Collaborative Research: SCH: Trustworthy and Explainable AI for Neurodegenerative Diseases
合作研究:SCH:值得信赖且可解释的人工智能治疗神经退行性疾病
基本信息
- 批准号:2123952
- 负责人:
- 金额:$ 36万
- 依托单位:
- 依托单位国家:美国
- 项目类别:Standard Grant
- 财政年份:2021
- 资助国家:美国
- 起止时间:2021-10-01 至 2025-09-30
- 项目状态:未结题
- 来源:
- 关键词:
项目摘要
Driven by its performance accuracy, machine learning (ML) has been used extensively for various applications in the healthcare domain. Despite its promising performance, researchers and the public have grown alarmed by two unsettling deficiencies of these otherwise useful and powerful models. First, there is a lack of trustworthiness - ML models are prone to interference or deception and exhibit erratic behaviors when in action dealing with unseen data, despite good practice during the training phase. Second, there is a lack of interpretability - ML models have been described as 'black-boxes' because there is little explanation for why the models make the predictions they do. This has called into question the applicability of ML to decision-making in critical scenarios such as image-based disease diagnostics or medical treatment recommendation. The ultimate goal of this project is to develop computational foundation for trustworthy and explainable Artificial Intelligence (AI), and offer a low-cost and non-invasive ML-based approach to early diagnosis of neurodegenerative diseases. In particular, the project aims to develop computational theories, ML algorithms, and prototype systems. The project includes developing principled solutions to trustworthy ML and making the ML prediction process transparent to end-users. The later will focus on explaining how and why an ML model makes such a prediction, while dissecting its underlying structure for deeper understanding. The proposed models are further extended to a multi-modal and spatial-temporal framework, an important aspect of applying ML models to healthcare. A verification framework with end-users is defined, which will further enhance the trustworthiness of the prototype systems. This project will benefit a variety of high-impact AI-based applications in terms of their explainability, trustworthy, and verifiability. It not only advances the research fronts of deep learning and AI, but also supports transformations in diagnosing neurodegenerative diseases. This project will develop the computational foundation for trustworthy and explainable AI with several innovations. First, the project will systematically study the trustworthiness of ML systems. This will be measured by novel metrics such as, adversarial robustness and semantic saliency, and will be carried out to establish the theoretical basis and practical limits of trustworthiness of ML algorithms. Second, the project provides a paradigm shift for explainable AI, explaining how and why a ML model makes its prediction, moving away from ad-hoc explanations (i.e. what features are important to the prediction). A proof-based approach, which probes all the hidden layers of a given model to identify critical layers and neurons involved in a prediction from a local point of view, will be devised. Third, a verification framework, where users can verify the model's performance and explanations with proofs, will be designed to further enhance the trustworthiness of the system. Finally, the project also advances the frontier of neurodegenerative diseases early diagnosis from multimodal imaging and longitudinal data by: (i) identifying retinal vasculature biomarkers using proof-based probing in biomarker graph networks; (ii) connecting biomarkers of the retina and the brain vasculature via cross- modality explainable AI model; and, (iii) recognizing the longitudinal trajectory of vasculature biomarkers via a spatio-temporal recurrent explainable model. This synergistic effort between computer science and medicine will enable a wide range of applications to trustworthy and explainable AI for healthcare. The results of this project will be assimilated into the courses and summer programs that the research team have developed with specially designed projects to train students with trustworthy and explainable AI.This award reflects NSF's statutory mission and has been deemed worthy of support through evaluation using the Foundation's intellectual merit and broader impacts review criteria.
在其性能准确性的驱动下,机器学习(ML)已被广泛用于医疗领域的各种应用。尽管表现出色,但研究人员和公众对这些原本有用且强大的模型的两种令人不安的缺陷感到震惊。首先,缺乏可信赖性 - ML模型容易受到干扰或欺骗,尽管在训练阶段进行了良好的练习,但在处理看不见数据的行动时表现出不稳定的行为。其次,缺乏可解释性-ML模型被描述为“黑盒”,因为对于为什么模型做出预测的原因很少。这使ML在基于图像的疾病诊断或医疗建议等关键情况下对决策的适用性提出了质疑。该项目的最终目的是为可信赖和可解释的人工智能(AI)建立计算基础,并提供低成本和非侵入性ML的方法来早期诊断神经退行性疾病。特别是,该项目旨在开发计算理论,ML算法和原型系统。该项目包括开发可信赖的ML的原则解决方案,并使ML预测过程透明到最终用户。后来的将重点放在解释ML模型如何以及为何进行这样的预测,同时剖析其潜在结构以深入理解。提出的模型进一步扩展到了多模式和时空框架,这是将ML模型应用于医疗保健的重要方面。定义了具有最终用户的验证框架,这将进一步增强原型系统的可信度。该项目将在其解释性,可信赖和可验证性方面受益于各种高影响AI的应用程序。它不仅可以提高深度学习和AI的研究方面,而且还支持诊断神经退行性疾病的转变。该项目将为可信赖且可解释的AI提供一些创新的计算基础。首先,该项目将系统地研究ML系统的可信度。这将通过新颖的指标(例如对抗性鲁棒性和语义显着性)来衡量,并将进行以建立ML算法可信度的理论基础和实际限制。其次,该项目为可解释的AI提供了范式转变,并解释了ML模型如何以及为什么进行预测,而不是临时解释(即,哪些功能对预测很重要)。从当地的角度来探讨给定模型的所有隐藏层,从当地的角度识别参与预测的关键层和神经元的所有隐藏层。第三,用户可以在其中验证模型的性能和证明的解释,旨在进一步增强系统的可信度。最后,该项目还通过以下方式从多模式成像和纵向数据中提高了神经退行性疾病的前沿,并通过以下方式诊断出:(i)使用生物标志物图网络中的基于证明的探测器识别视网膜脉管体生物标志物; (ii)通过可解释的AI模型连接视网膜和脑脉管系统的生物标志物; (iii)通过时空复发模型识别脉管系统生物标志物的纵向轨迹。计算机科学和医学之间的协同工作将使广泛的应用程序可以在医疗保健中值得信赖和可解释的AI。该项目的结果将被吸收到研究团队通过专门设计的项目开发的课程和夏季课程中,以培训具有值得信赖和可解释的AI的学生。该奖项反映了NSF的法定任务,并被认为是值得通过基金会的知识分子优点和更广泛影响的审查标准来通过评估来获得支持的。
项目成果
期刊论文数量(0)
专著数量(0)
科研奖励数量(0)
会议论文数量(0)
专利数量(0)
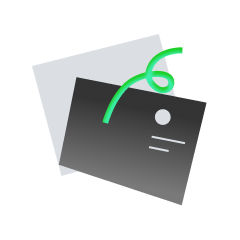
暂无数据
数据更新时间:2024-06-01
Eric Xing其他文献
What is Your Data Worth to GPT? LLM-Scale Data Valuation with Influence Functions
您的数据对 GPT 有何价值?
- DOI:
- 发表时间:20242024
- 期刊:
- 影响因子:0
- 作者:Sang Keun Choe;Hwijeen Ahn;Juhan Bae;Kewen Zhao;Minsoo Kang;Youngseog Chung;Adithya Pratapa;W. Neiswanger;Emma Strubell;Teruko Mitamura;Jeff Schneider;Eduard Hovy;Roger Grosse;Eric XingSang Keun Choe;Hwijeen Ahn;Juhan Bae;Kewen Zhao;Minsoo Kang;Youngseog Chung;Adithya Pratapa;W. Neiswanger;Emma Strubell;Teruko Mitamura;Jeff Schneider;Eduard Hovy;Roger Grosse;Eric Xing
- 通讯作者:Eric XingEric Xing
An exploratory study of self-supervised pre-training on partially supervised multi-label classification on chest X-ray images
胸部X射线图像部分监督多标签分类自监督预训练的探索性研究
- DOI:10.1016/j.asoc.2024.11185510.1016/j.asoc.2024.111855
- 发表时间:20242024
- 期刊:
- 影响因子:8.7
- 作者:Nanqing Dong;Michael Kampffmeyer;Haoyang Su;Eric XingNanqing Dong;Michael Kampffmeyer;Haoyang Su;Eric Xing
- 通讯作者:Eric XingEric Xing
共 2 条
- 1
Eric Xing的其他基金
III: Small: Multiple Device Collaborative Learning in Real Heterogeneous and Dynamic Environments
III:小:真实异构动态环境中的多设备协作学习
- 批准号:23119902311990
- 财政年份:2023
- 资助金额:$ 36万$ 36万
- 项目类别:Standard GrantStandard Grant
ML Basis for Intelligence Augmentation:Toward Personalized Modeling, Reasoning under Data-Knowledge Symbiosis, and Interpretable Interaction for AI-assisted Human Decision-making
智能增强的机器学习基础:面向人工智能辅助人类决策的个性化建模、数据知识共生下的推理和可解释的交互
- 批准号:20403812040381
- 财政年份:2021
- 资助金额:$ 36万$ 36万
- 项目类别:Continuing GrantContinuing Grant
CNS Core: Small: Toward Globally-Optimal Resource Distribution and Computation Acceleration in Multi-Tenant and Heterogeneous Machine Learning Systems
CNS 核心:小型:在多租户和异构机器学习系统中实现全局最优资源分配和计算加速
- 批准号:20082482008248
- 财政年份:2020
- 资助金额:$ 36万$ 36万
- 项目类别:Standard GrantStandard Grant
III: Small: A New Approach to Latent Space Learning with Diversity-Inducing Regularization and Applications to Healthcare Data Analytics
III:小型:具有多样性诱导正则化的潜在空间学习新方法及其在医疗保健数据分析中的应用
- 批准号:16175831617583
- 财政年份:2016
- 资助金额:$ 36万$ 36万
- 项目类别:Standard GrantStandard Grant
XPS: FULL: Broad-Purpose, Aggressively Asynchronous and Theoretically Sound Parallel Large-scale Machine Learning
XPS:FULL:用途广泛、积极异步且理论上合理的并行大规模机器学习
- 批准号:16295591629559
- 财政年份:2016
- 资助金额:$ 36万$ 36万
- 项目类别:Standard GrantStandard Grant
BIGDATA: F: DKA: Collaborative Research: Theory and Algorithms for Parallel Probabilistic Inference with Big Data, via Big Model, in Realistic Distributed Computing Environments
BIGDATA:F:DKA:协作研究:在现实分布式计算环境中通过大模型进行大数据并行概率推理的理论和算法
- 批准号:14476761447676
- 财政年份:2014
- 资助金额:$ 36万$ 36万
- 项目类别:Standard GrantStandard Grant
III: Small: Collaborative Research: Efficient, Nonparametric and Local-Minimum-Free Latent Variable Models: With Application to Large-Scale Computer Vision and Genomics
III:小型:协作研究:高效、非参数和局部最小自由潜变量模型:应用于大规模计算机视觉和基因组学
- 批准号:12182821218282
- 财政年份:2012
- 资助金额:$ 36万$ 36万
- 项目类别:Continuing GrantContinuing Grant
III: Small: Collaborative Research: Using Large-Scale Image Data for Online Social Media Analysis
III:小:协作研究:使用大规模图像数据进行在线社交媒体分析
- 批准号:11153131115313
- 财政年份:2011
- 资助金额:$ 36万$ 36万
- 项目类别:Standard GrantStandard Grant
Collaborative Research: Discovering and Exploiting Latent Communities in Social Media
协作研究:发现和利用社交媒体中的潜在社区
- 批准号:11111421111142
- 财政年份:2011
- 资助金额:$ 36万$ 36万
- 项目类别:Standard GrantStandard Grant
Indexing, Mining and Modeling Spatio-Temporal Patterns of Gene Expressions
基因表达时空模式的索引、挖掘和建模
- 批准号:06405430640543
- 财政年份:2007
- 资助金额:$ 36万$ 36万
- 项目类别:Continuing GrantContinuing Grant
相似国自然基金
支持二维毫米波波束扫描的微波/毫米波高集成度天线研究
- 批准号:62371263
- 批准年份:2023
- 资助金额:52 万元
- 项目类别:面上项目
腙的Heck/脱氮气重排串联反应研究
- 批准号:22301211
- 批准年份:2023
- 资助金额:30 万元
- 项目类别:青年科学基金项目
水系锌离子电池协同性能调控及枝晶抑制机理研究
- 批准号:52364038
- 批准年份:2023
- 资助金额:33 万元
- 项目类别:地区科学基金项目
基于人类血清素神经元报告系统研究TSPYL1突变对婴儿猝死综合征的致病作用及机制
- 批准号:82371176
- 批准年份:2023
- 资助金额:49 万元
- 项目类别:面上项目
FOXO3 m6A甲基化修饰诱导滋养细胞衰老效应在补肾法治疗自然流产中的机制研究
- 批准号:82305286
- 批准年份:2023
- 资助金额:30 万元
- 项目类别:青年科学基金项目
相似海外基金
Collaborative Research: SCH: Improving Older Adults' Mobility and Gait Ability in Real-World Ambulation with a Smart Robotic Ankle-Foot Orthosis
合作研究:SCH:使用智能机器人踝足矫形器提高老年人在现实世界中的活动能力和步态能力
- 批准号:23066602306660
- 财政年份:2023
- 资助金额:$ 36万$ 36万
- 项目类别:Standard GrantStandard Grant
Collaborative Research: SCH: A wireless optoelectronic implant for closed-loop control of bi-hormone secretion from genetically modified islet organoid grafts
合作研究:SCH:一种无线光电植入物,用于闭环控制转基因胰岛类器官移植物的双激素分泌
- 批准号:23067082306708
- 财政年份:2023
- 资助金额:$ 36万$ 36万
- 项目类别:Standard GrantStandard Grant
Collaborative Research: SCH: AI-driven RFID Sensing for Smart Health Applications
合作研究:SCH:面向智能健康应用的人工智能驱动的 RFID 传感
- 批准号:23067902306790
- 财政年份:2023
- 资助金额:$ 36万$ 36万
- 项目类别:Standard GrantStandard Grant
Collaborative Research: SCH: Improving Older Adults' Mobility and Gait Ability in Real-World Ambulation with a Smart Robotic Ankle-Foot Orthosis
合作研究:SCH:使用智能机器人踝足矫形器提高老年人在现实世界中的活动能力和步态能力
- 批准号:23066592306659
- 财政年份:2023
- 资助金额:$ 36万$ 36万
- 项目类别:Standard GrantStandard Grant
Collaborative Research: SCH: Therapeutic and Diagnostic System for Inflammatory Bowel Diseases: Integrating Data Science, Synthetic Biology, and Additive Manufacturing
合作研究:SCH:炎症性肠病的治疗和诊断系统:整合数据科学、合成生物学和增材制造
- 批准号:23067402306740
- 财政年份:2023
- 资助金额:$ 36万$ 36万
- 项目类别:Standard GrantStandard Grant