CAREER: Towards Fundamentals of Adaptive, Collaborative and Intelligent Radar Sensing and Perception
职业:探索自适应、协作和智能雷达传感和感知的基础知识
基本信息
- 批准号:2340029
- 负责人:
- 金额:$ 50.68万
- 依托单位:
- 依托单位国家:美国
- 项目类别:Continuing Grant
- 财政年份:2024
- 资助国家:美国
- 起止时间:2024-08-15 至 2029-07-31
- 项目状态:未结题
- 来源:
- 关键词:
项目摘要
Automotive radar imaging represents a pressing technological need in perception for automotive active safety and autonomous driving. Automotive radar operating at millimeter-wave frequencies (typically around 77 GHz) is indispensable due to its superior capability in measuring range, velocity, and offering better perception performance in occlusion situations under all weather conditions and much lower cost than Lidar. The state-of-the-art automotive radar is prone to mutual interference and multipath issues, and its limited angular resolution—approximately 13 degrees—is inadequate for facilitating perception tasks for fully autonomous driving. This CAREER project aims to innovate the automotive radar perception in service of well-being of individuals in society and reduction of fatal accidents on U.S. highways by exploring adaptive, collaborative sensing and radar imaging physics principles. It deepens our understanding of how such sensing can enhance the dynamic range and address the ill-posed nature of the radar imaging inverse problem. Addressing the challenges in automotive radar imaging necessitates collaboration between academia and industry. Through collaboration with automotive industry sector, this project will make the research more impactful and pertinent, hastening the transition of research findings into automotive industry applications. Autonomous vehicle research helps attract underrepresented researchers. Through K-12 outreach activities and recruiting underrepresented groups and women in engineering, this project will promote diversity in the STEM research, inspiring them to pursue advanced research in radar field. This CAREER project addresses the ill-posed inverse problem inherent in automotive radar imaging by investigation of learning based adaptive and collaborative methodologies. The project tackles the robust radar perception for autonomous vehicles problem through incorporation of the radar imaging physics to drive the design of innovative machine learning algorithms. Intelligent signal processing and machine learning techniques will be developed at multiple layers, including 1) learning-based adaptive radar transmit parameter adjustment, 2) iterative optimization algorithms to enhance the dynamic range of automotive radar sensing by exploiting constructive interference, 3) model-based learning framework for collaborative high-resolution radar imaging in an automotive radar network, 4) physics-aware radar machine learning algorithms for robust environment perception. These new techniques will demonstrate how the science insights advance high resolution radar imaging, and robustly detect and classify objects in the highly dynamic autonomous driving environment.This award reflects NSF's statutory mission and has been deemed worthy of support through evaluation using the Foundation's intellectual merit and broader impacts review criteria.
汽车雷达成像代表了对汽车主动安全性和自动驾驶的迫切技术需求。在毫米波频率下运行的汽车雷达(通常约为77 GHz)是必不可少的,这是由于其在所有天气条件下的遮挡情况下的测量范围,速度,速度的卓越能力,在遮挡情况下提供更好的感知性能,并且比LIDAR低得多。最先进的汽车雷达容易出现相互干扰和多径问题,并且其有限的角度分辨率(约13度)不足以支持完全自主驾驶的感知任务。该职业项目旨在通过探索适应性,协作敏感性和雷达成像物理学原理来创新汽车雷达感知,以服务社会中的个人福祉和减少美国高速公路的致命事故。它加深了我们对这种敏感性如何增强动态范围并解决雷达成像逆问题的性质的理解。解决了学术界与行业之间必要的合作中汽车雷达成像中所面临的挑战。通过与汽车行业的合作,该项目将使研究更具影响力和相关性,从而加快研究结果向汽车行业应用的过渡。自动驾驶汽车研究有助于吸引代表性不足的研究人员。通过K-12的宣传活动和招募人数不足的群体和工程女性,该项目将促进STEM研究的多样性,激发他们在雷达领域进行先进研究。该职业项目通过研究基于学习的自适应和协作方法来解决汽车雷达成像中固有的逆问题。该项目通过雷达成像物理学的工业系统来解决自动驾驶汽车问题的强大雷达感知,以推动创新的机器学习算法的设计。智能信号处理和机器学习技术将在多层开发,包括1)基于学习的自适应雷达传输参数调整,2)迭代优化算法,以增强基于建设性干扰的自动雷达敏感性的动态范围,3)基于基于Mangial Imental Imental Imental Imental Imental-Network radar radar radar radar radar radar,4鲁棒环境感知的算法。这些新技术将展示科学见解如何提高高分辨率雷达成像,并在高度动态的自主驾驶环境中稳健地检测和分类对象。该奖项反映了NSF的法定任务,并被认为是通过基金会的知识分子优点和更广泛影响的审查标准通过评估来通过评估来获得的支持。
项目成果
期刊论文数量(0)
专著数量(0)
科研奖励数量(0)
会议论文数量(0)
专利数量(0)
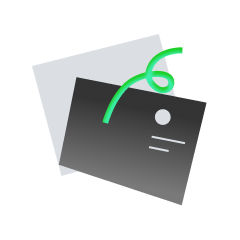
暂无数据
数据更新时间:2024-06-01
Shunqiao Sun其他文献
Redefining Automotive Radar Imaging: A Domain-Informed 1D Deep Learning Approach for High-Resolution and Efficient Performance
重新定义汽车雷达成像:一种基于领域的一维深度学习方法,可实现高分辨率和高效性能
- DOI:
- 发表时间:20242024
- 期刊:
- 影响因子:0
- 作者:Ru;Shunqiao Sun;Holger Caesar;Honglei Chen;Jian LiRu;Shunqiao Sun;Holger Caesar;Honglei Chen;Jian Li
- 通讯作者:Jian LiJian Li
Interpretable and Efficient Beamforming-Based Deep Learning for Single Snapshot DOA Estimation
用于单快照 DOA 估计的可解释且高效的基于波束成形的深度学习
- DOI:
- 发表时间:20232023
- 期刊:
- 影响因子:4.3
- 作者:Ru;Shunqiao Sun;Hongshan Liu;Honglei Chen;Jian LiRu;Shunqiao Sun;Hongshan Liu;Honglei Chen;Jian Li
- 通讯作者:Jian LiJian Li
Investigation of microwave transducers for linearity dependence and applications in quantum networking
微波换能器线性依赖性的研究及其在量子网络中的应用
- DOI:10.1117/12.263352210.1117/12.2633522
- 发表时间:20222022
- 期刊:
- 影响因子:0
- 作者:Summer Bolton;Joseph T. Lukens;Carson E. Moseley;Maddy Woodson;S. Estrella;Shunqiao Sun;S. Kim;P. KungSummer Bolton;Joseph T. Lukens;Carson E. Moseley;Maddy Woodson;S. Estrella;Shunqiao Sun;S. Kim;P. Kung
- 通讯作者:P. KungP. Kung
Ieee Transactions on Aerospace and Electronic Systems 1 Mimo-mc Radar: a Mimo Radar Approach Based on Matrix Completion
- DOI:
- 发表时间:
- 期刊:
- 影响因子:0
- 作者:Shunqiao SunShunqiao Sun
- 通讯作者:Shunqiao SunShunqiao Sun
Interference Mitigation in Automotive Radars
- DOI:
- 发表时间:20152015
- 期刊:
- 影响因子:0
- 作者:Shunqiao SunShunqiao Sun
- 通讯作者:Shunqiao SunShunqiao Sun
共 10 条
- 1
- 2
Shunqiao Sun的其他基金
CRII: CIF: A Sparse Framework Based Automotive Radar Sensing for Autonomous Vehicles
CRII:CIF:基于稀疏框架的自动驾驶汽车雷达传感
- 批准号:21533862153386
- 财政年份:2022
- 资助金额:$ 50.68万$ 50.68万
- 项目类别:Standard GrantStandard Grant
相似国自然基金
SHP2调控Treg向Th2-like Treg的可塑性转化在变应性鼻炎中的作用与机制研究
- 批准号:82301281
- 批准年份:2023
- 资助金额:30 万元
- 项目类别:青年科学基金项目
EAST高极向比压运行模式下芯部与边界兼容机制的数值模拟研究
- 批准号:12375228
- 批准年份:2023
- 资助金额:53 万元
- 项目类别:面上项目
CXCR5依赖的边缘区B细胞向滤泡树突状细胞呈递外泌体引发心脏移植排斥的研究
- 批准号:82300460
- 批准年份:2023
- 资助金额:30 万元
- 项目类别:青年科学基金项目
Dlx2通过调控Tspan13影响上颌突间充质干细胞骨向分化的机制研究
- 批准号:82301008
- 批准年份:2023
- 资助金额:30 万元
- 项目类别:青年科学基金项目
糖尿病心肌病心室重构的新机制:高糖诱导巨噬细胞脂质代谢重编程通过活性脂质MA调控心脏成纤维细胞向肌成纤维细胞转分化的机制研究
- 批准号:82300404
- 批准年份:2023
- 资助金额:30 万元
- 项目类别:青年科学基金项目
相似海外基金
Collaborative Research: NeTS: JUNO3: Towards an Internet of Federated Digital Twins (IoFDT) for Society 5.0: Fundamentals and Experimentation
合作研究:NetS:JUNO3:迈向社会 5.0 的联合数字孪生 (IoFDT) 互联网:基础知识和实验
- 批准号:22102542210254
- 财政年份:2022
- 资助金额:$ 50.68万$ 50.68万
- 项目类别:Standard GrantStandard Grant
Collaborative Research: NeTS: JUNO3: Towards an Internet of Federated Digital Twins (IoFDT) for Society 5.0: Fundamentals and Experimentation
合作研究:NetS:JUNO3:迈向社会 5.0 的联合数字孪生 (IoFDT) 互联网:基础知识和实验
- 批准号:22102552210255
- 财政年份:2022
- 资助金额:$ 50.68万$ 50.68万
- 项目类别:Continuing GrantContinuing Grant
FNR - Fundamentals of Negative Capacitance: Towards New Low Power Electronics
FNR - 负电容基础知识:迈向新型低功耗电子产品
- 批准号:EP/S010769/1EP/S010769/1
- 财政年份:2019
- 资助金额:$ 50.68万$ 50.68万
- 项目类别:Research GrantResearch Grant
向き付け不可能曲面の写像類群における組合せ論的群論の観点からの研究
组合群论视角下不可定向曲面映射类群研究
- 批准号:19K1454219K14542
- 财政年份:2019
- 资助金额:$ 50.68万$ 50.68万
- 项目类别:Grant-in-Aid for Early-Career ScientistsGrant-in-Aid for Early-Career Scientists
Various studies of homogeneous open convex cones and homogeneous Siegel domains
齐次开放凸锥和齐次西格尔域的各种研究
- 批准号:15K0490115K04901
- 财政年份:2015
- 资助金额:$ 50.68万$ 50.68万
- 项目类别:Grant-in-Aid for Scientific Research (C)Grant-in-Aid for Scientific Research (C)