Deep Learning Models for Metabolomics Analysis
用于代谢组学分析的深度学习模型
基本信息
- 批准号:10552395
- 负责人:
- 金额:$ 21.67万
- 依托单位:
- 依托单位国家:美国
- 项目类别:
- 财政年份:2023
- 资助国家:美国
- 起止时间:2023-04-01 至 2028-03-31
- 项目状态:未结题
- 来源:
- 关键词:
项目摘要
PROJECT SUMMARY
Untargeted metabolomics using tandem mass spectrometry (MS) have attained substantial success in the
discovery of biomarkers and advancing our understanding of cellular metabolism. Despite this success, only a
small fraction of measured spectra can currently be annotated (assigned a chemical identity). This bottleneck
can be attributed to the limitations of current annotation tools that have not yet exploited advances in deep
learning and available data modalities (spectra, peaks, molecules, and fragments). The goal of this application
is to advance the interpretation of spectra collected through untargeted metabolomics. We focus on annotating
data collected through liquid or gas chromatology followed by MS, or MS/MS, as these three tandem
technologies have become dominant technologies. Over the next five years, the plan is to harness deep learning
to address three problems: 1) annotation, 2) translation between spectra measured under different instrument
settings, and 3) explainable models for annotation, where explainability arises from connecting peaks to their
respective molecular fragments.
The Hassoun lab has extensive, relevant deep learning experience to effectively tackle these problems.
The Lab also has experience in dealing with the nuances of metabolomics datasets. The Lab recently developed
a novel deep learning annotation model that achieves 41% and 30% performance improvement over multi-layer
neural networks and graph neural networks, respectively. Additionally, our lab has developed an ontology-
traversal algorithm that yields correct-by-construction molecular substructures that can be assigned to peaks,
thus giving rise to datasets that can be used to train explainable annotation models.
The Significance of this research is that it addresses fundamental barriers that hinder developing deep
learning annotation models. Our models and datasets will be released on GitHub to benefit biological and
biomedical applications and metabolomics research. Because of their expected high accuracy and explainability,
the models will expedite the interpretation of experiments, improve our understanding of cellular metabolism,
and facilitate data sharing among labs. The innovation lies in maximally learn from data modalities and in
creating models that exploit the learned representations. Further, the annotation and translation problems are
formulated as a bidirectional mapping between domains, in contrast to current annotation models that assume
unimodal mappings. These innovations are necessary to advance metabolomics research and they will open
new research horizons in the field of metabolomics.
项目摘要
使用串联质谱法(MS)的非靶向代谢组学在该领域取得了巨大成功
发现生物标志物并推进我们对细胞代谢的理解。尽管取得了成功,但只有一个
目前可以注释测得的光谱的一小部分(分配了化学身份)。这个瓶颈
可以归因于尚未利用深层进步的当前注释工具的局限性
学习和可用的数据模式(光谱,峰,分子和片段)。该应用程序的目标
是为了推进通过非靶向代谢组学收集的光谱的解释。我们专注于注释
通过这三个串联,通过液体或气体色谱收集的数据,然后是MS/MS
技术已成为主导技术。在接下来的五年中,计划是利用深度学习
解决三个问题:1)注释,2)在不同仪器下测量光谱之间的翻译
设置和3)可解释的注释模型,其中解释性是由连接峰与它们的
各自的分子碎片。
Hassoun Lab具有丰富的,相关的深度学习经验,可以有效解决这些问题。
该实验室还具有处理代谢组学数据集的细微差别的经验。实验室最近开发了
一种新颖的深度学习注释模型,可在多层方面取得41%和30%的性能提高
神经网络和图形神经网络。此外,我们的实验室已经开发了一个本体学 -
遍历算法可得出可以分配到峰的分子下结构正确的遍历算法
因此产生可用于训练可解释的注释模型的数据集。
这项研究的意义在于它解决了阻碍深层发展的基本障碍
学习注释模型。我们的模型和数据集将在GitHub上发布,以使生物学和
生物医学应用和代谢组学研究。由于他们的预期高精度和解释性,
这些模型将加快实验的解释,提高我们对细胞代谢的理解,
并促进实验室之间的数据共享。创新在于最大程度地学习数据方式和
创建利用学习表示的模型。此外,注释和翻译问题是
与当前的注释模型相反,该模型是在域之间的双向映射
单峰映射。这些创新对于推进代谢组学研究是必要的,它们将开放
代谢组学领域的新研究视野。
项目成果
期刊论文数量(0)
专著数量(0)
科研奖励数量(0)
会议论文数量(0)
专利数量(0)
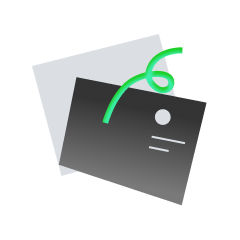
暂无数据
数据更新时间:2024-06-01
Soha Hassoun的其他基金
Using Common Fund Datasets to Illuminate Drug-Microbial Interactions
使用共同基金数据集阐明药物-微生物相互作用
- 批准号:1077733910777339
- 财政年份:2023
- 资助金额:$ 21.67万$ 21.67万
- 项目类别:
Computational Techniques for Advancing Untargeted Metabolomics Analysis
推进非靶向代谢组学分析的计算技术
- 批准号:1002212510022125
- 财政年份:2019
- 资助金额:$ 21.67万$ 21.67万
- 项目类别:
Computational Techniques for Advancing Untargeted Metabolomics Analysis
推进非靶向代谢组学分析的计算技术
- 批准号:1039401210394012
- 财政年份:2019
- 资助金额:$ 21.67万$ 21.67万
- 项目类别:
Computational Techniques for Advancing Untargeted Metabolomics Analysis
推进非靶向代谢组学分析的计算技术
- 批准号:1024207510242075
- 财政年份:2019
- 资助金额:$ 21.67万$ 21.67万
- 项目类别:
Computational Techniques for Advancing Untargeted Metabolomics Analysis
推进非靶向代谢组学分析的计算技术
- 批准号:1048081810480818
- 财政年份:2019
- 资助金额:$ 21.67万$ 21.67万
- 项目类别:
相似国自然基金
基于机器学习算法的针刺干预偏头痛预后差异生物学机制和临床-多组学预测模型构建研究
- 批准号:82374572
- 批准年份:2023
- 资助金额:48 万元
- 项目类别:面上项目
微生物生态系统共性规律探索及其对扰动响应的预测算法研究
- 批准号:32300078
- 批准年份:2023
- 资助金额:30 万元
- 项目类别:青年科学基金项目
基于耦合矩阵-张量分解算法开发环境微生物标志物挖掘工具
- 批准号:42377106
- 批准年份:2023
- 资助金额:49 万元
- 项目类别:面上项目
基于生成式人工智能的易合成与高生物活性的分子三维结构设计
- 批准号:22373085
- 批准年份:2023
- 资助金额:50.00 万元
- 项目类别:面上项目
面向可信虹膜识别的脉冲表达学习
- 批准号:62306307
- 批准年份:2023
- 资助金额:30.00 万元
- 项目类别:青年科学基金项目
相似海外基金
Fluency from Flesh to Filament: Collation, Representation, and Analysis of Multi-Scale Neuroimaging data to Characterize and Diagnose Alzheimer's Disease
从肉体到细丝的流畅性:多尺度神经影像数据的整理、表示和分析,以表征和诊断阿尔茨海默病
- 批准号:1046225710462257
- 财政年份:2023
- 资助金额:$ 21.67万$ 21.67万
- 项目类别:
New Algorithms for Cryogenic Electron Microscopy
低温电子显微镜的新算法
- 批准号:1054356910543569
- 财政年份:2023
- 资助金额:$ 21.67万$ 21.67万
- 项目类别:
Discovery-Driven Mathematics and Artificial Intelligence for Biosciences and Drug Discovery
用于生物科学和药物发现的发现驱动数学和人工智能
- 批准号:1055157610551576
- 财政年份:2023
- 资助金额:$ 21.67万$ 21.67万
- 项目类别:
Biomarkers for Brain Resetting as an Assistive Tool in the Treatment of Status Epilepticus
大脑重置生物标志物作为治疗癫痫持续状态的辅助工具
- 批准号:1069896910698969
- 财政年份:2023
- 资助金额:$ 21.67万$ 21.67万
- 项目类别:
A computational model for prediction of morphology, patterning, and strength in bone regeneration
用于预测骨再生形态、图案和强度的计算模型
- 批准号:1072794010727940
- 财政年份:2023
- 资助金额:$ 21.67万$ 21.67万
- 项目类别: