CAREER: Optimal Transport Beyond Probability Measures for Robust Geometric Representation Learning
职业生涯:超越概率测量的最佳传输以实现稳健的几何表示学习
基本信息
- 批准号:2339898
- 负责人:
- 金额:$ 55.36万
- 依托单位:
- 依托单位国家:美国
- 项目类别:Continuing Grant
- 财政年份:2024
- 资助国家:美国
- 起止时间:2024-02-01 至 2029-01-31
- 项目状态:未结题
- 来源:
- 关键词:
项目摘要
Machine learning is a pivotal technological pillar in modern human society, enabling the development of algorithms that automatically uncover valuable data patterns. Its profound influence extends across various industrial sectors, encompassing healthcare, automotive, energy, and entertainment. Furthermore, it has become an indispensable tool in scientific research, empowering scientists to analyze data, construct and validate hypotheses, and make predictions, thereby expediting the progress of scientific exploration and discovery. Despite its success, many foundational questions and theoretical aspects of machine learning remain poorly understood, posing unwanted ramifications associated with such technologies. Critical among these issues is determining how to accurately quantify the uncertainty of machine learning models and discerning when their predictions are trustworthy. Equally important is enhancing the efficiency and robustness of these methods, especially when they are required to learn patterns from limited data or demonstrations. To address some of these issues, the investigator will study the mathematical foundations of machine learning, using tools from optimal transport, integral geometry, and measure theory. The foundational tools developed in this project are anticipated to lead to the next generation of machine learning methods, notable for their efficiency, uncertainty awareness, interpretability, and robustness, with potential benefits in healthcare, transportation, and national defense. This research will be integrated with comprehensive education and outreach initiatives to encourage research involvement across academic levels, from high school to graduate studies. Particular emphasis will be placed on engaging disadvantaged groups, including minority and rural serving institutions in Middle Tennessee, and actively promoting diversity and inclusivity in STEM disciplines, particularly in artificial intelligence and machine learning education.This project is motivated by the fact that measuring meaningful distances between high-dimensional mathematical objects is central to modern machine learning. It aims to explore the impact of novel geometric distances on the efficiency and robustness of machine learning methods. The project's research agenda comprises three chronological phases: 1) developing scalable optimal transport-based metrics extending beyond probability measures, leveraging the investigator's prior work in (unbalanced) optimal transport, transport Lp, and generalized sliced distances in both flat and curved spaces, while considering their statistical, geometric, and topological properties; 2) creating Euclidean embeddings for the proposed metrics to facilitate their integration with traditional machine learning processes for efficient classification and clustering; and 3) combining transport-based embeddings with geometric deep representation learning models, and conducting high-dimensional studies to assess their impact on the performance and robustness of geometric deep learning methods. Phase 1 of the project develops computationally efficient distances for extended classes of measures, including both positive and signed vector measures, and explores their metric structure, topology, geodesics, and stability. Phase 2 concentrates on efficient embedding techniques for the transport-based metrics introduced in Phase 1, investigating their regularity, stability, and computational aspects. Phase 3 examines the invariance and equivariance of the transport-based embeddings developed in Phase 2 in relation to different symmetry groups and integrates these embeddings into geometric deep neural architectures. Lastly, the project aims to foster interdisciplinary collaboration by combining insights from integral geometry, measure theory, optimal transport, mathematical statistics, and machine learning, thereby encouraging knowledge exchange in these highly relevant fieldsThis award reflects NSF's statutory mission and has been deemed worthy of support through evaluation using the Foundation's intellectual merit and broader impacts review criteria.
机器学习是现代人类社会中的一个关键技术支柱,使能够自动发现有价值的数据模式的算法开发。它的深刻影响力跨越了各个工业领域,包括医疗保健,汽车,能源和娱乐。此外,它已成为科学研究中必不可少的工具,使科学家能够分析数据,构建和验证假设并做出预测,从而加快了科学探索和发现的进步。尽管取得了成功,但机器学习的许多基本问题和理论方面仍然鲜为人知,从而构成了与此类技术相关的不必要的后果。这些问题之间的关键是确定如何准确量化机器学习模型的不确定性,并在其预测值得信赖时辨别。同样重要的是提高这些方法的效率和鲁棒性,尤其是当它们被要求从有限的数据或演示中学习模式时。为了解决其中一些问题,研究者将使用最佳运输,积分几何形状和测量理论中的工具研究机器学习的数学基础。预计该项目中开发的基本工具将导致下一代机器学习方法,其效率,不确定性意识,可解释性和鲁棒性以及医疗保健,运输和国防的潜在好处。这项研究将与全面的教育和外展计划融合,以鼓励从高中到研究生学习的学术水平的研究参与。特别重点将放在田纳西州中部的少数群体和乡村服务机构,并积极促进茎学科的多样性和包容性,尤其是在人工智能和机器学习教育中,这是由衡量高位数学数学对象之间的有意义的距离,该事实是为了促进弱势群体的少数群体和农村服务机构,并会特别重视弱势群体。它旨在探索新型几何距离对机器学习方法效率和鲁棒性的影响。该项目的研究议程包括三个按时间顺序的阶段:1)开发可扩展的基于最佳运输的指标,扩展了概率措施,利用研究者的先前工作(不平衡)最佳运输,运输LP以及在平坦和弯曲空间中的广义切片距离,同时考虑其统计,几何学,几何学,几何学和拓扑特性; 2)为拟议的指标创建欧几里得嵌入,以促进其与传统的机器学习过程的集成,以有效地分类和聚类; 3)将基于运输的嵌入与几何深度表示模型相结合,并进行高维度研究以评估其对几何深度学习方法的性能和鲁棒性的影响。该项目的第1阶段为扩展的度量类别(包括正面和签名的矢量度量)开发了计算高效的距离,并探索了其度量结构,拓扑,地球学和稳定性。第2阶段集中于第1阶段引入的基于运输的指标的有效嵌入技术,研究其规律性,稳定性和计算方面。第3阶段研究了第2阶段与不同对称组相关的基于运输的嵌入的不变性和均衡,并将这些嵌入整合到几何深神经体系结构中。最后,该项目旨在通过结合整体几何学,测量理论,最佳运输,数学统计和机器学习的见解来促进跨学科的合作,从而鼓励在这些高度相关的领域中的知识交流,这反映了NSF的法规任务,并被认为是通过基金会的知识优点和广泛的criTia criter scriter criter criter criter criter criter criter criter criter criter criter criter criter criter criter criter criteria criter criter criteria criter criteria criter criteria criter criter criteria crietia均值得一提。
项目成果
期刊论文数量(0)
专著数量(0)
科研奖励数量(0)
会议论文数量(0)
专利数量(0)
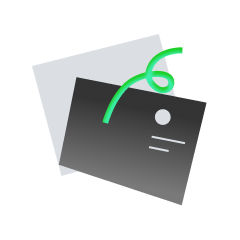
暂无数据
数据更新时间:2024-06-01
Soheil Kolouri其他文献
A symmetric deformation-based similarity measure for shape analysis
用于形状分析的基于对称变形的相似性度量
- DOI:10.1109/isbi.2015.716387610.1109/isbi.2015.7163876
- 发表时间:20152015
- 期刊:
- 影响因子:0
- 作者:Soheil Kolouri;D. Slepčev;G. RohdeSoheil Kolouri;D. Slepčev;G. Rohde
- 通讯作者:G. RohdeG. Rohde
Learning to Optimize Distributed Optimization: ADMM-based DC-OPF Case Study
学习优化分布式优化:基于 ADMM 的 DC-OPF 案例研究
- DOI:10.1109/pesgm52003.2023.1025343810.1109/pesgm52003.2023.10253438
- 发表时间:20232023
- 期刊:
- 影响因子:0
- 作者:Meiyi Li;Soheil Kolouri;Javad MohammadiMeiyi Li;Soheil Kolouri;Javad Mohammadi
- 通讯作者:Javad MohammadiJavad Mohammadi
MCNC: Manifold Constrained Network Compression
MCNC:流形约束网络压缩
- DOI:
- 发表时间:20242024
- 期刊:
- 影响因子:0
- 作者:Chayne Thrash;Ali Abbasi;Parsa Nooralinejad;Soroush Abbasi Koohpayegani;Reed Andreas;Hamed Pirsiavash;Soheil KolouriChayne Thrash;Ali Abbasi;Parsa Nooralinejad;Soroush Abbasi Koohpayegani;Reed Andreas;Hamed Pirsiavash;Soheil Kolouri
- 通讯作者:Soheil KolouriSoheil Kolouri
Is Multi-Task Learning an Upper Bound for Continual Learning?
多任务学习是持续学习的上限吗?
- DOI:
- 发表时间:20222022
- 期刊:
- 影响因子:0
- 作者:Zihao Wu;Huy Tran;H. Pirsiavash;Soheil KolouriZihao Wu;Huy Tran;H. Pirsiavash;Soheil Kolouri
- 通讯作者:Soheil KolouriSoheil Kolouri
Quantification of Respiratory Laryngeal Morphometry: Comparison of Laryngeal Lumen Angle Estimate Methods.
呼吸喉形态测量的量化:喉腔角度估计方法的比较。
- DOI:
- 发表时间:20162016
- 期刊:
- 影响因子:2.2
- 作者:Adrianna C. Shembel;Soheil Kolouri;Hongming Xu;Katherine Verdolini AbbottAdrianna C. Shembel;Soheil Kolouri;Hongming Xu;Katherine Verdolini Abbott
- 通讯作者:Katherine Verdolini AbbottKatherine Verdolini Abbott
共 10 条
- 1
- 2
相似国自然基金
基于情境最佳化的模型预测控制方法研究
- 批准号:62303416
- 批准年份:2023
- 资助金额:30 万元
- 项目类别:青年科学基金项目
基于因果学习的脓毒症最佳治疗策略与效应估计关键技术研究
- 批准号:62371438
- 批准年份:2023
- 资助金额:49.00 万元
- 项目类别:面上项目
两类区域上再生核希尔伯特空间中的n-最佳核逼近问题
- 批准号:12301101
- 批准年份:2023
- 资助金额:30 万元
- 项目类别:青年科学基金项目
Camassa-Holm方程和短脉冲型方程的最佳适定性与爆破现象研究
- 批准号:12301298
- 批准年份:2023
- 资助金额:30 万元
- 项目类别:青年科学基金项目
基于光域正交基分解的微波光子宽带矢量信号最佳接收方法研究
- 批准号:62305266
- 批准年份:2023
- 资助金额:30.00 万元
- 项目类别:青年科学基金项目
相似海外基金
Conference: Supplementary funding for the BIRS-CMO workshop Optimal Transport and Dynamics (24s5198)
会议:BIRS-CMO 研讨会最佳运输和动力学的补充资金 (24s5198)
- 批准号:24010192401019
- 财政年份:2024
- 资助金额:$ 55.36万$ 55.36万
- 项目类别:Standard GrantStandard Grant
Variational Optimal Transport Methods for Nonlinear Filtering
非线性滤波的变分最优传输方法
- 批准号:23189772318977
- 财政年份:2023
- 资助金额:$ 55.36万$ 55.36万
- 项目类别:Standard GrantStandard Grant
Collaborative Research: Parabolic Monge-Ampère Equations, Computational Optimal Transport, and Geometric Optics
合作研究:抛物线 Monge-AmpeÌre 方程、计算最优传输和几何光学
- 批准号:22466062246606
- 财政年份:2023
- 资助金额:$ 55.36万$ 55.36万
- 项目类别:Standard GrantStandard Grant
Online Inverse Optimal Transport for Societal Flows
社会流量的在线逆最优运输
- 批准号:EP/X010503/1EP/X010503/1
- 财政年份:2023
- 资助金额:$ 55.36万$ 55.36万
- 项目类别:Research GrantResearch Grant
Statistical Inference for Optimal Transport
最佳运输的统计推断
- 批准号:23106322310632
- 财政年份:2023
- 资助金额:$ 55.36万$ 55.36万
- 项目类别:Standard GrantStandard Grant