面向糖尿病电子病历大数据的可解释性时序信息挖掘研究
项目介绍
AI项目解读
基本信息
- 批准号:61802149
- 项目类别:青年科学基金项目
- 资助金额:21.0万
- 负责人:
- 依托单位:
- 学科分类:F0213.生物信息计算与数字健康
- 结题年份:2021
- 批准年份:2018
- 项目状态:已结题
- 起止时间:2019-01-01 至2021-12-31
- 项目参与者:张享周; 刘雪彦; 陈蔚琦; 罗启超; 刘康; 胡申玲; 舒昕宇; 马芳婷;
- 关键词:
项目摘要
The data mining of interpretable temporal information from massive diabetic electronic health records (EHR) is a key step to transform data into knowledge, and also provides an important basis for further individualized intelligent management of diabetes. However, different from the traditional time series analysis model, due to the uniqueness of the trajectory of each diabetic patient, the high-dimensional and sparse diabetic EHR time-series data presents a complex characteristic of disorder. To solve these problems, based on the previous works and the massive diabetic EHR data, this project mainly focuses on the problems of the interpretable temporal information mining, including: first, in order to alleviate the high-dimensionality and redundancy problems of diabetic EHR data, we propose a stable screening ensemble mechanism for risk importance of sequential aggregation features; secondly, based on the matrix information of patient temporal portrait, the common pattern and individual pattern will be explored by nonnegative matrix factorization and optimization model; finally, through the vectorization and graphical representation of the temporal matrix information, machine learning and deep learning methods will be used to predict and verify the diabetic temporal pattern and complications. This project will promote the intelligent level of diabetic management and lay a key technical foundation for further intelligent and personalized medical diagnosis and treatment.
从海量糖尿病电子病历数据中进行可解释性时序信息挖掘研究是将数据转化为知识的关键步骤,也为进一步的糖尿病个性化智能管理提供重要依据。但不同于传统时间序列分析模型,由于每个糖尿病患者轨迹的唯一性,高维稀疏的糖尿病电子病历时序数据呈现出“无序”的复杂特点。针对这些问题,在已有工作的基础上,本项目以糖尿病电子病历的海量数据为研究对象,重点研究可解释性时序信息挖掘问题,具体包括:首先,研究时序聚合特征信息风险重要性的稳定筛选集成机制,从而缓解电子病历数据的高维和冗余问题;其次,针对“患者时序画像”矩阵信息,利用非负矩阵分解和最优化模型求解等方法挖掘“公共时序模式”和“个性化时序模式”;最后,通过时序矩阵信息的向量化和图像化表示,利用机器学习和深度学习方法对糖尿病并发症进行预测验证。本项目有望推进糖尿病智能健康管理的技术和水平,为进一步的医疗健康智能化知识推理和个性化健康诊疗奠定关键技术基础。
结项摘要
从海量电子病历数据中进行可解释信息挖掘研究是将数据转化为知识的关键步骤,也为进一步的疾病个性化智能管理提供重要依据。但不同于传统时间序列分析模型,由于每个患者轨迹的唯一性,高维与稀疏的电子病历时序数据呈现出“无序”的复杂特点。首先,针对当前对电子病历特征主要采用简单过滤式特征选择以及筛选的最优特征子集的不稳定等问题,项目构建了稳定的特征筛选集成机制,并详细地分析了如何选取与数据样本大小相匹配的最优特征选择方法,以及通过构建数据层或方法层集成策略增强最优风险因子筛选的稳定性。其次,结合人工智能和专家先验知识,构建了一套挖掘潜在风险知识的模型框架,并从预测准确性、知识稳定性、与专家知识一致率等三个角度分析了该方法的有效性,利用该方法挖掘出年龄组之间的风险差异性和波动性,不仅提供了可解释性知识挖掘结果,而且为探索潜在新的风险知识并增强个性化解释提供了可能。最后,利用机器学习和深度学习方法构建了糖尿病肾病并发症的风险评估模型,有助于提高疾病的早期预测或预防,具有深远的临床指导意义。.项目组与暨南大学第一附属医院、南方医科大学第一附属医院(南方医院)的医生就患者的临床数据知识挖掘及辅助预测开展了深入合作与交流,顺利完成预期任务,在国际核心学术期刊(SCI收录)上已发表高水平论文5篇,二审阶段论文2篇,参加多次国际与国内学术会议。本课题按照申请书设定的技术路线与年度计划有序进行。本课题研究成果对患者疾病预测、患者群体风险差异性、和潜在风险知识挖掘等方面的研究提供新的解决思路和方法,不仅具有理论意义,而且具有广阔应用前景。
项目成果
期刊论文数量(5)
专著数量(0)
科研奖励数量(0)
会议论文数量(0)
专利数量(0)
Feature Ranking in Predictive Models for Hospital-Acquired Acute Kidney Injury.
医院获得性急性肾损伤预测模型中的特征排名
- DOI:10.1038/s41598-018-35487-0
- 发表时间:2018-11-23
- 期刊:Scientific reports
- 影响因子:4.6
- 作者:Wu L;Hu Y;Liu X;Zhang X;Chen W;Yu ASL;Kellum JA;Waitman LR;Liu M
- 通讯作者:Liu M
Development of a knowledge mining approach to uncover heterogeneous risk predictors of acute kidney injury across age groups.
开发知识挖掘方法来揭示不同年龄组急性肾损伤的异质风险预测因素
- DOI:10.1016/j.ijmedinf.2021.104661
- 发表时间:2021-12-09
- 期刊:INTERNATIONAL JOURNAL OF MEDICAL INFORMATICS
- 影响因子:4.9
- 作者:Wu, Lijuan;Hu, Yong;Zhang, Xiangzhou;Zhang, Jia;Liu, Mei
- 通讯作者:Liu, Mei
Which risk predictors are more likely to indicate severe AKI in hospitalized patients?
哪些风险预测因子更有可能表明住院患者出现严重 AKI?
- DOI:10.1016/j.ijmedinf.2020.104270
- 发表时间:2020-11
- 期刊:International journal of medical informatics
- 影响因子:4.9
- 作者:Wu L;Hu Y;Yuan B;Zhang X;Chen W;Liu K;Liu M
- 通讯作者:Liu M
数据更新时间:{{ journalArticles.updateTime }}
{{
item.title }}
{{ item.translation_title }}
- DOI:{{ item.doi || "--"}}
- 发表时间:{{ item.publish_year || "--" }}
- 期刊:{{ item.journal_name }}
- 影响因子:{{ item.factor || "--"}}
- 作者:{{ item.authors }}
- 通讯作者:{{ item.author }}
数据更新时间:{{ journalArticles.updateTime }}
{{ item.title }}
- 作者:{{ item.authors }}
数据更新时间:{{ monograph.updateTime }}
{{ item.title }}
- 作者:{{ item.authors }}
数据更新时间:{{ sciAawards.updateTime }}
{{ item.title }}
- 作者:{{ item.authors }}
数据更新时间:{{ conferencePapers.updateTime }}
{{ item.title }}
- 作者:{{ item.authors }}
数据更新时间:{{ patent.updateTime }}
其他文献
其他文献
{{
item.title }}
{{ item.translation_title }}
- DOI:{{ item.doi || "--" }}
- 发表时间:{{ item.publish_year || "--"}}
- 期刊:{{ item.journal_name }}
- 影响因子:{{ item.factor || "--" }}
- 作者:{{ item.authors }}
- 通讯作者:{{ item.author }}
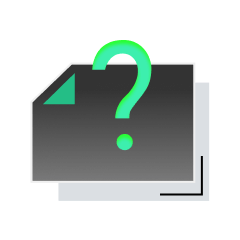
内容获取失败,请点击重试
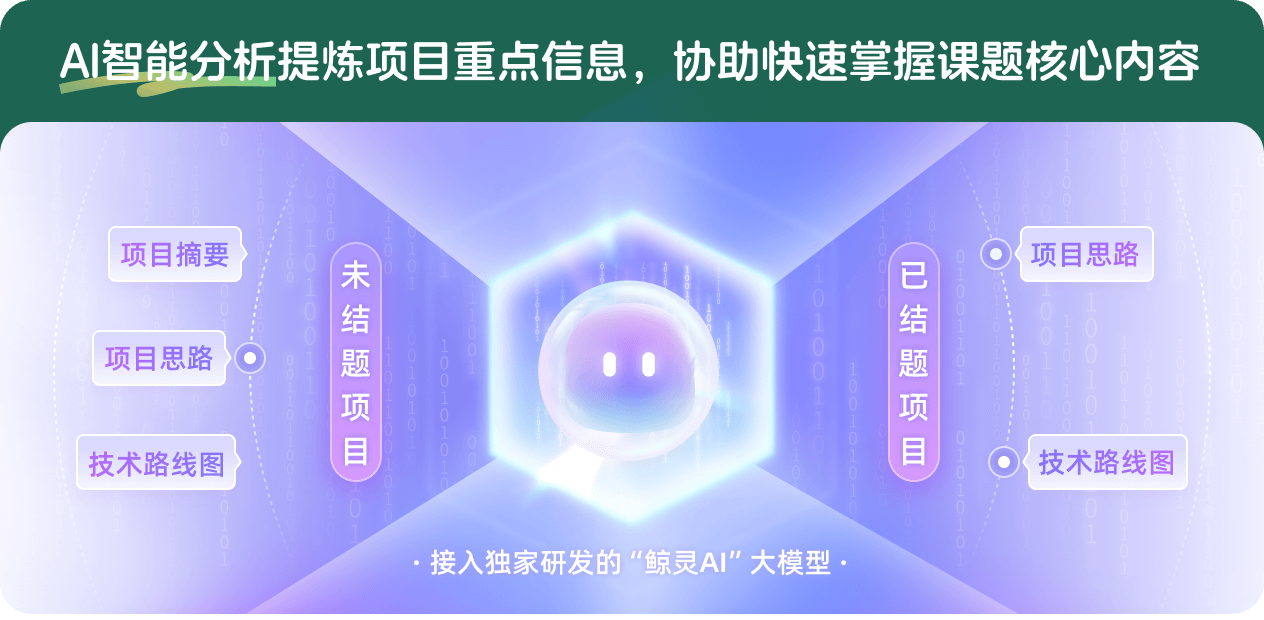
查看分析示例
此项目为已结题,我已根据课题信息分析并撰写以下内容,帮您拓宽课题思路:
AI项目摘要
AI项目思路
AI技术路线图
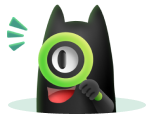
请为本次AI项目解读的内容对您的实用性打分
非常不实用
非常实用
1
2
3
4
5
6
7
8
9
10
您认为此功能如何分析更能满足您的需求,请填写您的反馈:
相似国自然基金
{{ item.name }}
- 批准号:{{ item.ratify_no }}
- 批准年份:{{ item.approval_year }}
- 资助金额:{{ item.support_num }}
- 项目类别:{{ item.project_type }}
相似海外基金
{{
item.name }}
{{ item.translate_name }}
- 批准号:{{ item.ratify_no }}
- 财政年份:{{ item.approval_year }}
- 资助金额:{{ item.support_num }}
- 项目类别:{{ item.project_type }}