可液化地基-地铁地下结构地震失效振动台实验与数值模拟
项目介绍
AI项目解读
基本信息
- 批准号:51508526
- 项目类别:青年科学基金项目
- 资助金额:23.0万
- 负责人:
- 依托单位:
- 学科分类:E0810.工程防灾
- 结题年份:2018
- 批准年份:2015
- 项目状态:已结题
- 起止时间:2016-01-01 至2018-12-31
- 项目参与者:刘爱文; 唐柏赞; 熊政辉; 王元德;
- 关键词:
项目摘要
Earthquake is one of the main causes of the underground subway structure disaster. Seismic failure mechanism and mode of underground subway structure which located in the poor geological condition has became the hotspot on the research field of geotechnical earthquake engineering. Current research has not yet formed the space-time evolution progress and the causes of catastrophe processing on most unfavorable structural component of underground subway structure. In this project, shaking-table tests on the seismic failure of liquefiable soil-underground subway structure will be carried out, moreover, the vision-based physical quantities testing technologies and distributed and flexible pore water pressure sensor array will be developed and applied in shaking-table test to record the disaster process of underground structure. Three-dimensional effective stress analysis parallel computing explicit finite element subroutine by mean of the VUMAT connector of software ABAQUS will be developed. On basis of the subroutine, the numerical simulations on the damage process of liquefaction soil-underground subway structure will be performed to validate and analyze the seismic failure mechanism and space-time evolution progress obtained by shaking-table tests. The project intended for the development and improvement advanced experimental technology and three dimensional nonlinear calculation method for engineering application, which can provide insight into the seismic performance and failure mechanism of liquefiable soil-underground subway structure and theoretical basis for anti-seismic design of underground structure.
地震是引起地铁地下结构灾害的最主要因素之一,不良地基条件下地铁地下结构地震破坏机理与模式已成为岩土地震工程研究的热点问题。已有研究尚不能有效描述地震动作用下液化地基-地铁地下结构地震失效的演化过程及空间最不利构件的灾变成因。本项目拟开展可液化地基-地铁地下结构振动台模型实验,研发面向振动台实验的基于机器视觉理论的非接触性物理量新型测试方法及分布式柔性孔压传感链,实现地下结构灾变过程的可视化与数据化;基于ABAQUS软件的VUMAT接口开发考虑地基液化的三维有效应力显式有限元并行计算模块,开展液化地基-地铁地下结构失效过程数值模拟,验证、分析振动台实验获取的液化地基-地铁地下结构地震损伤与失效的时空演化过程。本研究旨在发展和完善可液化地基-地下结构振动台实验技术与适宜的三维非线性数值计算方法,揭示液化地基上地铁地下结构地震破坏机理与模式,为可液化地基地铁地下结构抗震性能设计提供理论依据。
结项摘要
我国大型城市轨道交通地下结构建设处于蓬勃发展时期,建设场地条件越趋复杂,车站结构形式也复杂多样。近年地震灾害现象表明,地下结构在强地震作用下可能会出现严重的震害及次生灾害,城市大型地下结构工程的抗震安全性已成为倍受关注的社会问题之一。因此,研究不良地质条件下不规则地下结构的地震安全性具有重要的学术意义与工程应用价值。本文以不规则(上层五跨下层三跨)地铁地下车站结构和场地条件为原型,分别采用模拟地震振动台试验和数值模拟方法开展研究,探讨饱和砂土地基和软弱粘土地基中变截面地铁地下车站结构的地震反应特性及损伤机理。为满足振动台试验中多种类型物理量的有效测试,研发了分布式柔性孔压传感链技术,应用了阵列式位移计测试技术,并基于机器视觉研发的非接触性动态位移测试技术实现了地下结构灾变过程的可视化及数据化。研发的分布式柔性孔压传感链测试技术可以有效解决振动过程中传感器与土体惯性力不同而产生的自身摇摆、移位等问题。项目形成了地基土-地铁地下结构体系地震损伤与破坏时空演化过程的大型振动台试验成套试验流程及方法。对完成的地铁地下车站结构地震灾变机理振动台系列试验结果进行对比分析,包括地铁车站模型结构周围地基土的加速度反应规律、地表震陷特征,模型地基土与结构接触动土压力反应特性,变截面地铁车站模型结构的加速度、侧向位移和动应变的反应规律等,得出了不同场地中变截面地铁地下结构地震反应特性的差异性。基于有限元软件ABAQUS计算平台,建立了地基土-地下结构地震响应分析的技术流程,基于此开展了软弱场地中变截面地铁地下车站结构振动台试验的数值模拟,并与振动台模型试验结果进行了比较,验证了该数值分析方法的可行性和有效性。在此基础上,对复杂环境下足尺变截面地铁地下车站结构进行了数值模拟计算,给出了其地震反应特性。在项目的支持下,发表相关的SCI/EI检索论文8篇,申报专利4项,软件著作权3项;协助培养硕士生1名,博士生2名。
项目成果
期刊论文数量(8)
专著数量(0)
科研奖励数量(1)
会议论文数量(0)
专利数量(2)
振动台试验中小缩尺比模型材料试验研究
- DOI:10.13204/j.gyjz201612020
- 发表时间:2016
- 期刊:工业建筑
- 影响因子:--
- 作者:权登州;王毅红;马蓬渤;井彦林;陈苏
- 通讯作者:陈苏
黄土地区地铁车站地震反应的频域分析及空间效应
- DOI:--
- 发表时间:2016
- 期刊:振动与冲击
- 影响因子:--
- 作者:权登州;王毅红;马蓬渤;井彦林
- 通讯作者:井彦林
Burnout syndrome at the emergency service
基于视觉识别的地震地面位移测试方法
- DOI:10.1155/2019
- 发表时间:2006-01-01
- 期刊:Secondary Journal of Resuscitation and Emergency Medicine
- 影响因子:--
- 作者:Kalemoglu, M.
- 通讯作者:Kalemoglu, M.
Visual Identity-Based Earthquake Ground Displacement Testing Method
基于视觉识别的地震地面位移测试方法
- DOI:10.1155/2019/2585423
- 发表时间:2019-01
- 期刊:Shock and Vibration
- 影响因子:1.6
- 作者:Chen Su;Zhao Sicheng;Dai Zhijun;Li Xiaojun;Zhou Yue
- 通讯作者:Zhou Yue
近海域地震动的时频特征与工程特性
- DOI:--
- 发表时间:2018
- 期刊:振动与冲击
- 影响因子:--
- 作者:陈苏;周越;李小军;傅磊
- 通讯作者:傅磊
数据更新时间:{{ journalArticles.updateTime }}
{{
item.title }}
{{ item.translation_title }}
- DOI:{{ item.doi || "--"}}
- 发表时间:{{ item.publish_year || "--" }}
- 期刊:{{ item.journal_name }}
- 影响因子:{{ item.factor || "--"}}
- 作者:{{ item.authors }}
- 通讯作者:{{ item.author }}
数据更新时间:{{ journalArticles.updateTime }}
{{ item.title }}
- 作者:{{ item.authors }}
数据更新时间:{{ monograph.updateTime }}
{{ item.title }}
- 作者:{{ item.authors }}
数据更新时间:{{ sciAawards.updateTime }}
{{ item.title }}
- 作者:{{ item.authors }}
数据更新时间:{{ conferencePapers.updateTime }}
{{ item.title }}
- 作者:{{ item.authors }}
数据更新时间:{{ patent.updateTime }}
其他文献
黏弹性人工边界在ABAQUS中的实现及地震动输入方法的比较研究
- DOI:10.13722/j.cnki.jrme.2019.1068
- 发表时间:2020
- 期刊:岩石力学与工程学报
- 影响因子:--
- 作者:马笙杰;迟明杰;陈红娟;陈苏
- 通讯作者:陈苏
自噬与癌症
- DOI:--
- 发表时间:2015
- 期刊:中国生物工程杂志
- 影响因子:--
- 作者:景园雅;陈平;常建锋;陈苏
- 通讯作者:陈苏
基于深度学习的地震震级分类
- DOI:10.11939/jass.20210046
- 发表时间:2022
- 期刊:地震学报
- 影响因子:--
- 作者:刘涛;戴志军;陈苏;傅磊
- 通讯作者:傅磊
多人工波速优化透射边界在谱元法地震波动模拟中的应用
- DOI:10.11939/jass.20210090
- 发表时间:2022
- 期刊:地震学报
- 影响因子:--
- 作者:邢浩洁;刘爱文;李小军;陈苏;傅磊
- 通讯作者:傅磊
弹性波模拟中局部透射边界的反射特征及参数优化
- DOI:--
- 发表时间:2022
- 期刊:振动与冲击
- 影响因子:--
- 作者:邢浩洁;李小军;刘爱文;李鸿晶;周正华;陈苏
- 通讯作者:陈苏
其他文献
{{
item.title }}
{{ item.translation_title }}
- DOI:{{ item.doi || "--" }}
- 发表时间:{{ item.publish_year || "--"}}
- 期刊:{{ item.journal_name }}
- 影响因子:{{ item.factor || "--" }}
- 作者:{{ item.authors }}
- 通讯作者:{{ item.author }}
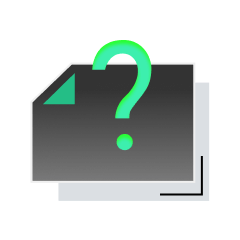
内容获取失败,请点击重试
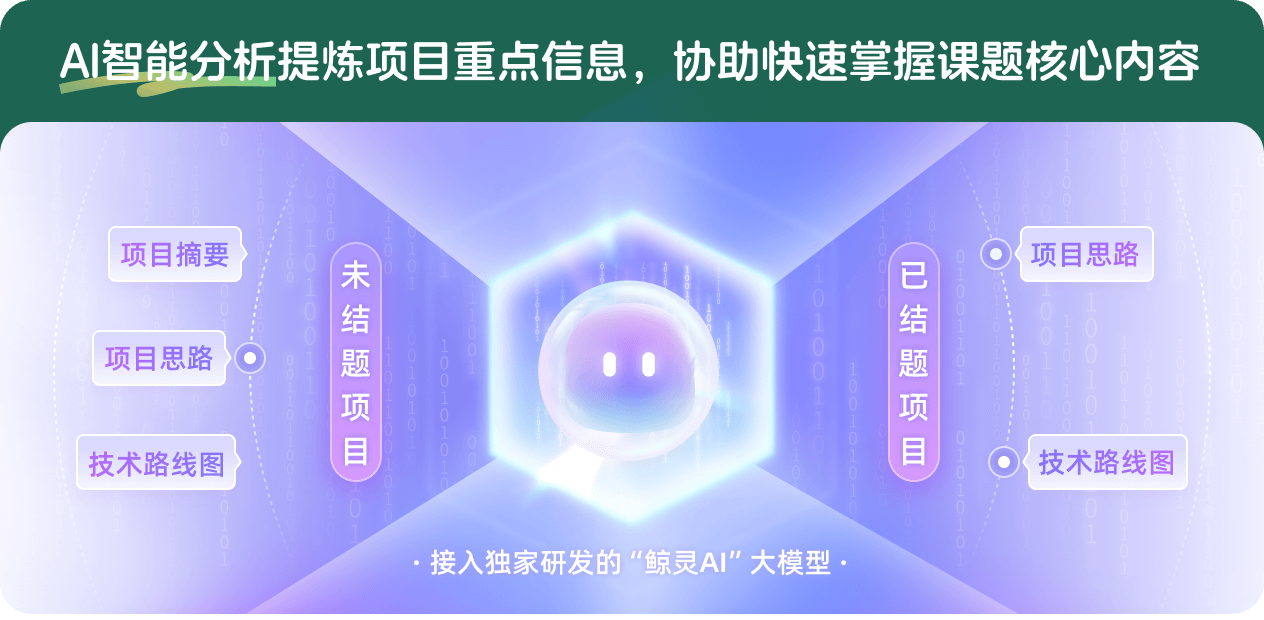
查看分析示例
此项目为已结题,我已根据课题信息分析并撰写以下内容,帮您拓宽课题思路:
AI项目摘要
AI项目思路
AI技术路线图
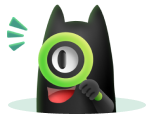
请为本次AI项目解读的内容对您的实用性打分
非常不实用
非常实用
1
2
3
4
5
6
7
8
9
10
您认为此功能如何分析更能满足您的需求,请填写您的反馈:
相似国自然基金
{{ item.name }}
- 批准号:{{ item.ratify_no }}
- 批准年份:{{ item.approval_year }}
- 资助金额:{{ item.support_num }}
- 项目类别:{{ item.project_type }}
相似海外基金
{{
item.name }}
{{ item.translate_name }}
- 批准号:{{ item.ratify_no }}
- 财政年份:{{ item.approval_year }}
- 资助金额:{{ item.support_num }}
- 项目类别:{{ item.project_type }}