基于相似参照运算模型的容积率值域生成机制与方法
项目介绍
AI项目解读
基本信息
- 批准号:51578126
- 项目类别:面上项目
- 资助金额:62.0万
- 负责人:
- 依托单位:
- 学科分类:E0801.建筑学
- 结题年份:2019
- 批准年份:2015
- 项目状态:已结题
- 起止时间:2016-01-01 至2019-12-31
- 项目参与者:高源; 朱渊; 龚恺; 华好; 邢佳林; 李京津; 兰文龙; 林岩; 孔斌;
- 关键词:
项目摘要
Floor area ratio (FAR) is the one of the core indexes in urban land regulation and planning design administration. How to derive FAR interval reasonably is the necessary and long-term key problem in China’s urbanization. FAR mainly complies with the master planning and empirical judgment in practice, and the existing researches emphasis on the model establishment of economy, environment, function, policy on land itself, while ignore the interaction between lands. Actually, the decision-making of each land’s FAR always refers to the lands that have the similar conditions consciously or unconsciously, so that the city becomes a complex system composed of lands that refer to and affect each other. Therefore, as the necessary complement to the existing researches, the applicant tries to discover the spontaneous interactive mechanism based on the similarity reference relationship between urban lands. By the abstraction, simulation, improvement and intervention of the mechanism, the reproducible computational model of similarity reference can be established, which may lead to the credible generating method of FAR and enhance the impersonality, justice and transparency of FAR decision-making.
容积率是用地调控和规划设计管理的核心指标之一。如何确定容积率的合理值域是我国城市化进程亟待解决并将长期面对的要害问题。在实践中,容积率主要依据上位规划的指标细分和经验判断,而现有相关研究重在用地本身基于经济、环境、功能、政策等方面的模型建构,而忽视了用地之间的相互影响。其实每一用地的容积率决策,总会有意或无意地反复参照用地条件类似的相关用地状况,使城市成为各用地彼此参照、相互影响的复杂系统。因此,作为对该领域研究的必要补充,申请人试图揭示城市用地之间基于相似参照关系的自发互动机制,并通过对该机制的抽象、模拟、改进和介入,建立可复制的相似参照运算模型,继而通过整合既有相关研究确定的容积率区间,形成切实可行的容积率生成方法,以提高容积率决策的客观、公正和透明度。
结项摘要
通常的容积率判定主要来自相对个人化的主观经验和相对宽泛的固定技术规范,或者依赖经济、日照、交通等单一功能向度的理想模型,而没有认识到容积率决策背后的城市空间形态整体逻辑,缺乏具有科学依据的系统技术支持。本研究通过阐明城市空间形态建构机理,综合考虑用地自发参照关系、既往开发经验和城市发展的复杂性,在城市整体空间形态的系统优化基础上判定容积率值域。研究认为,相似参照是建成环境背后的一个普遍逻辑,是城市用地之间互动的内在动态机制,而对相似参照行为的模拟则是理解城市空间形态的一把钥匙。本研究通过问卷调查和既往批出用地的数据分析,共同证明相似参照原则的普遍存在和认同。研究将容积率与高度控制作为一个整体,纳入城市空间形态的理解中,形成针对单一用地、针对特定类型用地、针对全城或较大片区的较为完整的多层次开发强度决策方法体系。尤其通过独创的概率参照算法,体现出容纳实际偶然性的决策理性,超越了通常容积率决策“区间”或“值域”的概念。同时,课题提出了适用于本运算模型的用地因子评价及其权重设置的方法。课题研发的软件将外部介入引导和城市自发生长有机结合,并应用于7个有影响力的大尺度城市项目和2个具体地块决策项目,并获得广泛公众影响。总体来说,本课题取得了“基础研究-论文发表-模型与软件-专利技术-实际应用-评价获奖-宣传推广”全链条系统性成果,超出研究预期。
项目成果
期刊论文数量(6)
专著数量(0)
科研奖励数量(6)
会议论文数量(3)
专利数量(4)
建筑遗产信息共享与价值评估在线平台初探
- DOI:--
- 发表时间:2019
- 期刊:室内设计与装修
- 影响因子:--
- 作者:张愚
- 通讯作者:张愚
城市高度形态的相似参照逻辑与模拟
- DOI:--
- 发表时间:2016
- 期刊:新建筑
- 影响因子:--
- 作者:张愚;王建国
- 通讯作者:王建国
Optimization of large-scale urban spatial form based on decision support system for land development intensity
优化
- DOI:10.1360/n092014-00436
- 发表时间:2016
- 期刊:Zhongguo Kexue Jishu Kexue/Scientia Sinica Technologica
- 影响因子:--
- 作者:Wang Jianguo;Zhang Yu
- 通讯作者:Zhang Yu
基于经验类比的轨道一般站容积率量化研究
- DOI:--
- 发表时间:2019
- 期刊:城市规划
- 影响因子:--
- 作者:周梦茹;高源;胡智行;吴晓
- 通讯作者:吴晓
建筑师
- DOI:--
- 发表时间:2020
- 期刊:游戏的人:从游戏广场到“新巴比伦”
- 影响因子:--
- 作者:朱渊;孙磊磊
- 通讯作者:孙磊磊
数据更新时间:{{ journalArticles.updateTime }}
{{
item.title }}
{{ item.translation_title }}
- DOI:{{ item.doi || "--"}}
- 发表时间:{{ item.publish_year || "--" }}
- 期刊:{{ item.journal_name }}
- 影响因子:{{ item.factor || "--"}}
- 作者:{{ item.authors }}
- 通讯作者:{{ item.author }}
数据更新时间:{{ journalArticles.updateTime }}
{{ item.title }}
- 作者:{{ item.authors }}
数据更新时间:{{ monograph.updateTime }}
{{ item.title }}
- 作者:{{ item.authors }}
数据更新时间:{{ sciAawards.updateTime }}
{{ item.title }}
- 作者:{{ item.authors }}
数据更新时间:{{ conferencePapers.updateTime }}
{{ item.title }}
- 作者:{{ item.authors }}
数据更新时间:{{ patent.updateTime }}
其他文献
城市历史空间的计算机视觉模拟研
- DOI:--
- 发表时间:--
- 期刊:建筑学报, 2004年 02期
- 影响因子:--
- 作者:张愚;诸葛净;陈薇
- 通讯作者:陈薇
CURE聚类方法及其在交通信息服务系统中的应用
- DOI:--
- 发表时间:--
- 期刊:科学技术与工程
- 影响因子:--
- 作者:张愚;翁小雄
- 通讯作者:翁小雄
span style=font-family:宋体;font-size:10.5pt;将人的皮肤成纤维细胞直接诱导为神经干细胞/span
- DOI:--
- 发表时间:2014
- 期刊:基础医学与临床
- 影响因子:--
- 作者:贾伟丽;李默;吴建宇;王淑艳;陈志国;张愚
- 通讯作者:张愚
A decision-making model of development intensity based on similarity relationship between land attributes intervened by urban design
城市设计干预下基于土地属性相似关系的开发强度决策模型
- DOI:10.1007/s11431-010-4029-x
- 发表时间:2010-07
- 期刊:Sci China E-Tech Sci
- 影响因子:--
- 作者:王建国;张愚;冯瀚
- 通讯作者:冯瀚
城市设计干预下基于用地属性相似关系的开发强度决策模型
- DOI:--
- 发表时间:--
- 期刊:中国科学 技术科学
- 影响因子:--
- 作者:王建国;张愚;冯翰
- 通讯作者:冯翰
其他文献
{{
item.title }}
{{ item.translation_title }}
- DOI:{{ item.doi || "--" }}
- 发表时间:{{ item.publish_year || "--"}}
- 期刊:{{ item.journal_name }}
- 影响因子:{{ item.factor || "--" }}
- 作者:{{ item.authors }}
- 通讯作者:{{ item.author }}
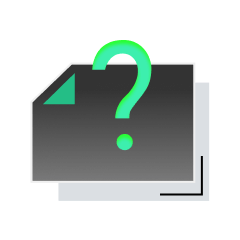
内容获取失败,请点击重试
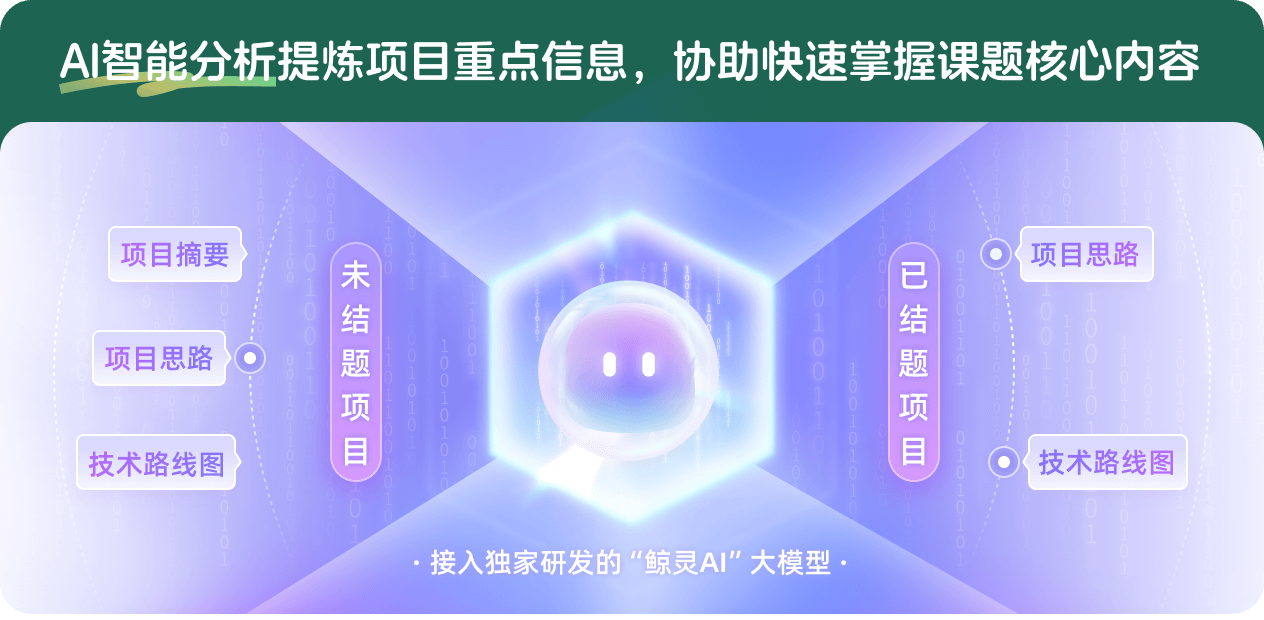
查看分析示例
此项目为已结题,我已根据课题信息分析并撰写以下内容,帮您拓宽课题思路:
AI项目摘要
AI项目思路
AI技术路线图
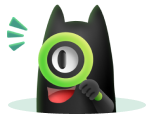
请为本次AI项目解读的内容对您的实用性打分
非常不实用
非常实用
1
2
3
4
5
6
7
8
9
10
您认为此功能如何分析更能满足您的需求,请填写您的反馈:
相似国自然基金
{{ item.name }}
- 批准号:{{ item.ratify_no }}
- 批准年份:{{ item.approval_year }}
- 资助金额:{{ item.support_num }}
- 项目类别:{{ item.project_type }}
相似海外基金
{{
item.name }}
{{ item.translate_name }}
- 批准号:{{ item.ratify_no }}
- 财政年份:{{ item.approval_year }}
- 资助金额:{{ item.support_num }}
- 项目类别:{{ item.project_type }}