Statistical methods for large n and p problems
大型 n 和 p 问题的统计方法
基本信息
- 批准号:8019742
- 负责人:
- 金额:$ 37.21万
- 依托单位:
- 依托单位国家:美国
- 项目类别:
- 财政年份:2010
- 资助国家:美国
- 起止时间:2010-09-30 至 2014-08-31
- 项目状态:已结题
- 来源:
- 关键词:
项目摘要
DESCRIPTION (provided by applicant): Abstract Modern observational and experimental biological data has undergone a revolution. Driven by new biotechnology and computing advances, high dimensional, high density, functional multilevel and longitudinal biological signals are becoming commonplace in medical and public health research. These types of signals historically occurred in small clinical or experimental settings, often referred to as the "small n, large p" problem. We view the extension of these biological signals to cohort studies with longitudinal or hierarchical structure as a next generation of biostatistical problems. We've taken to calling this the "hierarchical large n, large p" problem. The goal of this grant is to introduce general methods for analyzing this form of biostatistical data. We propose three major aims for the analysis of multilevel or longitudinally collected biosignals. The first extends multilevel functional principal components, the investigators' generalization of functional principal components, to longitudinal and high dimensional settings. The second considers the investigators bi-directional filtering and extends it in high-dimensional and longitudinal settings. The third considers model-based independent component blind source separation and extends it to longitudinal settings. To solve this aim, we will also consider the fundamental problem of running MCMC samplers for high dimensional parameter spaces. Specifically, no current work exists for convergence control when the number of parameters is larger than the number of iterations. We propose a method of convergence control using finite population sampling. Our methods will be applied to unique data sets involving imaging (MRI, fMRI, DTI), electrophysiology (EEG, ECOG), sleep measurement (polysomnography) and novel measurements of aging (accelerometer). In the preliminary results, we demonstrate our capacity for working with such data with novel findings in the analysis of EEG, MRI and fMRI data sets. Methods such as unsupervised clustering, blind source separation and dimension reduction are generally recognized first steps in analyzing high dimensional data, and have been applied success- fully in an amazingly diverse collection of settings. Our proposal generalizes these basic approaches to high dimensional data while considering hierarchical and longitudinal directions of variation. Hence, our approaches will form a basic foundation for next generation biomedical functional data.
PUBLIC HEALTH RELEVANCE: Modern observational data is often longitudinal or multilevel functional biological signals. We propose a foundational approach for the analysis of such data, including scalable computing to next generation data sets.
描述(由申请人提供):抽象的现代观察和实验生物学数据已经发生了革命。在新的生物技术和计算进步的驱动下,高维,高密度,功能多级和纵向生物学信号在医学和公共卫生研究中变得司空见惯。这些类型的信号历史上发生在小的临床或实验环境中,通常称为“小N,大P”问题。我们将这些生物信号的扩展为具有纵向或分层结构的队列研究是下一代生物统计问题。我们将其称为“分层大N,大P”问题。该赠款的目的是引入一般方法来分析这种形式的生物统计数据。 我们提出了三个主要目标,以分析多级或纵向收集的生物信号。第一个将多级功能主成分(研究者对功能主组件的概括)扩展到纵向和高维设置。第二个考虑了研究人员双向滤波,并在高维和纵向环境中扩展了它。第三个考虑了基于模型的独立组件盲源分离,并将其扩展到纵向设置。为了解决这个目标,我们还将考虑为高维参数空间运行MCMC采样器的基本问题。具体而言,当参数数量大于迭代次数时,没有目前的收敛控制工作。我们提出了一种使用有限种群抽样的收敛控制方法。 我们的方法将应用于涉及成像(MRI,fMRI,DTI),电生理学(EEG,ECOG),睡眠测量(多性测量)和衰老的新测量(加速度计)的独特数据集。在初步结果中,我们证明了在EEG,MRI和FMRI数据集分析中使用新发现的此类数据的能力。 通常在分析高维数据的方面公认了诸如无监督聚类,盲源分离和降低维度的方法,并已成功地应用于多样化的设置集合中。我们的建议将这些基本方法推广到高维数据的同时,同时考虑了变异的层次结构和纵向方向。因此,我们的方法将构成下一代生物医学功能数据的基本基础。
公共卫生相关性:现代观察数据通常是纵向或多级功能生物学信号。我们提出了一种基础方法来分析此类数据,包括可扩展计算到下一代数据集。
项目成果
期刊论文数量(0)
专著数量(0)
科研奖励数量(0)
会议论文数量(0)
专利数量(0)
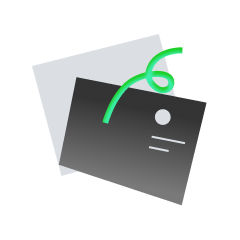
暂无数据
数据更新时间:2024-06-01
BRIAN Scott CAFFO的其他基金
Statistical methods for structural and functional integration in multi-modal neuroimaging data
多模态神经影像数据结构和功能整合的统计方法
- 批准号:1029672910296729
- 财政年份:2021
- 资助金额:$ 37.21万$ 37.21万
- 项目类别:
Statistical methods for structural and functional integration in multi-modal neuroimaging data
多模态神经影像数据结构和功能整合的统计方法
- 批准号:1044505310445053
- 财政年份:2021
- 资助金额:$ 37.21万$ 37.21万
- 项目类别:
Statistical methods for structural and functional integration in multi-modal neuroimaging data
多模态神经影像数据结构和功能整合的统计方法
- 批准号:1058615510586155
- 财政年份:2021
- 资助金额:$ 37.21万$ 37.21万
- 项目类别:
Big Data education for the masses: MOOCs, modules, & intelligent tutoring systems
面向大众的大数据教育:MOOC、模块、
- 批准号:88293708829370
- 财政年份:2014
- 资助金额:$ 37.21万$ 37.21万
- 项目类别:
Statistical methods for large n and p problems
大型 n 和 p 问题的统计方法
- 批准号:85131628513162
- 财政年份:2010
- 资助金额:$ 37.21万$ 37.21万
- 项目类别:
Statistical methods for large n and p problems
大型 n 和 p 问题的统计方法
- 批准号:81461078146107
- 财政年份:2010
- 资助金额:$ 37.21万$ 37.21万
- 项目类别:
Statistical methods for large n and p problems
大型 n 和 p 问题的统计方法
- 批准号:87280088728008
- 财政年份:2010
- 资助金额:$ 37.21万$ 37.21万
- 项目类别:
Statistical methods for large n and p problems
大型 n 和 p 问题的统计方法
- 批准号:83210378321037
- 财政年份:2010
- 资助金额:$ 37.21万$ 37.21万
- 项目类别:
Statistical methods for large n and p problems
大型 n 和 p 问题的统计方法
- 批准号:91341389134138
- 财政年份:2010
- 资助金额:$ 37.21万$ 37.21万
- 项目类别:
A mentored training program in quantitative medical imaging
定量医学成像指导培训计划
- 批准号:72262937226293
- 财政年份:2006
- 资助金额:$ 37.21万$ 37.21万
- 项目类别:
相似海外基金
Uncovering Mechanisms of Racial Inequalities in ADRD: Psychosocial Risk and Resilience Factors for White Matter Integrity
揭示 ADRD 中种族不平等的机制:心理社会风险和白质完整性的弹性因素
- 批准号:1067635810676358
- 财政年份:2024
- 资助金额:$ 37.21万$ 37.21万
- 项目类别:
The Influence of Lifetime Occupational Experience on Cognitive Trajectories Among Mexican Older Adults
终生职业经历对墨西哥老年人认知轨迹的影响
- 批准号:1074860610748606
- 财政年份:2024
- 资助金额:$ 37.21万$ 37.21万
- 项目类别:
The Proactive and Reactive Neuromechanics of Instability in Aging and Dementia with Lewy Bodies
衰老和路易体痴呆中不稳定的主动和反应神经力学
- 批准号:1074953910749539
- 财政年份:2024
- 资助金额:$ 37.21万$ 37.21万
- 项目类别:
Individual Predoctoral Fellowship
个人博士前奖学金
- 批准号:1075203610752036
- 财政年份:2024
- 资助金额:$ 37.21万$ 37.21万
- 项目类别:
Fluency from Flesh to Filament: Collation, Representation, and Analysis of Multi-Scale Neuroimaging data to Characterize and Diagnose Alzheimer's Disease
从肉体到细丝的流畅性:多尺度神经影像数据的整理、表示和分析,以表征和诊断阿尔茨海默病
- 批准号:1046225710462257
- 财政年份:2023
- 资助金额:$ 37.21万$ 37.21万
- 项目类别: