基于电子病历数据挖掘的急性冠脉综合征风险评估及干预效果分析方法研究
项目介绍
AI项目解读
基本信息
- 批准号:61672450
- 项目类别:面上项目
- 资助金额:62.0万
- 负责人:
- 依托单位:
- 学科分类:F0214.新型计算及其应用基础
- 结题年份:2020
- 批准年份:2016
- 项目状态:已结题
- 起止时间:2017-01-01 至2020-12-31
- 项目参与者:董蔚; 季磊; 燕辉; 陈佩佩; 周浩; 张颖; 胡丹青; 陆易; 杨智翔;
- 关键词:
项目摘要
Acute coronary syndrome (ACS) is very harmful to humans, therefore both comprehensive ACS risk assessment and effect analysis of corresponding medical interventions are critical/essential for efficient ACS treatment and management. Traditional ACS risk assessment models, e.g., GRACE, TIMI and Pursuit, etc., have several serious limitations. In particular, they consider a small set of handpicked factors of ACS and lack the ability to discover potential risk factors for both ischemic and hemorrhagic adverse events in an integrated manner. As well, existing approaches lack the ability to look insight into the behaviors of ACS medical interventions on the occurrences of major adverse events in clinical settings, and thus cannot provide sufficient information for the intervention effect analysis of ACS..Recently, with the rapid development of healthcare information systems, a large collection of electronic medical records (EMRs) has become available, which provides the opportunity to study medical cases, evidence and knowledge for various medical applications. In particular, heterogeneous clinical information of patients (e.g., the patient demographics, laboratory tests results, radiological examination reports, and medical interventions, etc.) are regularly recorded in EMRs, and thus suggest data-centric and hypothesis-free approaches from which data mining techniques can be utilized for stratifying clinical risks of ACS patients and optimizing the strategies of performing appropriate medical interventions in ACS treatment processes. .To this end, the target of this research is to propose a data mining methodology that can be applied to EMRs to assess clinical risks of ACS patients and analyze ACS medical interventions. An ACS lexicon is to be constructed to assist structured extraction and semantic processing of EMRs. Based on the processed EMR data set, a novel data mining-based approach is proposed to generate an ACS risk assessment model for predicting the occurrences of both ischemic and hemorrhagic adverse events, and assessing the entire risk of an ACS patient in an integrated manner. In addition, we plan to identify causal patterns between medical interventions and main adverse events such that diagnostic information can be provided to assist clinicians to analyze the effects of ACS medical interventions, reduce the occurrences of adverse events, and improve ACS treatment practice in an informative manner. .The issue of meaningful or secondary use of EMRs represents a promising and interesting research direction in medical informatics. Our research proposes novel approaches to explore the huge potential of EMRs for ACS risk assessment and medical intervention effect analysis, as a crucial advantage over traditional techniques for ACS prevention and treatment.
急性冠脉综合征(ACS)对人类危害极大,全面准确的风险评估和干预效果分析是ACS疾病防治的基础。现有ACS风险评估方法存在风险因素纳入不全的问题,缺乏发现和整合缺血性事件和出血事件的风险因素、评定ACS总风险的能力。此外,临床缺少有效分析医疗干预应用效果的方法。电子病历记录了患者疾病诊疗真实信息,挖掘电子病历数据可望为评估疾病风险、分析干预效果提供潜在规律性知识。为此,本项目研究基于电子病历数据挖掘的ACS风险评估与干预效果分析方法体系。首先建立ACS数据词典,对电子病历数据进行结构化提取和语义预处理。在此基础上,建立ACS风险评估模型,发现和整合缺血性事件和出血事件的潜在风险因素,评估ACS患者疾病总风险;挖掘医疗干预与临床主要不良事件的关联知识,分析干预应用效果,为降低不良事件发生风险提供参考依据。项目研究为ACS疾病防治提供了新的思路和方法,对电子病历的研究与应用也具有重要科学意义。
结项摘要
作为一种严重的急性心血管疾病,急性冠脉综合征是近一半心血管疾病的死亡归因,一直以来受到临床的广泛关注。针对急性冠脉综合征患者的风险评估与干预效果分析,能够针对患者预后提供预警性信息,并为临床诊疗决策提供支持。.电子病历记录了患者疾病诊疗真实信息,挖掘电子病历数据可望为评估疾病风险、分析干预效果提供潜在规律性知识。本项目首先针对电子病历数据的提取和预处理问题开展研究,提出了电子病历数据结构化提取与语义处理方法,为后续研究的开展提供数据基础。在此基础上,本项目挖掘真实世界临床数据,发现潜在的风险因素,构建数据驱动的急性冠脉综合征风险评估模型,实现对急性冠脉综合征等重大心血管病的短期风险精准预警和长期病情演变判断。在疾病风险评估基础上,本项目针对利用真实世界数据进行干预效果评估所面临的反事实结果缺失和选择偏误问题进行方法探究,提出了基于解耦表征学习的干预效果分析方法,挖掘医疗干预手段与干预结果之间的关联模式,分析医疗干预措施对患者预后的影响,为调整和改善不合理的干预措施,降低临床主要不良事件的发生风险提供依据。最后,本研究还开发了心血管疾病风险评估与干预效果分析决策支持系统,对本项目中的研究成果进行整合和部署应用。.本项目顺利完成了原定研究目标,解决了从电子病历数据提取与处理,到疾病风险评估和干预效果评估的一系列相关领域重要科学问题,取得了丰硕的成果:培养博士研究生3名,硕士研究生6名;以第一作者或通讯作者发表SCI期刊论文17篇(第一标注12 篇,第二标注5 篇); 发表会议论文1篇(第一标注);研发急性冠脉综合征风险评估与干预效果分析系统一个;并作为主要创新成果之一获2017年度全军科技进步一等奖。.本项目研究对疾病的风险评估和预后分析等精准医疗的核心问题进行深入的数据分析、建模和技术创新,充分彰显人工智能技术与循证医疗大数据结合起来对临床诊疗实践产生的叠加价值,对解决上述国家重大需求意义重大,科学意义明显。
项目成果
期刊论文数量(18)
专著数量(0)
科研奖励数量(1)
会议论文数量(1)
专利数量(9)
Interpretable clinical prediction via attention-based neural network
通过基于注意力的神经网络进行可解释的临床预测
- DOI:10.1186/s12911-020-1110-7
- 发表时间:2020-07-09
- 期刊:BMC MEDICAL INFORMATICS AND DECISION MAKING
- 影响因子:3.5
- 作者:Chen, Peipei;Dong, Wei;Huang, Zhengxing
- 通讯作者:Huang, Zhengxing
医疗大数据在心衰中心建设中的作用
- DOI:--
- 发表时间:2018
- 期刊:中国医学前沿杂志(电子版)
- 影响因子:--
- 作者:董蔚;李佳月;黄正行;应俊;王彬华;薛万国;陈韵岱;何昆仑
- 通讯作者:何昆仑
Endpoint prediction of heart failure using electronic health records
使用电子健康记录进行心力衰竭的终点预测
- DOI:10.1016/j.jbi.2020.103518
- 发表时间:2020-09-01
- 期刊:JOURNAL OF BIOMEDICAL INFORMATICS
- 影响因子:4.5
- 作者:Chu, Jiebin;Dong, Wei;Huang, Zhengxing
- 通讯作者:Huang, Zhengxing
Utilizing electronic health records to predict multi-type major adverse cardiovascular events after acute coronary syndrome
利用电子健康记录预测急性冠脉综合征后多类重大心血管不良事件
- DOI:10.1007/s10115-018-1270-2
- 发表时间:2019-09-01
- 期刊:KNOWLEDGE AND INFORMATION SYSTEMS
- 影响因子:2.7
- 作者:Huang, Zhengxing;Lu, Yi;Dong, Wei
- 通讯作者:Dong, Wei
Utilizing Electronic Medical Records to Discover Changing Trends of Medical Behaviors Over Time.
利用电子病历发现医疗行为随时间变化的趋势
- DOI:10.3414/me16-01-0047
- 发表时间:2017-05-05
- 期刊:Methods of information in medicine
- 影响因子:1.7
- 作者:Yin L;Huang Z;Dong W;He C;Duan H
- 通讯作者:Duan H
数据更新时间:{{ journalArticles.updateTime }}
{{
item.title }}
{{ item.translation_title }}
- DOI:{{ item.doi || "--"}}
- 发表时间:{{ item.publish_year || "--" }}
- 期刊:{{ item.journal_name }}
- 影响因子:{{ item.factor || "--"}}
- 作者:{{ item.authors }}
- 通讯作者:{{ item.author }}
数据更新时间:{{ journalArticles.updateTime }}
{{ item.title }}
- 作者:{{ item.authors }}
数据更新时间:{{ monograph.updateTime }}
{{ item.title }}
- 作者:{{ item.authors }}
数据更新时间:{{ sciAawards.updateTime }}
{{ item.title }}
- 作者:{{ item.authors }}
数据更新时间:{{ conferencePapers.updateTime }}
{{ item.title }}
- 作者:{{ item.authors }}
数据更新时间:{{ patent.updateTime }}
其他文献
结构化电子病历数据录入方法
- DOI:--
- 发表时间:--
- 期刊:浙江大学学报(工学版)
- 影响因子:--
- 作者:李昊旻;段会龙;吕旭东;黄正行
- 通讯作者:黄正行
其他文献
{{
item.title }}
{{ item.translation_title }}
- DOI:{{ item.doi || "--" }}
- 发表时间:{{ item.publish_year || "--"}}
- 期刊:{{ item.journal_name }}
- 影响因子:{{ item.factor || "--" }}
- 作者:{{ item.authors }}
- 通讯作者:{{ item.author }}
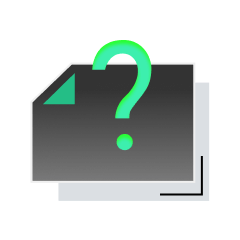
内容获取失败,请点击重试
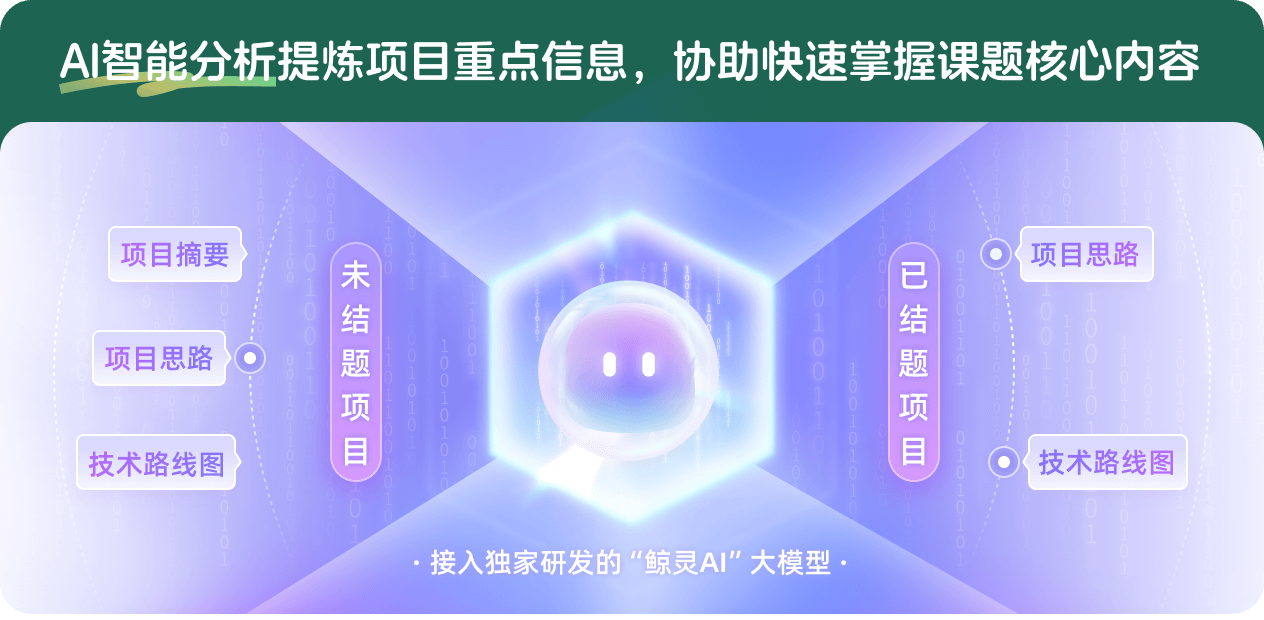
查看分析示例
此项目为已结题,我已根据课题信息分析并撰写以下内容,帮您拓宽课题思路:
AI项目摘要
AI项目思路
AI技术路线图
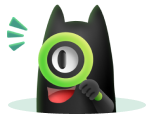
请为本次AI项目解读的内容对您的实用性打分
非常不实用
非常实用
1
2
3
4
5
6
7
8
9
10
您认为此功能如何分析更能满足您的需求,请填写您的反馈:
黄正行的其他基金
基于电子病历数据挖掘的慢性病演变过程分析方法研究
- 批准号:82272129
- 批准年份:2022
- 资助金额:52 万元
- 项目类别:面上项目
基于过程挖掘的临床路径建模与分析方法研究
- 批准号:81101126
- 批准年份:2011
- 资助金额:14.0 万元
- 项目类别:青年科学基金项目
相似国自然基金
{{ item.name }}
- 批准号:{{ item.ratify_no }}
- 批准年份:{{ item.approval_year }}
- 资助金额:{{ item.support_num }}
- 项目类别:{{ item.project_type }}
相似海外基金
{{
item.name }}
{{ item.translate_name }}
- 批准号:{{ item.ratify_no }}
- 财政年份:{{ item.approval_year }}
- 资助金额:{{ item.support_num }}
- 项目类别:{{ item.project_type }}