对称性破缺对介观尺度下氢键流体相平衡的调控研究
项目介绍
AI项目解读
基本信息
- 批准号:21306034
- 项目类别:青年科学基金项目
- 资助金额:25.0万
- 负责人:
- 依托单位:
- 学科分类:B0801.化工热力学
- 结题年份:2016
- 批准年份:2013
- 项目状态:已结题
- 起止时间:2014-01-01 至2016-12-31
- 项目参与者:李江涛; 李志云; 宋洪赞; 乔严君; 魏慧敏;
- 关键词:
项目摘要
Hydrogen bonding fluid is a typical kind of inhomogeneous and multi-scale system in which exists the interplay between the geometrical phase and thermodynamic phase. Such system usually displays very rich phase behaviors because various clusters of fluid molecules can be formed by hydrogen bonds. In this proposal, the aggregated structure, phase equilibria and some fundamental properties of hydrogen bonding fluid in the case of broken symmetry are investigated in terms of density functional theory for classical fluids. In detail, the broken symmetries under study are mainly induced by the confined microcavity, external field and the dipole interaction between molecules, which would be the focus of the present project. The main problems to be discussed in this project are given as follows. First, the aggregated structure, phase equilibria and some fundamental physical properties of hydrogen bonding fluid in the case of broken symmetry mentioned above. Second, the relationship between the geometrical phases and thermodynamic phases, where the geometrical phases are referred to the sol phase and gel phase, respectively. Third, the depletion potential between Janus colloid particles immersed in a hydrogen bonding fluid. The project aims to reveal the influences of broken symmetry on the aggregated structures and phase behavior of hydrogen bonding fluid and further to deepen the understanding of phase behavior of hydrogen bonding fluid. It is expected to provide useful clues for the practical applications of hydrogen bonding fluid in fields of chemistry and chemical engineering, physical and biophysics and material science.
氢键流体是一种典型的多尺度、非均相体系, 其中的热力学相和几何相相互耦合, 呈现出丰富的相态结构. 本项目拟利用经典流体的密度泛函理论对微腔中氢键流体在对称性发生破缺情况下的聚集态结构、相平衡及其相关物理化学特征予以研究. 研究中的对称性破缺主要包括因空间、外场以及分子偶极相互作用所致的对称性破缺. 研究内容主要涵盖: (1). 氢键流体在对称性破缺情况下的聚集态结构、相平衡行为及其相关物理化学特征; (2). 对称性破缺条件下, 氢键流体的几何相与热力学相之间的相互关系; (3). 氢键流体中Janus胶体粒子间的耗尽势. 研究旨在探索对称性破缺对典型微腔中氢键流体物理化学特征的影响, 从而深化有关氢键流体相态结构和相行为的认知, 为研究化学、化工、物理、生物和材料科学等领域中氢键流体的重要作用提供可能的理论线索.
结项摘要
氢键流体是一种典型的多尺度、非均相体系,其热力学相和几何相相互耦合,在受限条件尤其是对称性破缺情况下呈现出更为丰富的相态结构。本项目利用经典流体的密度泛函理论对受限于微腔中氢键流体在对称性破缺条件下的聚集态结构、相平衡及其相关物理化学特征予以研究,并针对软物质科学领域中补丁粒子的聚集态结构进行了深入探讨。.目前已顺利完成了计划书中的各项内容,并适当增加了相关体系的Monte Carlo模拟研究。研究内容主要涵盖:引入空间及外场所致的对称性破缺作用,阐明对称性破缺对氢键流体及其他几类典型流体的聚集态结构、相平衡及相关物理化学特征的影响;考察微腔表面对氢键流体相行为的调控作用,讨论表面作用对微腔中氢键流体相态结构的调控机制;揭示介观尺度下对称性破缺特别是半透膜存在下氢键流体的渗透压问题,为研究生物、化学和物理领域中的氢键作用提供可能的理论线索;针对软物质科学领域中的补丁粒子(主要包括Patchy粒子和Janus粒子)的聚集态结构进行了研究,同时为后续研究内容作出了相应的规划和设计;研究了氢键流体的物理凝胶化现象,探讨了聚集过程中体系自身特征与外部热力学条件之间的联系;作为拓展研究,课题组开展了环化效应的Monte Carlo模拟研究,提出了一种新的Monte Carlo模拟方案并在此方案的基础上成功地自主开发了模拟程序,用于相关的研究之中。.课题旨在深化有关氢键流体相态结构和相行为的认知,为进一步探索生物、材料和物理等领域中氢键作用、介观尺度下的渗透压问题以及多孔材料的气体吸附等方面提供理论依据。项目执行期间共发表SCI检索论文8篇(含接收发表2篇),中文核心期刊3篇,国内会议分组报告1人次。在基金资助下,共培养硕士研究生5名。
项目成果
期刊论文数量(11)
专著数量(0)
科研奖励数量(0)
会议论文数量(5)
专利数量(0)
Phase equilibria of hydrogen bonding fluid in a slit pore with broken symmetry
对称破缺狭缝孔内氢键流体的相平衡
- DOI:10.1063/1674-0068/28/cjcp1501001
- 发表时间:2015
- 期刊:Chinese Journal of Chemical Physics
- 影响因子:1
- 作者:Liu Xiao-Yu;Li Jiang-Tao;Gu Fang;Wang Hai-Jun
- 通讯作者:Wang Hai-Jun
三元自缩合乙烯基聚合体系回转半径的Monte Carol模拟
- DOI:--
- 发表时间:2014
- 期刊:河北大学学报(自然科学版)
- 影响因子:--
- 作者:洪晓钟;顾芳;巴信武
- 通讯作者:巴信武
对称性破缺狭缝中Lennard-Jones流体的相平衡
- DOI:--
- 发表时间:2015
- 期刊:河北大学学报(自然科学版)
- 影响因子:--
- 作者:顾芳;刘晓雨;张泽坤;张雅楠
- 通讯作者:张雅楠
ABg型超支化聚合反应中的环化效应
- DOI:--
- 发表时间:2015
- 期刊:高等学校化学学报
- 影响因子:--
- 作者:常沛杨;王云明;顾芳;王海军
- 通讯作者:王海军
Structure of solution of colloid and hydrogen bonding fluid near a semipermeable membrane
半透膜附近胶体和氢键流体溶液的结构
- DOI:10.1007/s40242-017-6398-1
- 发表时间:2017
- 期刊:Chemical Research in Chinese Universities
- 影响因子:3.1
- 作者:Zhao Meng;Chen Han-Fei;Gu Fang;Wang Hai-Jun
- 通讯作者:Wang Hai-Jun
数据更新时间:{{ journalArticles.updateTime }}
{{
item.title }}
{{ item.translation_title }}
- DOI:{{ item.doi || "--"}}
- 发表时间:{{ item.publish_year || "--" }}
- 期刊:{{ item.journal_name }}
- 影响因子:{{ item.factor || "--"}}
- 作者:{{ item.authors }}
- 通讯作者:{{ item.author }}
数据更新时间:{{ journalArticles.updateTime }}
{{ item.title }}
- 作者:{{ item.authors }}
数据更新时间:{{ monograph.updateTime }}
{{ item.title }}
- 作者:{{ item.authors }}
数据更新时间:{{ sciAawards.updateTime }}
{{ item.title }}
- 作者:{{ item.authors }}
数据更新时间:{{ conferencePapers.updateTime }}
{{ item.title }}
- 作者:{{ item.authors }}
数据更新时间:{{ patent.updateTime }}
其他文献
乳源免疫调节肽提高DDP对卵巢癌细胞的影响
- DOI:10.19405/j.cnki.issn1000-1492.2016.10.010
- 发表时间:2016
- 期刊:安徽医科大学学报
- 影响因子:--
- 作者:周娟;刘琛;顾芳;赵梦静;杨雪;王晶;秦宜德
- 通讯作者:秦宜德
Gd_2Co_2Al电子结构和磁性的第一性原理研究
- DOI:--
- 发表时间:--
- 期刊:物理学报
- 影响因子:--
- 作者:顾芳;刘甦;杨丽娟;刘楣;张加宏
- 通讯作者:张加宏
激光衍射法测量粒子群窄分布反演优化算法
- DOI:--
- 发表时间:2012
- 期刊:南京信息工程大学学报(自然科学版)
- 影响因子:--
- 作者:顾芳;张加宏;刘清惓;李敏
- 通讯作者:李敏
乳源免疫调节肽对人卵巢癌SKOV_3细胞生长及凋亡的影响
- DOI:--
- 发表时间:--
- 期刊:肿瘤
- 影响因子:--
- 作者:李素萍;郭晓婕;贾晓益;顾芳;秦宜德;刘忠
- 通讯作者:刘忠
气溶胶质量浓度测量系统的设计与实现
- DOI:--
- 发表时间:2017
- 期刊:电子器件
- 影响因子:--
- 作者:沈雷;张加宏;顾芳;冒晓莉;刘毅
- 通讯作者:刘毅
其他文献
{{
item.title }}
{{ item.translation_title }}
- DOI:{{ item.doi || "--" }}
- 发表时间:{{ item.publish_year || "--"}}
- 期刊:{{ item.journal_name }}
- 影响因子:{{ item.factor || "--" }}
- 作者:{{ item.authors }}
- 通讯作者:{{ item.author }}
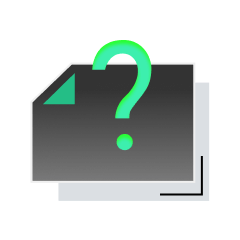
内容获取失败,请点击重试
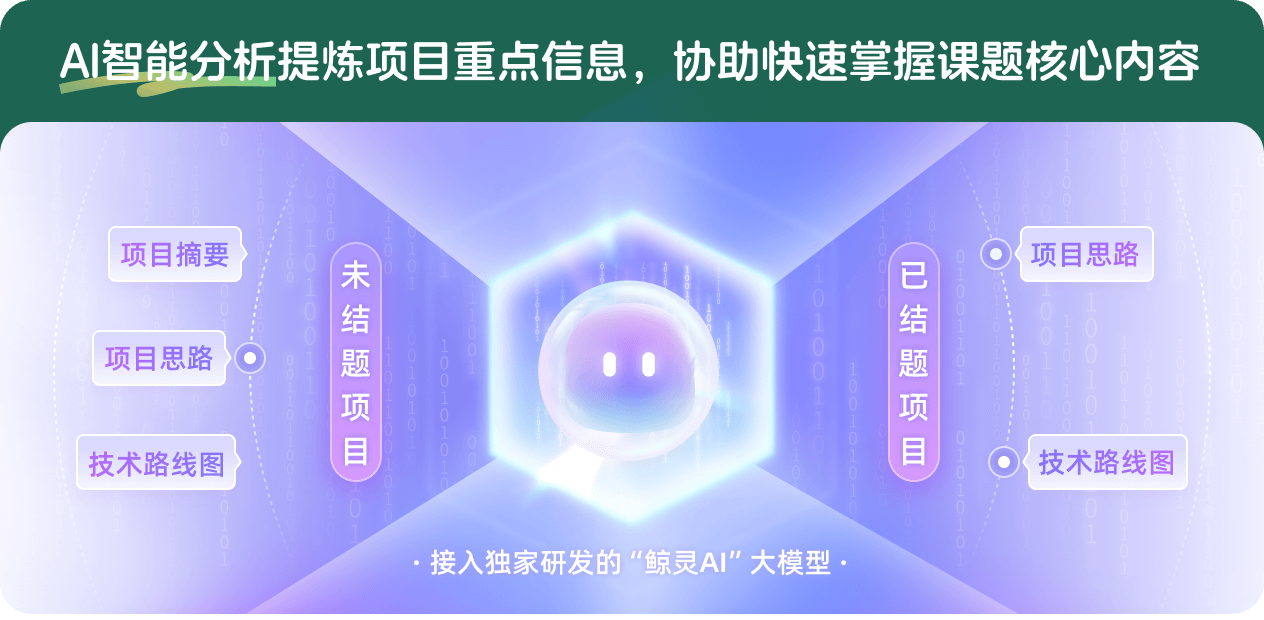
查看分析示例
此项目为已结题,我已根据课题信息分析并撰写以下内容,帮您拓宽课题思路:
AI项目摘要
AI项目思路
AI技术路线图
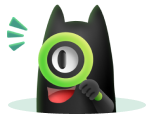
请为本次AI项目解读的内容对您的实用性打分
非常不实用
非常实用
1
2
3
4
5
6
7
8
9
10
您认为此功能如何分析更能满足您的需求,请填写您的反馈:
相似国自然基金
{{ item.name }}
- 批准号:{{ item.ratify_no }}
- 批准年份:{{ item.approval_year }}
- 资助金额:{{ item.support_num }}
- 项目类别:{{ item.project_type }}
相似海外基金
{{
item.name }}
{{ item.translate_name }}
- 批准号:{{ item.ratify_no }}
- 财政年份:{{ item.approval_year }}
- 资助金额:{{ item.support_num }}
- 项目类别:{{ item.project_type }}