黄芪当归合剂对慢性肾损害进展的保护作用相关基因研究
项目介绍
AI项目解读
基本信息
- 批准号:39970929
- 项目类别:面上项目
- 资助金额:14.0万
- 负责人:
- 依托单位:
- 学科分类:H3302.中西医结合临床基础
- 结题年份:2002
- 批准年份:1999
- 项目状态:已结题
- 起止时间:2000-01-01 至2002-12-31
- 项目参与者:李惊子; 管又飞; 余凌; 周安宁; 张宏; 郑欣; 侯平; 李文梅;
- 关键词:
项目摘要
在既往确证黄芪当归(A&A)防止肾脏硬化的基础上,本课题从中医阴阳平衡观点的角度探髌渥饔玫墓丶颍ㄉ系鞅;せ蚝?或抑制损害基因)。应用mRNA差异显示技术,并通过克隆、测序、筛库等获得A&A延缓慢性肾损害进展的作用相关基因全长;研究其在肾组织生怼⒉±碜刺卤泶锏那榭觯怀醪教剿髌涔δ堋?...
结项摘要
项目成果
期刊论文数量(0)
专著数量(0)
科研奖励数量(0)
会议论文数量(0)
专利数量(0)
数据更新时间:{{ journalArticles.updateTime }}
{{
item.title }}
{{ item.translation_title }}
- DOI:{{ item.doi || "--"}}
- 发表时间:{{ item.publish_year || "--" }}
- 期刊:{{ item.journal_name }}
- 影响因子:{{ item.factor || "--"}}
- 作者:{{ item.authors }}
- 通讯作者:{{ item.author }}
数据更新时间:{{ journalArticles.updateTime }}
{{ item.title }}
- 作者:{{ item.authors }}
数据更新时间:{{ monograph.updateTime }}
{{ item.title }}
- 作者:{{ item.authors }}
数据更新时间:{{ sciAawards.updateTime }}
{{ item.title }}
- 作者:{{ item.authors }}
数据更新时间:{{ conferencePapers.updateTime }}
{{ item.title }}
- 作者:{{ item.authors }}
数据更新时间:{{ patent.updateTime }}
其他文献
Thermal Kinetics of Coal Spontaneous Combustion Based on Multiphase Fully Coupled Fluid–Mechanical Porous Media Model
基于多相全耦合流体机械多孔介质模型的煤自燃热动力学
- DOI:10.1007/s11053-022-10101-3
- 发表时间:2022-07
- 期刊:Springer Nature
- 影响因子:--
- 作者:王海燕;黎经雷;樊程;王磊;陈晓
- 通讯作者:陈晓
Pyrolysis and combustion characteristics and reaction kinetics of carbon fiber/epoxy composites
碳纤维/环氧树脂复合材料的热解燃烧特性及反应动力学
- DOI:10.1063/1.5128460
- 发表时间:2019-12
- 期刊:AIP Advances
- 影响因子:1.6
- 作者:王海燕;张雷
- 通讯作者:张雷
5-氨基酮戊酸光动力疗法对人卵巢癌细胞的杀伤作用及对HSP70和HIF-1α表达的影响
- DOI:--
- 发表时间:2011
- 期刊:医学与哲学(临床决策论坛版)
- 影响因子:--
- 作者:姜侃;张友忠;焦慧;王海燕;JIANG Kan,ZHANG You-zhong,JIAO Hui,et al.Departmen
- 通讯作者:JIANG Kan,ZHANG You-zhong,JIAO Hui,et al.Departmen
基于三角模型的城市土地可持续利用评价——以江苏省无锡市为例
- DOI:--
- 发表时间:--
- 期刊:经济地理
- 影响因子:--
- 作者:朱明;王海燕;赵艳;张健;陕永杰;濮励杰
- 通讯作者:濮励杰
香椿属中三萜类化学成分及生物活性研究进展
- DOI:--
- 发表时间:2013
- 期刊:现代生物医学进展
- 影响因子:--
- 作者:王海燕;王荔;骆春丽;岑娟
- 通讯作者:岑娟
其他文献
{{
item.title }}
{{ item.translation_title }}
- DOI:{{ item.doi || "--" }}
- 发表时间:{{ item.publish_year || "--"}}
- 期刊:{{ item.journal_name }}
- 影响因子:{{ item.factor || "--" }}
- 作者:{{ item.authors }}
- 通讯作者:{{ item.author }}
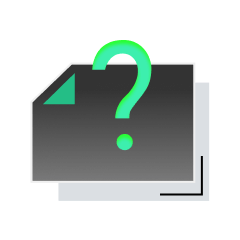
内容获取失败,请点击重试
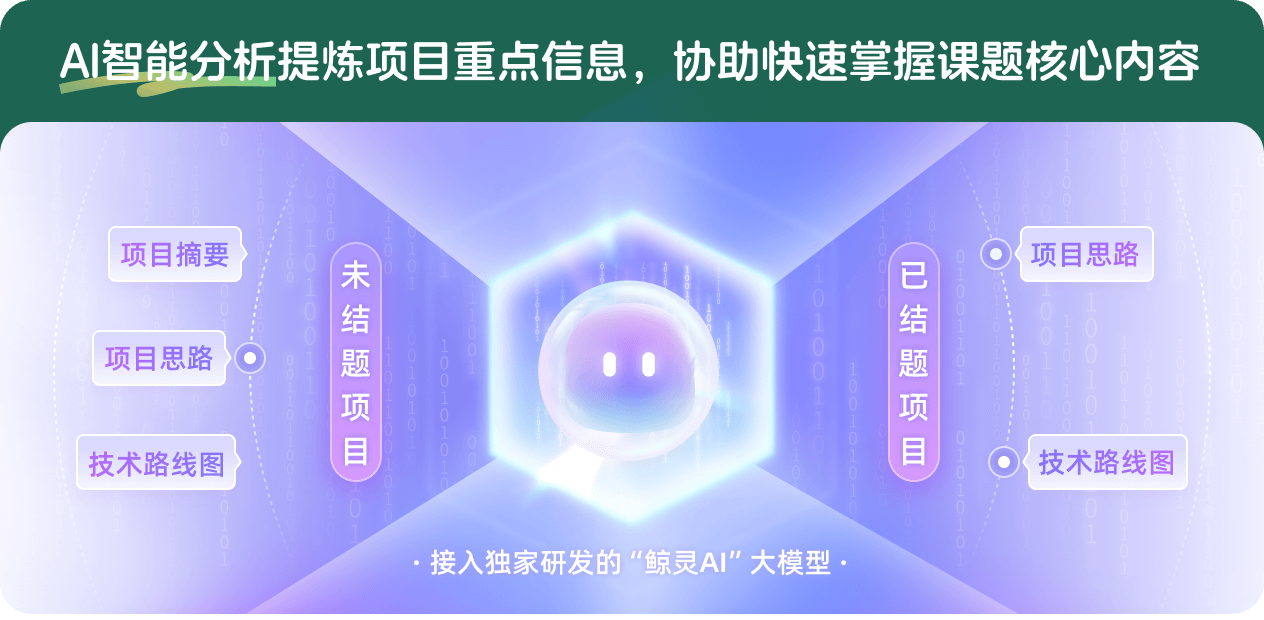
查看分析示例
此项目为已结题,我已根据课题信息分析并撰写以下内容,帮您拓宽课题思路:
AI项目摘要
AI项目思路
AI技术路线图
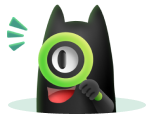
请为本次AI项目解读的内容对您的实用性打分
非常不实用
非常实用
1
2
3
4
5
6
7
8
9
10
您认为此功能如何分析更能满足您的需求,请填写您的反馈:
王海燕的其他基金
透明质酸在子宫内膜微环境的表达及对胚胎发育和粘附的影响
- 批准号:30671971
- 批准年份:2006
- 资助金额:28.0 万元
- 项目类别:面上项目
G1期蛋白在肾小球系膜细胞炎症增殖中调控作用的研究
- 批准号:39670350
- 批准年份:1996
- 资助金额:9.5 万元
- 项目类别:面上项目
免疫介导炎症性疾病发生机理的研究
- 批准号:39130100
- 批准年份:1991
- 资助金额:45.0 万元
- 项目类别:重点项目
白细胞介素1(IL1)在IgA肾病发病过程中的作用
- 批准号:38870425
- 批准年份:1988
- 资助金额:3.0 万元
- 项目类别:面上项目
相似国自然基金
{{ item.name }}
- 批准号:{{ item.ratify_no }}
- 批准年份:{{ item.approval_year }}
- 资助金额:{{ item.support_num }}
- 项目类别:{{ item.project_type }}
相似海外基金
{{
item.name }}
{{ item.translate_name }}
- 批准号:{{ item.ratify_no }}
- 财政年份:{{ item.approval_year }}
- 资助金额:{{ item.support_num }}
- 项目类别:{{ item.project_type }}