基于深度神经网络的粗糙表面散射理论研究
项目介绍
AI项目解读
基本信息
- 批准号:11804263
- 项目类别:青年科学基金项目
- 资助金额:20.0万
- 负责人:
- 依托单位:
- 学科分类:A2202.光与物质相互作用
- 结题年份:2021
- 批准年份:2018
- 项目状态:已结题
- 起止时间:2019-01-01 至2021-12-31
- 项目参与者:卢莉萍; 华瑾; 于小宁; 顾永健; 郑潇; 李浩;
- 关键词:
项目摘要
The detection and analysis on light scattering from randomly rough surfaces is of great significance in target detections and identifications, therefore, the study on light scattering calculation and rough surface estimation not only can provide quantitative relation between rough surface and scattering field, but also offers further application potentials. Compared to the experimental research on light scattering, numerical methods attract much attention because they do not rely on complicated devices, and avoid errors inevitably occurred in experimental measurements. However, most traditional numerical methods for light scattering from randomly rough surfaces require ensemble average which often leads to low computational efficiency; besides, the existing methods for rough surface estimation usually have poor reconstruction precision. In order to overcome the disadvantages of these traditional methods, this project will realize rapid and accurate light scattering computation and rough surface estimation by introducing deep neural networks into the research on light scattering from randomly rough surfaces. First, the light scattering calculation model will be constructed to solve the forward scattering problem according the training data obtained via the classical light scattering computation algorithms. Next, with the exchange between the input as rough surface and the output as the light scattering field in the previous light scattering calculation model, the rough surface estimation model will also be obtained in order to retrieve the high-precision rough surface characteristics from complete or partial of light scattering distributions as the inverse problem solution. The project intends to build a bidirectional quantitative relation between the rough surface and the light scattering distribution, and it is expected to provide important references for applications such as laser radar, laser detection and target recognition.
随机粗糙表面散射光的检测与分析在目标探测与识别等领域中具有重要研究意义与价值,因此开展散射场计算和散射面参数估计的研究不仅有助于定量探索表面与散射场的关系,还可为其应用奠定坚实的基础。相比于实验研究,数值方法无需复杂装置,且避免了测量误差,因而获得了广泛关注。然而传统的散射场数值计算方法多依赖系综平均,计算效率低;另外现有的散射面参数估计方法通常重构精度较差。为了克服传统方法的不足,本项目将深度神经网络引入随机粗糙表面光散射场的研究中,首先构建散射场计算模型,使用经典方法构建数据集开展模型训练以实现不依赖系综平均的散射场快速精确计算。在此基础上,将代表散射面特征的输入与代表散射场的输出互换,进而构建散射场分析模型,实现从全部或部分散射信息快速重构高精度的散射表面特征参数。本项目拟最终构建散射表面与散射分布的双向定量化关系,因此有望为激光雷达、激光探测与目标识别等应用提供重要参考。
结项摘要
在光波段下大部分表面被认为是粗糙表面,由于表面散射光中含有大量散射表面的信息,因此在遥感和目标识别研究中粗糙表面光散射理论是重要理论基础。开展表面光散射理论研究不仅有助于定量探索表面与散射场的关系,还可为其应用奠定坚实的基础。相比于实验研究,数值研究无需复杂装置,且避免了测量误差,因而获得了广泛关注,然而传统散射场数值计算方法多依赖系综平均,因此计算强度大,计算效率低。为了克服传统方法的不足,本项目引入了深度神经网络到散射研究中,首先构建了散射场信息与随机粗糙表面有效参数的多参数模型,获得大量散射场数据;设计了基于深度神经网络的粗糙表面全角度空间散射场计算模型,用于从已知表面和入射光参数重建光散射场;构建了散射场分析模型,用于从光散射场、表面材料和入射光计算随机粗糙表面粗糙度参数;基于本项目的大量研究数据和算法,设计了表面散射计算软件。以一维随机粗糙表面光散射场正问题和逆问题计算为例,证明了所采用的深度神经网络能够快速提供精确、稳定的结果,说明深度学习是一个非常好的数值重构散射数据的方法,另外深度学习也为更广泛范围内的数值计算光散射问题开辟了一条新途径。因此,基于深度学习的方法可以避免了经典方法所需的大量重复过程,这是该方法的关键优势。本项目研究成果可为激光雷达、激光探测与目标识别等应用提供重要参考。
项目成果
期刊论文数量(8)
专著数量(0)
科研奖励数量(0)
会议论文数量(4)
专利数量(1)
Fuzzy recognition of missile borne multi-line array infrared detection based on size calculating
基于尺寸计算的弹载多线阵红外探测模糊识别
- DOI:10.1016/j.dt.2020.06.004
- 发表时间:2021-07-10
- 期刊:DEFENCE TECHNOLOGY
- 影响因子:5.1
- 作者:Lei, Bing-Shan;Li, Jing;Yan, Ke-Ding
- 通讯作者:Yan, Ke-Ding
深粗糙随机表面光散射特性数值研究
- DOI:--
- 发表时间:--
- 期刊:上海电力大学学报
- 影响因子:--
- 作者:闫克丁;赵英然;于小宁;杨建华;杨树蔚
- 通讯作者:杨树蔚
Programmable liquid crystal display based noise reduced dynamic synthetic coded aperture imaging camera (NoRDS-CAIC)
基于可编程液晶显示的降噪动态合成编码孔径成像相机(NoRDS-CAIC)
- DOI:10.1364/oe.385547
- 发表时间:2020-02-17
- 期刊:OPTICS EXPRESS
- 影响因子:3.8
- 作者:Jiang, Zhilong;Yang, Shuwei;Wang, Shouyu
- 通讯作者:Wang, Shouyu
PhaseRMiC: phase real-time microscope camera for live cell imaging.
PhaseRMiC:用于活细胞成像的相位实时显微镜相机。
- DOI:10.1364/boe.430115
- 发表时间:2021-07
- 期刊:Biomedical optics express
- 影响因子:3.4
- 作者:Chao Chen;Yu-Nan Lu;Huachuan Huang;Keding Yan;Zhilong Jiang;Xiaoliang He;Yan Kong;Cheng Liu;Fei Liu;Liang Xue;Shouyu Wang
- 通讯作者:Shouyu Wang
基于穆勒矩阵的金属和电介质识别方法
- DOI:10.3788/lop56.142401
- 发表时间:2019
- 期刊:激光与光电子学进展
- 影响因子:--
- 作者:闫振纲;孙卫平;李杰;武江鹏;朱新宏;袁梦笛;薛亮;闫克丁
- 通讯作者:闫克丁
数据更新时间:{{ journalArticles.updateTime }}
{{
item.title }}
{{ item.translation_title }}
- DOI:{{ item.doi || "--"}}
- 发表时间:{{ item.publish_year || "--" }}
- 期刊:{{ item.journal_name }}
- 影响因子:{{ item.factor || "--"}}
- 作者:{{ item.authors }}
- 通讯作者:{{ item.author }}
数据更新时间:{{ journalArticles.updateTime }}
{{ item.title }}
- 作者:{{ item.authors }}
数据更新时间:{{ monograph.updateTime }}
{{ item.title }}
- 作者:{{ item.authors }}
数据更新时间:{{ sciAawards.updateTime }}
{{ item.title }}
- 作者:{{ item.authors }}
数据更新时间:{{ conferencePapers.updateTime }}
{{ item.title }}
- 作者:{{ item.authors }}
数据更新时间:{{ patent.updateTime }}
其他文献
其他文献
{{
item.title }}
{{ item.translation_title }}
- DOI:{{ item.doi || "--" }}
- 发表时间:{{ item.publish_year || "--"}}
- 期刊:{{ item.journal_name }}
- 影响因子:{{ item.factor || "--" }}
- 作者:{{ item.authors }}
- 通讯作者:{{ item.author }}
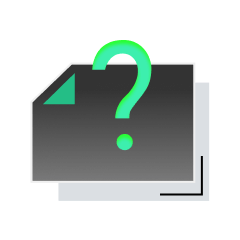
内容获取失败,请点击重试
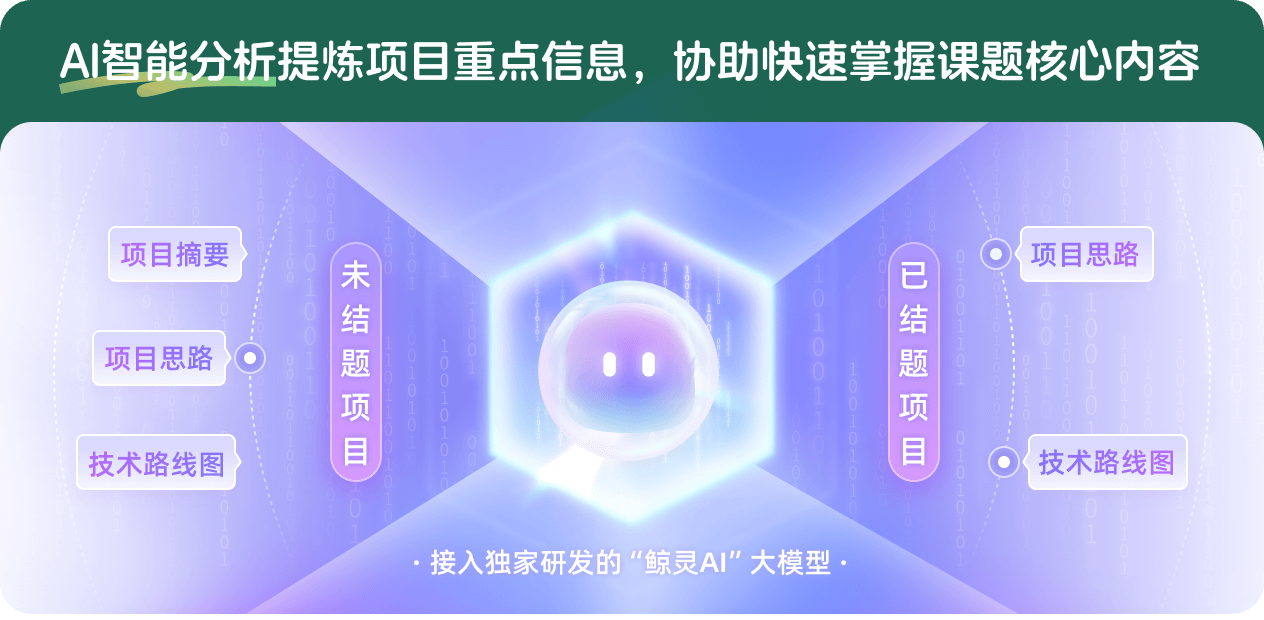
查看分析示例
此项目为已结题,我已根据课题信息分析并撰写以下内容,帮您拓宽课题思路:
AI项目摘要
AI项目思路
AI技术路线图
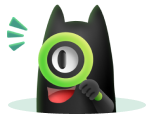
请为本次AI项目解读的内容对您的实用性打分
非常不实用
非常实用
1
2
3
4
5
6
7
8
9
10
您认为此功能如何分析更能满足您的需求,请填写您的反馈:
相似国自然基金
{{ item.name }}
- 批准号:{{ item.ratify_no }}
- 批准年份:{{ item.approval_year }}
- 资助金额:{{ item.support_num }}
- 项目类别:{{ item.project_type }}
相似海外基金
{{
item.name }}
{{ item.translate_name }}
- 批准号:{{ item.ratify_no }}
- 财政年份:{{ item.approval_year }}
- 资助金额:{{ item.support_num }}
- 项目类别:{{ item.project_type }}