EAGER:Predictive Surrogate Modeling and Analysis of Radiative Heat transfer in Porous Media
EAGER:多孔介质中辐射传热的预测替代模型和分析
基本信息
- 批准号:1926882
- 负责人:
- 金额:$ 16.58万
- 依托单位:
- 依托单位国家:美国
- 项目类别:Standard Grant
- 财政年份:2019
- 资助国家:美国
- 起止时间:2019-06-01 至 2020-11-30
- 项目状态:已结题
- 来源:
- 关键词:
项目摘要
Radiative heat transfer in porous media is complex and ambiguous; yet, measuring the radiative properties of a target porous media is of vital importance. Measurement and prediction of the radiative properties is critical for simulation and design of energy technologies that involve porous material structures, including pebble beds in nuclear reactors, selective laser sintering technology, solar absorbers, solar thermochemical reactors, biological tissues, thermal barriers for jet engines and space vehicles, ceramic foams for catalytic combustion and many more. At present, predicting radiative properties of randomly packed beds requires large time-consuming ray-tracing simulations. This project replaces these computations with efficient machine learning based methods to revolutionize a wide range of related applications and underlying technologies. This transformative project demonstrates that surrogate models can approximate and predict the probability distribution functions of radiative properties of randomly packed structures reliably and efficiently. Large time-consuming ray-tracing Monte Carlo simulations are replaced by predictive models based on machine learning methods. The inputs to the models are statistics of a wide range of variables pertaining to the physical configurations of void, solid, boundary conditions and dimensions and the medium shape. Various learning models are studied for data fitting, and an analysis of accuracy versus the cost of computation is performed for each. Data sampling, model selection and model fitting are all engineered to render surrogate models that are accurate, efficient, scalable and generalizable. Sampling, design of experiment and model fitting is studied for each surrogate model to reduce the computational load while minimizing the cost of data collection and learning. The practical accuracy of the proposed models is validated based on comparison with direct Monte Carlo simulations and previously established laboratory-based experiments. The proposed predictive models are applied in computed tomography for inference of porous media structures in various applications.This award reflects NSF's statutory mission and has been deemed worthy of support through evaluation using the Foundation's intellectual merit and broader impacts review criteria.
多孔介质中的辐射传热是复杂而模棱两可的。但是,测量目标多孔介质的辐射特性至关重要。辐射特性的测量和预测对于涉及多孔材料结构的能源技术的模拟和设计至关重要,包括核反应器中的卵石床,选择性激光烧结技术,太阳能吸收器,太阳能热化学反应堆,生物组织,生物组织,喷气发动机和太空车辆的热屏障,陶器和陶器的cat仪和cat构燃料的多种多样。目前,预测随机包装床的辐射特性需要大量耗时的射线追踪模拟。该项目用基于机器学习的方法取代了这些计算,以彻底改变广泛的相关应用程序和基础技术。这个变革性的项目表明,替代模型可以可靠,有效地近似和预测随机堆积结构的辐射特性的概率分布功能。大型耗时的射线追踪蒙特卡洛模拟被基于机器学习方法的预测模型所取代。模型的输入是与空隙,实心,边界条件和尺寸和中等形状的物理配置有关的多种变量的统计数据。研究了各种学习模型以进行数据拟合,并对每个学习模型进行了分析与每个计算成本进行分析。数据采样,模型选择和模型拟合均经过设计,以渲染准确,高效,可扩展且可推广的替代模型。为每个替代模型研究了采样,实验设计和模型拟合的设计,以减少计算负载,同时最大程度地减少数据收集和学习成本。根据与直接蒙特卡洛模拟和先前建立的基于实验室的实验的比较,对所提出的模型的实际精度进行了验证。所提出的预测模型用于计算机断层扫描中用于推断各种应用中多孔媒体结构的推断。该奖项反映了NSF的法定任务,并且使用基金会的知识分子优点和更广泛的影响评估标准,认为NSF的法定任务值得通过评估。
项目成果
期刊论文数量(0)
专著数量(0)
科研奖励数量(0)
会议论文数量(0)
专利数量(0)
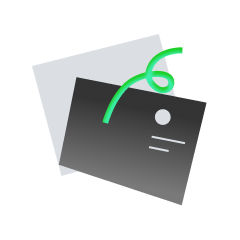
暂无数据
数据更新时间:2024-06-01
Shima Hajimirza其他文献
Using hybrid deep learning to predict spectral responses of quantum dot-embedded nanoporous thin-film solar cells
- DOI:10.1016/j.jqsrt.2024.10925810.1016/j.jqsrt.2024.109258
- 发表时间:2025-01-012025-01-01
- 期刊:
- 影响因子:
- 作者:Farhin Tabassum;George-Rafael Domenikos;Shima HajimirzaFarhin Tabassum;George-Rafael Domenikos;Shima Hajimirza
- 通讯作者:Shima HajimirzaShima Hajimirza
共 1 条
- 1
Shima Hajimirza的其他基金
CAREER: Precise Mathematical Modeling and Experimental Validation of Radiation Heat Transfer in Complex Porous Media Using Analytical Renewal Theory Abstraction-Regressions
职业:使用分析更新理论抽象回归对复杂多孔介质中的辐射传热进行精确的数学建模和实验验证
- 批准号:23390322339032
- 财政年份:2024
- 资助金额:$ 16.58万$ 16.58万
- 项目类别:Continuing GrantContinuing Grant
EAGER:Predictive Surrogate Modeling and Analysis of Radiative Heat transfer in Porous Media
EAGER:多孔介质中辐射传热的预测替代模型和分析
- 批准号:20541242054124
- 财政年份:2020
- 资助金额:$ 16.58万$ 16.58万
- 项目类别:Standard GrantStandard Grant
Enhancing Quantum Efficiency of Thin Film Solar Cells via Joint Characterization of Radiation and Recombination
通过辐射和复合的联合表征提高薄膜太阳能电池的量子效率
- 批准号:21030082103008
- 财政年份:2020
- 资助金额:$ 16.58万$ 16.58万
- 项目类别:Standard GrantStandard Grant
Enhancing Quantum Efficiency of Thin Film Solar Cells via Joint Characterization of Radiation and Recombination
通过辐射和复合的联合表征提高薄膜太阳能电池的量子效率
- 批准号:19319661931966
- 财政年份:2019
- 资助金额:$ 16.58万$ 16.58万
- 项目类别:Standard GrantStandard Grant
相似国自然基金
MRI融合多组学特征量化高级别成人型弥漫性脑胶质瘤免疫微环境并预测术后复发风险的研究
- 批准号:82302160
- 批准年份:2023
- 资助金额:30 万元
- 项目类别:青年科学基金项目
数字机理耦合建模联动元知识决策的电主轴轴承预测性维护方法研究
- 批准号:52305589
- 批准年份:2023
- 资助金额:30 万元
- 项目类别:青年科学基金项目
头部激波和熵层对高超声速圆锥边界层感受性的影响及SF-HLNS预测方法
- 批准号:12372222
- 批准年份:2023
- 资助金额:53 万元
- 项目类别:面上项目
复杂环境下旅游需求预测的非平稳性溯源、稳健建模与精度提升策略研究
- 批准号:72374083
- 批准年份:2023
- 资助金额:41 万元
- 项目类别:面上项目
广域残积土滑坡强降雨触发机制与危险性动态预测研究
- 批准号:42301103
- 批准年份:2023
- 资助金额:30 万元
- 项目类别:青年科学基金项目
相似海外基金
Surrogate Augmented Deep Predictive Learning for Retinopathy of Prematurity
早产儿视网膜病变的替代增强深度预测学习
- 批准号:1074028910740289
- 财政年份:2023
- 资助金额:$ 16.58万$ 16.58万
- 项目类别:
Characterizing Evoked Potentials of Deep Brain Stimulation for Parkinson's Disease
表征帕金森病深部脑刺激的诱发电位
- 批准号:1060537010605370
- 财政年份:2023
- 资助金额:$ 16.58万$ 16.58万
- 项目类别:
Clinical Trial Readiness for Monitoring Muscle Inflammation in Duchenne Muscular Dystrophy
监测杜氏肌营养不良症肌肉炎症的临床试验准备
- 批准号:1072546510725465
- 财政年份:2023
- 资助金额:$ 16.58万$ 16.58万
- 项目类别:
Novel ultrahigh speed swept source OCT angiography methods in diabetic retinopathy
糖尿病视网膜病变的新型超高速扫源 OCT 血管造影方法
- 批准号:1065664410656644
- 财政年份:2023
- 资助金额:$ 16.58万$ 16.58万
- 项目类别:
Brain-Imaging Markers of Neurotoxicity and Long-Term Outcomes after CAR-T Cell Therapy
CAR-T 细胞治疗后神经毒性和长期结果的脑成像标志物
- 批准号:1065710610657106
- 财政年份:2023
- 资助金额:$ 16.58万$ 16.58万
- 项目类别: