图像分割变分模型的非重叠型区域分解算法研究
项目介绍
AI项目解读
基本信息
- 批准号:11701418
- 项目类别:青年科学基金项目
- 资助金额:25.0万
- 负责人:
- 依托单位:
- 学科分类:A0504.微分方程数值解
- 结题年份:2020
- 批准年份:2017
- 项目状态:已结题
- 起止时间:2018-01-01 至2020-12-31
- 项目参与者:刘璐; 孟巧珍; 赵自娟;
- 关键词:
项目摘要
Image segmentation is an important research topics in digital image processing and computer vision. With the development of image acquisition technology, the resolution and dimension of the images keep growing. It is of great significance to develop efficient and stable numerical algorithms for large-scale image segmentation problems. Based on our previous works, we plan to design parallel algorithms for variational image segmentation models by making use of domain decomposition method and multigrid technique, and analyze the convergence of the proposed algorithms. Firstly, we will investigate the non-overlapping domain decomposition method for the dual formulation of convex Chan-Vese model, i.e., continuous max flow model, and use two level method to enhance the global information for fast convergence. Secondly, we will further improve the non-overlapping domain decomposition method for convex Chan-Vese model based on the multigrid technique with the piecewise constant basis function,which can alleviate its sensitivity to regularization parameter and promote its stability. Finally, thanks to the well extendibility of the continuous max flow model, we will develop the non-overlapping domain decomposition method for convex Potts model, which will realize the fast multi-phase segmentation of image. Our research results will be helpful to solve large-scale image segmentation problems in industry, remote sensing and medical science, etc.
图像分割是数字图像处理和计算机视觉领域的重要研究课题。随着采集技术的发展,图像的分辨率不断提高,维数也不断增加。发展高效稳定的数值方法, 对解决大尺度图像的分割问题具有重要意义。本项目拟在前期工作的基础上,运用区域分解方法和多重网格技术,研究图像分割中变分模型的可并行化算法,并分析算法的收敛性。首先,研究凸松弛Chan-Vese模型对偶问题(即连续最大流模型)的非重叠型区域分解算法,并使用二重网格技术增加模型的全局信息,以提高算法的收敛速度;其次,利用基于分片常数基函数的多重网格技术,改善凸松弛Chan-Vese模型的非重叠型区域分解算法对正则化参数的敏感度,以提高算法的稳定性;最后,利用连续最大流模型良好的可扩展性,研究凸松弛Potts模型的非重叠型区域分解算法,以实现图像的多相位快速分割。本项目的研究成果将有助于解决工业、遥感、医学等领域的大尺度图像分割问题。
结项摘要
计算机视觉和医疗信息等领域的大量问题都离不开图像处理技术。随着图像采集和存储技术的不断提高,图像维数越来越大,细节越来越丰富,因此亟需更有效的数学模型和高效的数值算法用以满足人们对于图像处理精度和速度的要求。本项目基于这一背景,主要研究了下列内容:.(1)图像处理总变差正则化模型和曲率正则化模型的单水平及多水平区域分解算法研究。我们运用非重叠型区域分解方法设计了总变差正则化图像分割、去噪和修补模型的可并行化算法,算法只在子区域的一层边界传递信息,降低了通讯代价。由于算法是在离散框架下导出,可以得到算法的收敛性。特别地,对于具有强凸数据项的图像去噪问题,我们可以得到具有平方收敛阶的非重叠型区域分解算法。我们进一步研究了总变差正则化模型的多水平的区域分解算法,运用分片常数函数的子空间矫正法保证每个支集上的计算只发生在边界处,且运用四色分解实现了单水平问题的并行计算。此外,我们利用微分几何理论,设计了曲率正则化模型的多水平区域分解算法,有效地提高了高阶正则项的计算效率。.(2)图像处理的非凸正则化模型和算法的研究。非凸正则化方法在图像处理问题上取得了很好的数值效果,特别是曲率正则化可以很好的保持图像的对比度、边和角的那个几何信息。我们提出了全新的曲率正则项,包括Weingarten map,平均曲率、高斯曲率和全曲率,并设计了高效的数值算法应用于各类图像处理问题,如分割、去噪、修补和重建等,均取了很好的处理效果。.(3)模型驱动的深度学习方法的研究。针对深度学习对于数据迁移性和稳定性等问题,我们利用数据先验和数学模型,提出了模型驱动的深度网络,降低了模型对于图像噪声的敏感性、提高了不完备数据的CT重建效果和三维图像分割的信息融合。.以上的模型和算法在效果和效率上都比以往的方法有较大的提升,有望应用于计算机视觉和医学影像分析等实际问题中。
项目成果
期刊论文数量(12)
专著数量(0)
科研奖励数量(0)
会议论文数量(3)
专利数量(0)
Total Variation-Based Phase Retrieval for Poisson Noise Removal
用于泊松噪声消除的基于总变分的相位检索
- DOI:10.1137/16m1103270
- 发表时间:2018-01-01
- 期刊:SIAM JOURNAL ON IMAGING SCIENCES
- 影响因子:2.1
- 作者:Chang, Huibin;Lou, Yifei;Marchesini, Stefano
- 通讯作者:Marchesini, Stefano
Adaptive trainable non-linear reaction diffusion for Rician noise removal
用于莱斯噪声消除的自适应可训练非线性反应扩散
- DOI:10.1049/iet-ipr.2019.1097
- 发表时间:2020-09
- 期刊:IET Image Processing
- 影响因子:2.3
- 作者:Huan Yang;Hongwei Li;Yuping Duan
- 通讯作者:Yuping Duan
The TVp Regularized Mumford-Shah Model for Image Labeling and Segmentation
用于图像标记和分割的 TVp 正则化 Mumford-Shah 模型
- DOI:10.1109/tip.2020.2997524
- 发表时间:2020-06
- 期刊:IEEE Transactions on Image Processing
- 影响因子:10.6
- 作者:Yutong Li;Chunlin Wu;Yuping Duan
- 通讯作者:Yuping Duan
A weighted bounded Hessian variational model for image labeling and segmentation
用于图像标记和分割的加权有界 Hessian 变分模型
- DOI:10.1016/j.sigpro.2020.107564
- 发表时间:2020-08
- 期刊:Signal Processing
- 影响因子:4.4
- 作者:Yijie Yang;Qiuxiang Zhong;Yuping Duan;Tieyong Zeng
- 通讯作者:Tieyong Zeng
Learned Full-Sampling Reconstruction From Incomplete Data
学会了从不完整数据中进行全采样重建
- DOI:10.1109/tci.2020.2996751
- 发表时间:2020
- 期刊:IEEE Transactions on Computational Imaging
- 影响因子:5.4
- 作者:Cheng Weilin;Wang Yu;Li Hongwei;Duan Yuping
- 通讯作者:Duan Yuping
数据更新时间:{{ journalArticles.updateTime }}
{{
item.title }}
{{ item.translation_title }}
- DOI:{{ item.doi || "--"}}
- 发表时间:{{ item.publish_year || "--" }}
- 期刊:{{ item.journal_name }}
- 影响因子:{{ item.factor || "--"}}
- 作者:{{ item.authors }}
- 通讯作者:{{ item.author }}
数据更新时间:{{ journalArticles.updateTime }}
{{ item.title }}
- 作者:{{ item.authors }}
数据更新时间:{{ monograph.updateTime }}
{{ item.title }}
- 作者:{{ item.authors }}
数据更新时间:{{ sciAawards.updateTime }}
{{ item.title }}
- 作者:{{ item.authors }}
数据更新时间:{{ conferencePapers.updateTime }}
{{ item.title }}
- 作者:{{ item.authors }}
数据更新时间:{{ patent.updateTime }}
其他文献
胎盘来源的miRNAs在子痫前期发生发展的作用及临床价值
- DOI:--
- 发表时间:2019
- 期刊:中华检验医学杂志
- 影响因子:--
- 作者:段玉萍;姜连生;李莉;厉倩
- 通讯作者:厉倩
白细胞介素-6、微小 RNA-210与子痫前期的相关性及其临床价值
- DOI:--
- 发表时间:2020
- 期刊:国际检验医学杂志
- 影响因子:--
- 作者:段玉萍;钟丽丽;洪茂;谢骊;蔡雷鸣;吴晶晶;陈雄;厉倩
- 通讯作者:厉倩
其他文献
{{
item.title }}
{{ item.translation_title }}
- DOI:{{ item.doi || "--" }}
- 发表时间:{{ item.publish_year || "--"}}
- 期刊:{{ item.journal_name }}
- 影响因子:{{ item.factor || "--" }}
- 作者:{{ item.authors }}
- 通讯作者:{{ item.author }}
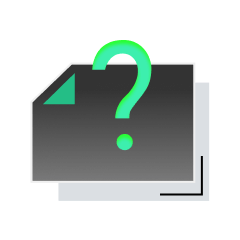
内容获取失败,请点击重试
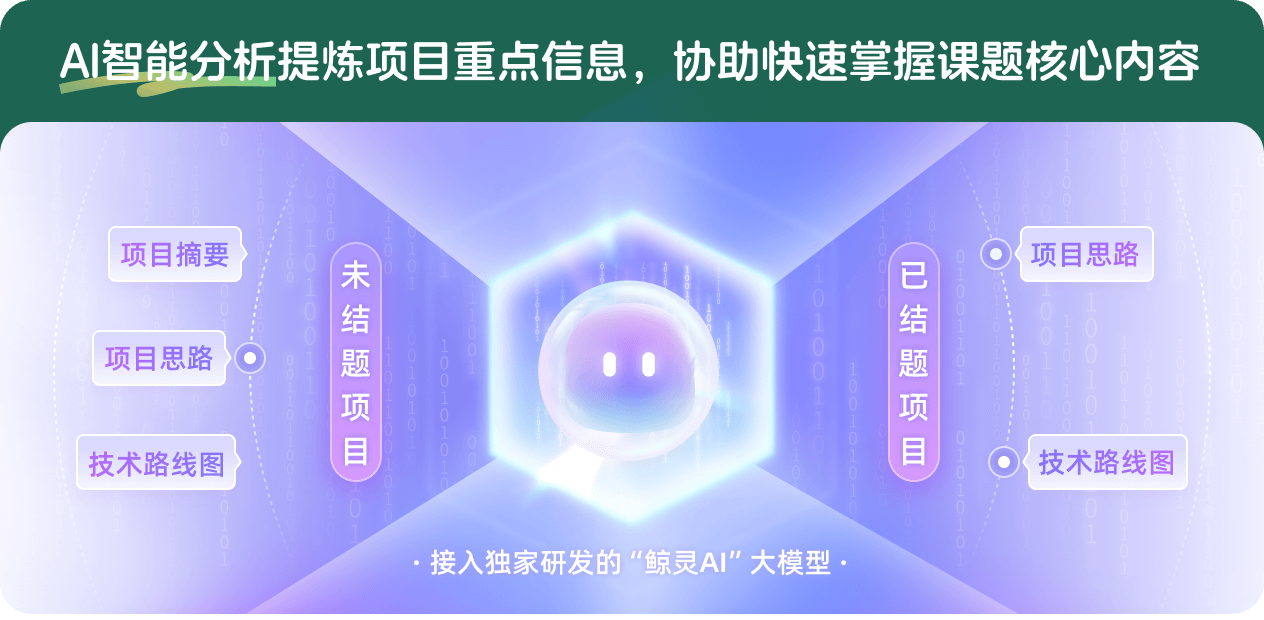
查看分析示例
此项目为已结题,我已根据课题信息分析并撰写以下内容,帮您拓宽课题思路:
AI项目摘要
AI项目思路
AI技术路线图
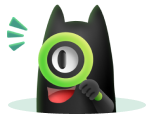
请为本次AI项目解读的内容对您的实用性打分
非常不实用
非常实用
1
2
3
4
5
6
7
8
9
10
您认为此功能如何分析更能满足您的需求,请填写您的反馈:
段玉萍的其他基金
图像处理问题中的两类曲率正则化方法及应用
- 批准号:
- 批准年份:2020
- 资助金额:51 万元
- 项目类别:面上项目
相似国自然基金
{{ item.name }}
- 批准号:{{ item.ratify_no }}
- 批准年份:{{ item.approval_year }}
- 资助金额:{{ item.support_num }}
- 项目类别:{{ item.project_type }}
相似海外基金
{{
item.name }}
{{ item.translate_name }}
- 批准号:{{ item.ratify_no }}
- 财政年份:{{ item.approval_year }}
- 资助金额:{{ item.support_num }}
- 项目类别:{{ item.project_type }}