7万
- 项目类别:
Role of skeletal muscle IPMK in nutrient metabolism and exercise
骨骼肌IPMK在营养代谢和运动中的作用
- 批准号:1063907310639073
- 财政年份:2023
- 资助金额:$ 50.7万$ 50.7万
- 项目类别:
The role of ZCWPW1 in meiosis
ZCWPW1 在减数分裂中的作用
- 批准号:1068018910680189
- 财政年份:2023
- 资助金额:$ 50.7万$ 50.7万
- 项目类别: