不确定工况PEMFC-SC混合能源多目标实时优化管理方法研究
项目介绍
AI项目解读
基本信息
- 批准号:61603337
- 项目类别:青年科学基金项目
- 资助金额:18.0万
- 负责人:
- 依托单位:
- 学科分类:F0302.控制系统与应用
- 结题年份:2019
- 批准年份:2016
- 项目状态:已结题
- 起止时间:2017-01-01 至2019-12-31
- 项目参与者:于在河; 李修亮; 张蔚; 王新光; 赵吉壮; 黄少会;
- 关键词:
项目摘要
In fuel cell hybrid electric new energy vehicle area, the energy optimal control under uncertainty running conditions is hot spots of hybrid energy management system, there are important research and application significances. A fast sliding window kernel PCA is first proposed to obtain the vehicle running signal reliably by computing complexity and precision analysis, and driving condition prediction model is constructed by artificial intelligence method, such as deep learning neural network; Set wave oscillation as the life cycle index and acceleration time as driving performance, the multi-objective constraint optimization scheme is then studied to satisfy the SOC inequality constraint of super capacity and vehicle power demand equality constraint; Combined driving condition predictive model with Pareto front distributed characteristics, a new fuzzy energy real time management rule based on uncertain driving condition is put forward and illustrated by simulations and experiments. The project is mainly focus on the problem of real time energy management and control for PEMFC-SC, which will provide a new method and thinking to solve the application bottleneck of fuel cell.
在燃料电池混合动力新能源汽车领域,不确定车辆运行工况下的能量优化控制是混合能源管理系统的研究热点,有重要的理论和应用研究意义。首先,研究加窗KPCA快速主元分析方法实现多维车辆运行信息的特征提取,并进行计算复杂度和精度分析。在此基础上,结合人工智能方法如深度学习神经网络,建立车辆运行不确定实时工况预测模型;其次,研究以纹波脉动为生命周期指标,以加速时间为动力性能指标的双目标带约束优化策略,使模糊能源管理器满足超级电容SOC不等式约束条件和车辆运行能源需求等式约束条件;最后,研究特定工况的动力性需求和Pareto前端分布特性之间的关系,制定不确定工况自适应的模糊能量在线管理新规则,并进行仿真和试验验证。本项目旨在解决不确定工况下的PEMFC-SC混合能源管理实时优化控制问题,延长燃料电池生命周期,提高整车动力性能,为克服燃料电池的应用瓶颈提供一种新的思路和方法。
结项摘要
项目主要研究不确定工况下的燃料电池/超级电容能源管理优化控制策略,以降低燃料电池氢气消耗,延长使用寿命。所开展研究内容如下:(1)研究了加窗KPCA快速主元分析方法进行车辆运行信息的特征提取,并结合神经网络进行车辆不确定实时工况的识别。(2)研究了一种以燃料电池电流纹波脉动最小、燃料消耗最少为目标的模糊能源管理策略,采用GA进行双目标带约束优化求解,使模糊能源管理器满足超级电容SOC不等式约束条件和车辆运行能源需求等式约束条件;(3)研究了基于神经网络不确定路况识别的模糊能源在线管理新规则。(4)引入Q增强学习和CNN深度学习神经网络,以进一步增强自适应工况感知能力,有利于控制器的性能优化提高。(5)进行了仿真和实验验证,在一定程度上解决了不确定工况下的PEMFC-SC混合能源管理实时优化管理和控制。
项目成果
期刊论文数量(4)
专著数量(0)
科研奖励数量(0)
会议论文数量(6)
专利数量(5)
Fuzzy Optimal Energy Management for Fuel Cell and Supercapacitor Systems Using Neural Network Based Driving Pattern Recognition
使用基于神经网络的驾驶模式识别的燃料电池和超级电容器系统的模糊最优能量管理
- DOI:10.1109/tfuzz.2018.2856086
- 发表时间:2019-01
- 期刊:IEEE TRANSACTIONS ON FUZZY SYSTEMS
- 影响因子:11.9
- 作者:Zhang Ridong;Tao Jili;Zhou Huiyu
- 通讯作者:Zhou Huiyu
Improved linear quadratic and proportional control system for improved liquid level system regulation in a coke fractionation tower
改进的线性二次和比例控制系统,用于改进焦炭分馏塔中的液位系统调节
- DOI:10.1016/j.isatra.2017.04.011
- 发表时间:2017
- 期刊:ISA Transactions
- 影响因子:7.3
- 作者:Tao Jili;Fan Qinru;Ma Longhua
- 通讯作者:Ma Longhua
CNN and KPCA-Based Automated Feature Extraction for Real Time Driving Pattern Recognition
基于 CNN 和 KPCA 的自动特征提取,用于实时驾驶模式识别
- DOI:10.1109/access.2019.2938768
- 发表时间:2019-09
- 期刊:IEEE Access
- 影响因子:3.9
- 作者:Xie Liang;Tao Jili;Zhou Huiyu
- 通讯作者:Zhou Huiyu
数据更新时间:{{ journalArticles.updateTime }}
{{
item.title }}
{{ item.translation_title }}
- DOI:{{ item.doi || "--"}}
- 发表时间:{{ item.publish_year || "--" }}
- 期刊:{{ item.journal_name }}
- 影响因子:{{ item.factor || "--"}}
- 作者:{{ item.authors }}
- 通讯作者:{{ item.author }}
数据更新时间:{{ journalArticles.updateTime }}
{{ item.title }}
- 作者:{{ item.authors }}
数据更新时间:{{ monograph.updateTime }}
{{ item.title }}
- 作者:{{ item.authors }}
数据更新时间:{{ sciAawards.updateTime }}
{{ item.title }}
- 作者:{{ item.authors }}
数据更新时间:{{ conferencePapers.updateTime }}
{{ item.title }}
- 作者:{{ item.authors }}
数据更新时间:{{ patent.updateTime }}
其他文献
其他文献
{{
item.title }}
{{ item.translation_title }}
- DOI:{{ item.doi || "--" }}
- 发表时间:{{ item.publish_year || "--"}}
- 期刊:{{ item.journal_name }}
- 影响因子:{{ item.factor || "--" }}
- 作者:{{ item.authors }}
- 通讯作者:{{ item.author }}
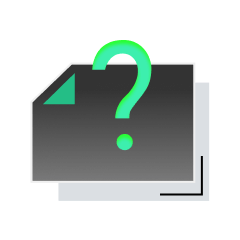
内容获取失败,请点击重试
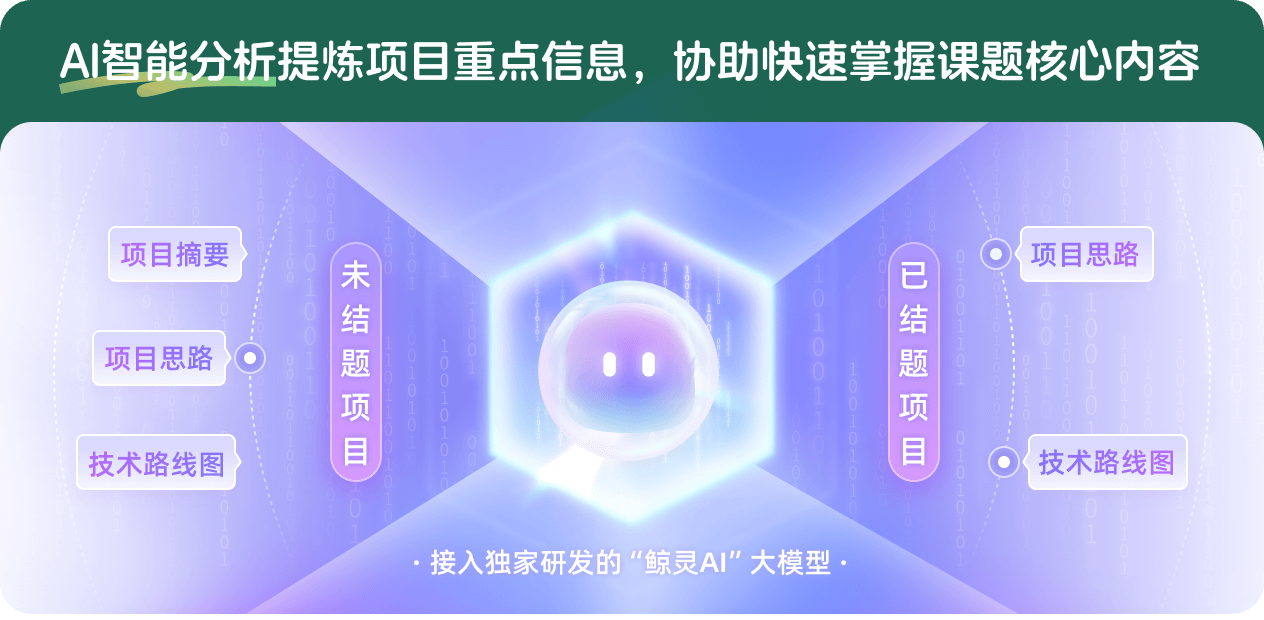
查看分析示例
此项目为已结题,我已根据课题信息分析并撰写以下内容,帮您拓宽课题思路:
AI项目摘要
AI项目思路
AI技术路线图
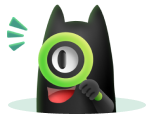
请为本次AI项目解读的内容对您的实用性打分
非常不实用
非常实用
1
2
3
4
5
6
7
8
9
10
您认为此功能如何分析更能满足您的需求,请填写您的反馈:
相似国自然基金
{{ item.name }}
- 批准号:{{ item.ratify_no }}
- 批准年份:{{ item.approval_year }}
- 资助金额:{{ item.support_num }}
- 项目类别:{{ item.project_type }}
相似海外基金
{{
item.name }}
{{ item.translate_name }}
- 批准号:{{ item.ratify_no }}
- 财政年份:{{ item.approval_year }}
- 资助金额:{{ item.support_num }}
- 项目类别:{{ item.project_type }}