基于生态位和协同演化理论的制造企业信息化演进机制研究
项目介绍
AI项目解读
基本信息
- 批准号:71171145
- 项目类别:面上项目
- 资助金额:42.0万
- 负责人:
- 依托单位:
- 学科分类:G0112.信息系统与管理
- 结题年份:2015
- 批准年份:2011
- 项目状态:已结题
- 起止时间:2012-01-01 至2015-12-31
- 项目参与者:牛占文; 胡明艳; 赵楠; 霍明; 尹睿智; 张冬明; 程刚; 潘翊;
- 关键词:
项目摘要
"两化"融合是国家发展战略,制造业信息化已成为促进制造业发展的重要手段,但目前企业信息化研究大量基于静态层面,缺乏对企业信息化生态特征的演化分析。本课题针对制造企业信息化的历史发展和动态复杂状况,从信息化演进入手,主要研究:(1)在生态位、企业演化论、自组织理论的基础上,构建制造企业信息化演进模型,研究制造企业信息化演进机制;(2)以中小企业集群为对象,研究企业集群信息化协同演进机制;(3)结合中国制造业信息化调查数据,智能分析制造企业信息化演进关键影响因素,量化、实证制造企业信息化演进模型和产业集群协同演化模型。本课题对企业信息化过程的生态特征进行理论探索,形成对企业信息化实践的有益指导,为企业信息化战略制定与规划提供理论支撑和诊断分析,为行业和区域信息化政策制定提供更为全面的理论依据。
结项摘要
“两化”融合成为国家发展战略,大力推进制造业信息化成为国家科技中长期发展规划中促进制造业发展的三大方向之一。但我国制造企业信息化推进过程中,仍然存在诸多问题,比如信息化效益不突出,信息化发展的内部机制不明确,缺乏对企业信息化生命特征的演化分析。.课题围绕制造企业信息化发展过程,对制造企业信息化关键因素、演进机制和协同发展机制进行研究。课题以自组织理论为基础,对企业信息化演进机制进行研究,实证IT投资回报率是主导企业信息化演化的序参量。将企业信息化演进模型推广到产业链和互联网环境下,构建了产业链信息化发展主要影响因素的协同关系。对云制造平台企业生态位宽度及重叠度进行了研究,构建了个体间竞争合作演化模型。.课题构建了企业信息化价值链路,为信息化关键因素分析指出了新路径,缩短了信息化投入与目标的中间过程。通过研究企业能力体系、能力价值量化评估方法和主成份分析等智能分析技术,依据全国制造业信息化数据统计调查及部分企业调研数据,实证分析了制造业信息化关键因素。.课题建立了企业能力体系的基本构成,量化了能力效益和信息化价值的计算方法,明确信息化投入、业务信息能力和信息化战略地位是影响信息化应用水平的关键因素,描述了企业信息化和产业链信息化协同演进机制,成果可形成对企业信息化实践的有益指导,为企业信息化战略制定与规划提供理论支撑和诊断分析。
项目成果
期刊论文数量(17)
专著数量(0)
科研奖励数量(0)
会议论文数量(2)
专利数量(0)
Robust Principal Component Analysis Algorithm Based on Nonparametric Correlation Coefficient Matrix
基于非参数相关系数矩阵的鲁棒主成分分析算法
- DOI:10.4028/www.scientific.net/amr.989-994.2613
- 发表时间:2014-07
- 期刊:Applied Mechanics and Materials
- 影响因子:--
- 作者:Zhao Nan;Shao Hongyu
- 通讯作者:Shao Hongyu
基于不完全信息博弈的云制造环境下信任形成机制研究
- DOI:--
- 发表时间:2015
- 期刊:计算机集成制造系统
- 影响因子:--
- 作者:毕克克;牛占文;赵楠;彭巍
- 通讯作者:彭巍
基于AHP与IFS的单件生产模式下制造需求与资源匹配算法
- DOI:--
- 发表时间:2015
- 期刊:中国机械工程
- 影响因子:--
- 作者:牛占文;赵楠;彭巍;仝克宁
- 通讯作者:仝克宁
基于MRO的重型机床闭环PLM模型及关键技术研究
- DOI:--
- 发表时间:2014
- 期刊:现代企业教育
- 影响因子:--
- 作者:郑庆;仝克宁;赵楠;邵宏宇
- 通讯作者:邵宏宇
Key Factor Extraction of Supply Chain Performance Based on Heterogeneous Selective Ensemble PCA
基于异构选择性集成PCA的供应链绩效关键因子提取
- DOI:10.4028/www.scientific.net/amm.397-400.2626
- 发表时间:2013-09
- 期刊:Applied Mechanics and Materials
- 影响因子:--
- 作者:张聪慧;赵楠;邵宏宇
- 通讯作者:邵宏宇
数据更新时间:{{ journalArticles.updateTime }}
{{
item.title }}
{{ item.translation_title }}
- DOI:{{ item.doi || "--"}}
- 发表时间:{{ item.publish_year || "--" }}
- 期刊:{{ item.journal_name }}
- 影响因子:{{ item.factor || "--"}}
- 作者:{{ item.authors }}
- 通讯作者:{{ item.author }}
数据更新时间:{{ journalArticles.updateTime }}
{{ item.title }}
- 作者:{{ item.authors }}
数据更新时间:{{ monograph.updateTime }}
{{ item.title }}
- 作者:{{ item.authors }}
数据更新时间:{{ sciAawards.updateTime }}
{{ item.title }}
- 作者:{{ item.authors }}
数据更新时间:{{ conferencePapers.updateTime }}
{{ item.title }}
- 作者:{{ item.authors }}
数据更新时间:{{ patent.updateTime }}
其他文献
基于RS与AHP的中小企业云制造模式下多服务主体信用评价体系构建
- DOI:--
- 发表时间:2013
- 期刊:计算机集成制造系统
- 影响因子:--
- 作者:郭伟;仝克宁;邵宏宇;王磊;郑庆
- 通讯作者:郑庆
基于自组织理论的企业IT能力研究
- DOI:--
- 发表时间:2015
- 期刊:管理现代化
- 影响因子:--
- 作者:霍明;胡继连;邵宏宇;安康
- 通讯作者:安康
其他文献
{{
item.title }}
{{ item.translation_title }}
- DOI:{{ item.doi || "--" }}
- 发表时间:{{ item.publish_year || "--"}}
- 期刊:{{ item.journal_name }}
- 影响因子:{{ item.factor || "--" }}
- 作者:{{ item.authors }}
- 通讯作者:{{ item.author }}
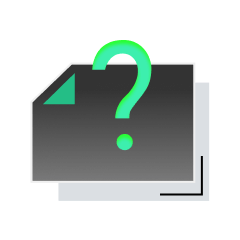
内容获取失败,请点击重试
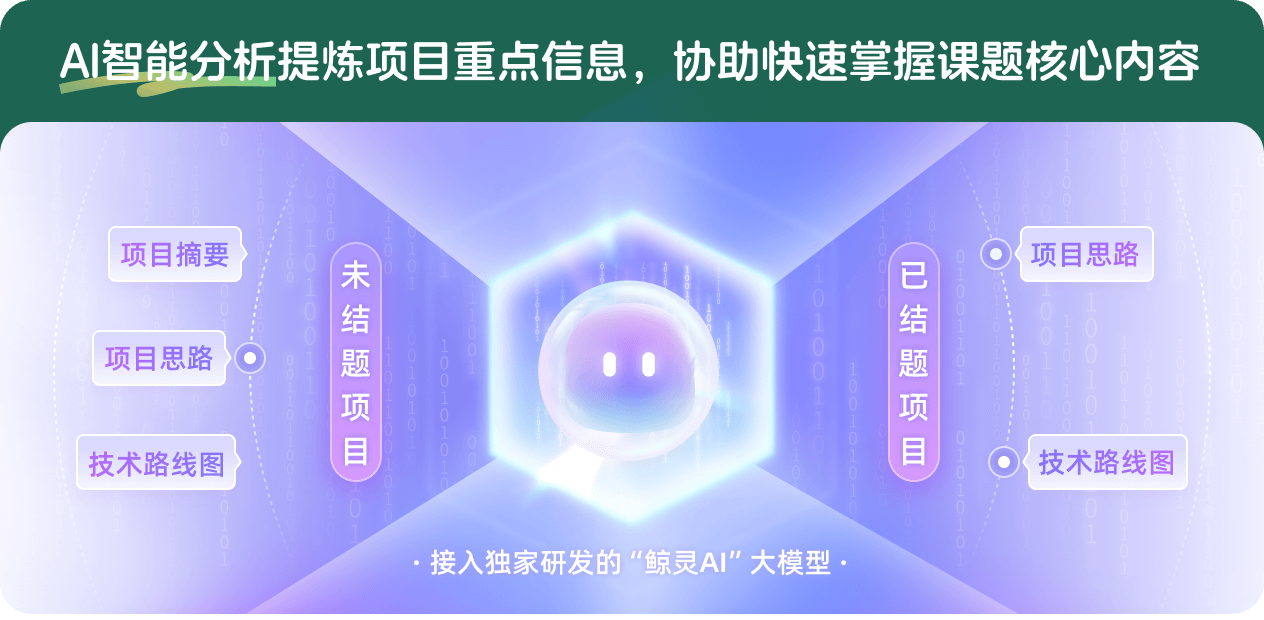
查看分析示例
此项目为已结题,我已根据课题信息分析并撰写以下内容,帮您拓宽课题思路:
AI项目摘要
AI项目思路
AI技术路线图
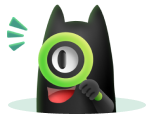
请为本次AI项目解读的内容对您的实用性打分
非常不实用
非常实用
1
2
3
4
5
6
7
8
9
10
您认为此功能如何分析更能满足您的需求,请填写您的反馈:
相似国自然基金
{{ item.name }}
- 批准号:{{ item.ratify_no }}
- 批准年份:{{ item.approval_year }}
- 资助金额:{{ item.support_num }}
- 项目类别:{{ item.project_type }}
相似海外基金
{{
item.name }}
{{ item.translate_name }}
- 批准号:{{ item.ratify_no }}
- 财政年份:{{ item.approval_year }}
- 资助金额:{{ item.support_num }}
- 项目类别:{{ item.project_type }}