Improved characterization of failure behaviours of sheet metals based on pattern recognition methods
基于模式识别方法改进金属板材失效行为表征
基本信息
- 批准号:325262702
- 负责人:
- 金额:--
- 依托单位:
- 依托单位国家:德国
- 项目类别:Research Grants
- 财政年份:2017
- 资助国家:德国
- 起止时间:2016-12-31 至 2022-12-31
- 项目状态:已结题
- 来源:
- 关键词:
项目摘要
The aim of the research project is an objective classification of failure behaviour of sheet metals during forming processes with the aid of pattern recognition methods. In order to extend the FLC with failure stages, the video sequences of the forming processes were annotated by experts and the individual images of the sequences were assigned to the following different failure classes: homogeneous forming, diffuse necking, localised necking and crack initiation. Based on the expert annotations, a supervised pattern recognition method was developed that provides automatic classification into the respective failure stages. In particular, a sensitivity of up to 92% was achieved for the local necking class, whereas the consistency of the expert annotations and artifacts in the strain distributions negatively impaired the quality of the results. In order to achieve expert independence and transferability to new materials, an unsupervised pattern recognition method was additionally developed, which as a result provides an FLC with different failure probabilities and achieves higher forming limits compared to conventional evaluation procedures with the same safety margin. These results were additionally validated by metallographic investigations. In the new project phase an extension of the methodology to additional material characterization tests will be investigated. The challenges are not only the development of the machine learning approach to potentially more robust deep learning methods, but also the comprehensive analysis of material mechanisms under different strain conditions. The transferability of the evaluation method is evaluated by means of uniaxial tensile, notch tensile and hydraulic bulge tests and the application spectrum is additionally extended to the bending test. The limit strains of the experiments, determined by machine learning, are validated with metallographic investigations and material-specific behavior patterns are additionally identified in the individual experiments. Furthermore, in the first phase it was ascertained that discontinuity in the strain distribution, such as local maxima, can be detected automatically. However, the determination of an objective failure definition is complex and an objective failure definition cannot yet be conclusively established. On the one hand, the metallographic results can only be transferred to pattern recognition to a limited extent, since the observed discontinuities are in the micrometer range and are therefore only partially captured by the strain measurement. On the other hand, the discontinuities are strain localizations that may not coincide with the material failure. Hereby, the effects of the discontinuity on the methodology are investigated. In order to quantify the material properties, a stochastic evaluation based on more than 100 tensile tests is carried out to determine which material properties, forming stages and patterns can be detected during material aging.
研究项目的目的是借助模式识别方法对纸金属的故障行为进行客观分类。为了在失败阶段扩展FLC,专家注释了形成过程的视频序列,并将序列的各个图像分配给以下不同的故障类别:均匀形成,弥漫性颈部,局部颈部和裂纹启动。根据专家注释,开发了一种监督模式识别方法,该方法将自动分类为各自的故障阶段。特别是,对于本地颈部类别,敏感性高达92%,而专家注释和菌株分布中的人工制品的一致性对结果的质量负面损害。为了实现专家的独立性和向新材料的转移性,另外开发了一种无监督的模式识别方法,因此,与具有相同安全保证金的常规评估程序相比,它提供了具有不同故障概率的FLC,并获得了更高的形成限制。这些结果还通过金属学调查来验证。在新的项目阶段,将研究该方法将方法扩展到其他材料表征测试。挑战不仅是机器学习方法的开发,以实现更强大的深度学习方法,而且是对不同应变条件下材料机制的全面分析。评估方法的可传递性是通过单轴拉伸,缺口拉伸和液压凸起测试评估的,并且应用频谱还扩展到弯曲测试。 通过机器学习确定的实验的极限菌株通过金相研究验证,并且在单个实验中还可以鉴定出特定于材料的行为模式。此外,在第一阶段,可以自动检测到应变分布(例如局部最大值)的不连续性。但是,确定客观故障定义是复杂的,目的失败定义尚未得到最终确定。一方面,由于观察到的不连续性在千分尺范围内,因此金属图结果只能在有限的程度上传递到模式识别,因此仅通过应变测量部分捕获。另一方面,不连续性是可能与物质故障一致的应变局部定位。因此,研究了不连续性对方法的影响。为了量化材料特性,进行了基于100多次拉伸测试的随机评估,以确定在材料衰老期间可以检测到哪些材料特性,形成阶段和模式。
项目成果
期刊论文数量(0)
专著数量(0)
科研奖励数量(0)
会议论文数量(0)
专利数量(0)
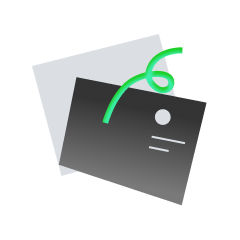
暂无数据
数据更新时间:2024-06-01
Professor Dr.-Ing....的其他基金
Joint Iterative Reconstruction and Motion Compensation for Optical Coherence Tomography Angiography
光学相干断层扫描血管造影的联合迭代重建和运动补偿
- 批准号:414781207414781207
- 财政年份:2019
- 资助金额:----
- 项目类别:Research GrantsResearch Grants
Consistency Conditions for Artifact Reduction in Cone-beam CT
锥束CT伪影减少的一致性条件
- 批准号:273134754273134754
- 财政年份:2015
- 资助金额:----
- 项目类别:Research GrantsResearch Grants
Temporally resolved 3-D retinal blood flow quantification using advanced motion correction and signal reconstruction in optical coherence tomography angiography
在光学相干断层扫描血管造影中使用先进的运动校正和信号重建进行时间分辨 3D 视网膜血流定量
- 批准号:508075009508075009
- 财政年份:
- 资助金额:----
- 项目类别:Research GrantsResearch Grants
相似国自然基金
高应力及颗粒破碎对水合物沉积物力学特性影响的宏细观研究与本构表征
- 批准号:52308358
- 批准年份:2023
- 资助金额:10 万元
- 项目类别:青年科学基金项目
钙钛矿发光二极管界面电压分布的表征和调控研究
- 批准号:22372193
- 批准年份:2023
- 资助金额:50 万元
- 项目类别:面上项目
基于城市形态双重表征互动学习的人工智能辅助城市设计技术
- 批准号:52308068
- 批准年份:2023
- 资助金额:30 万元
- 项目类别:青年科学基金项目
单属种濒危植物蒜头果Ⅱ型MADS-box基因的全基因组表征及在果实发育过程中的功能解析
- 批准号:32360090
- 批准年份:2023
- 资助金额:32 万元
- 项目类别:地区科学基金项目
新型乳酸菌胞外多糖的结构表征及其对小鼠肠道屏障的作用机制
- 批准号:32360570
- 批准年份:2023
- 资助金额:32 万元
- 项目类别:地区科学基金项目
相似海外基金
Parallel Characterization of Genetic Variants in Chemotherapy-Induced Cardiotoxicity Using iPSCs
使用 iPSC 并行表征化疗引起的心脏毒性中的遗传变异
- 批准号:1066361310663613
- 财政年份:2023
- 资助金额:----
- 项目类别:
Microenvironmental characterization and manipulation to prevent secondary caries
预防继发龋的微环境特征和操作
- 批准号:1081403010814030
- 财政年份:2023
- 资助金额:----
- 项目类别:
Characterization of the Regulation and Gene Targets of TBX2 in Rhabdomyosarcoma
横纹肌肉瘤中 TBX2 的调控和基因靶点的表征
- 批准号:1073102510731025
- 财政年份:2023
- 资助金额:----
- 项目类别:
Genome-wide characterization of complex variants and their phenotypic effects in African populations
复杂变异的全基因组特征及其在非洲人群中的表型效应
- 批准号:1072181110721811
- 财政年份:2023
- 资助金额:----
- 项目类别:
Systematic Characterization and Targeting of Neomorphic Drivers in Cancer
癌症新形态驱动因素的系统表征和靶向
- 批准号:1071797310717973
- 财政年份:2023
- 资助金额:----
- 项目类别: