基于结构深度学习群的空间目标识别技术研究
项目介绍
AI项目解读
基本信息
- 批准号:61471297
- 项目类别:面上项目
- 资助金额:82.0万
- 负责人:
- 依托单位:
- 学科分类:F0116.图像信息处理
- 结题年份:2018
- 批准年份:2014
- 项目状态:已结题
- 起止时间:2015-01-01 至2018-12-31
- 项目参与者:张秀伟; 魏巍; 朱宇; 张瑧; 陈雪凌; 郭婧; 丁晨; 严杭琦; 韩超;
- 关键词:
项目摘要
Space surveillance has long been recognized as playing a significant and prominent role in space exploration and national defense. Traditional space surveillance systems, however, are founded on ground-based radar and telescope networks, and hence have their intrinsic limitations. With the advances of space technologies, an increasing amount of resources have been devoted to developing space-based space surveillance (SBSS) systems, in which automated space target recognition using space imaging is an essential step. The challenges of space imaging-based space target recognition mainly arise from two clauses. First, due to the limited imaging hardware and orbit properties of both the imaging platform and target, space images are usually suffered from limited resolution, noise, inhomogeneous illumination, complex background and relatively heavy blur. Second, space targets are exceptionally diverse in shape, structure and functions. Therefore, conventional recognition techniques, which are largely based on "shallow" models such as perceptrons, support vector machines, decision trees and boosted classifiers, can hardly address these challenges successfully. This project aims to provide a holistic solution to space imaging-based automated space target recognition using the deep learning theory, for which novel models and algorithms will be developed. There will be three research components. First, we will develop a deep network-based unified image restoration and segmentation (DN-UIRS) model, which enables low-quality image restoration via constrained sparse reconstruction and critical target parts delineation via the joint use of the Markov random field model and deep belief network. Second, since very limited image samples can be captured for each target, we will incorporate network structure optimization and transfer learning methods into deep convolutional neural networks to create a novel deep learning (DL-SSL) model for small sample learning problems, and will apply this model to classifying the detected critical target parts. Third, as a single classifier may not be capable of identifying an extremely large number of space targets, we will design the structured deep learning group (SDLG) model for space target recognition, and will develop a novel population-based optimization technique called the social network algorithm (SNA), which mimics the information distribution and learning mechanism of social networks on the internet, for the joint and harmonious optimization of the SDLP model. The success of this project will advance the deep learning theory and techniques to improve the automated space image understanding and space target surveillance, which will in turn result in immediate impact to SBSS research and applications, as well as bring in significant benefits to space exploration and our national defense.
构建天基航天器和空间碎片监测系统,对宇航探索和空间安全都有重要意义。其中,利用空间平台获取的光学图像,进行空间目标识别,是天基空间监测的核心任务和关键技术之一。受载荷、功耗、轨道和速度等限制,空间平台对目标成像的距离远,时间短,视角变化大;因此空间图像模糊性高,分辨率低,光照不均,背景复杂,且目标在序列图像中存在明显的平移、旋转和尺度变化。本项目将针对这些挑战,提出一套基于空间图像的空间目标深度学习识别技术:针对图像低质量,提出基于自编码网络和深度信念网络的图像分割模型,提取目标局部构件;针对目标图像样本少,提出基于结构优化和迁移学习的小样本深度卷积网络模型,识别目标局部构件;针对目标形态复杂种类繁多,提出结构深度学习群模型,并设计社交网络算法对其进行联合协同优化,实现深度学习模型的并行训练和增量式学习,提高空间目标识别精度。研究成果将拓展深度学习的理论和应用领域,提高天基空间监测的水平。
结项摘要
构建天基航天器和空间碎片监测系统,对宇航探索和空间安全都有重要意义。其中,利用空间平台获取的光学图像,进行空间目标识别,是天基空间监测的核心任务和关键技术之一。受载荷、功耗、轨道和速度等限制,空间平台对目标成像的距离远,时间短,视角变化大;因此空间图像模糊性高,分辨率低,光照不均,背景复杂,且目标在序列图像中存在明显的平移、旋转和尺度变化。本项目针对这些挑战,重点探索了如何将目标区域的光滑性和连续性约束与深度学习模型相结合更好的解决目标分割问题,如何使用预训练和先验信息构造小样本深度卷积网络模型,以及设计网络群体优化算法对深度学习模型的结构进行联合优化,从并联或串联的深度集成学习、与传统方法相结合的深度学习、与领域先验知识相结合的深度学习、深度对抗学习、协同深度学习和深度注意力学习这六个方面着手,提出了一套用于空间目标检测、分割和分类识别的深度学习新模型和新方法,其中包括用于空间目标局部构件提取的图像增强及分割联合深度网络模型、用于空间目标局部构件分类的小样本深度卷积网络模型、用于空间目标分割、特征提取和识别的深度学习模型和基于仿生群体优化算法的深度学习模型结构优化算法。项目期间,构造了STK和ISS这两个来源不同的仿真数据库;累计申请发明专利13项,已获授权1项;在本领域一流国际学术期刊和会议发表(含录用)论文56篇,包括30篇期刊论文和26篇会议论文;其中,以第一作者或通讯作者身份发表论文40篇,包括16篇期刊论文和24篇会议论文。以第一作者或通讯作者身份在中科院认定的顶级和一区期刊IEEE-TMI和Information Fusion上各发表论文1篇,在IEEE-JBHI和Applied Soft Computing等二区期刊发表论文7篇,在其它SCI期刊6篇。本项目的成果提高了空间目标的识别精度,拓展了深度学习的理论和应用,为空间监视和空间碎片监测提供了潜在的技术支持。
项目成果
期刊论文数量(30)
专著数量(0)
科研奖励数量(0)
会议论文数量(26)
专利数量(13)
Normalized Non-Negative Sparse Encoder for Fast Image Representation
用于快速图像表示的归一化非负稀疏编码器
- DOI:10.1109/tcsvt.2018.2852731
- 发表时间:2019-07
- 期刊:IEEE Transactions on Circuits and Systems for Video Technology
- 影响因子:8.4
- 作者:Shizhou Zhang;Jinjun Wang;Weiwei Shi;Yihong Gong;Yong Xia;Yanning Zhang
- 通讯作者:Yanning Zhang
GA-SVM based feature selection and parameter optimization in hospitalization expense modeling
基于GA-SVM的住院费用建模特征选择与参数优化
- DOI:10.1016/j.asoc.2018.11.001
- 发表时间:2019-02-01
- 期刊:APPLIED SOFT COMPUTING
- 影响因子:8.7
- 作者:Zhou Tao;Lu Huiling;Yong Xia
- 通讯作者:Yong Xia
Cell image segmentation using bacterial foraging optimization
使用细菌觅食优化进行细胞图像分割
- DOI:10.1016/j.asoc.2017.05.019
- 发表时间:2017-09-01
- 期刊:APPLIED SOFT COMPUTING
- 影响因子:8.7
- 作者:Pan, Yongsheng;Xia, Yong;Fulham, Michael
- 通讯作者:Fulham, Michael
Pedestrian search in surveillance videos by learning discriminative deep features
通过学习判别性深度特征在监控视频中进行行人搜索
- DOI:10.1016/j.neucom.2017.12.042
- 发表时间:2017-12
- 期刊:Neurocomputing
- 影响因子:6
- 作者:Zhang Shizhou;Cheng De;Gong Yihong;Shi Dahu;Qiu Xi;Xia Yong;Zhang Yanning
- 通讯作者:Zhang Yanning
Affective Image Classification by Jointly Using Interpretable Art Features and Learned Semantic Annotations
联合使用可解释的艺术特征和学习的语义注释进行情感图像分类
- DOI:--
- 发表时间:2018
- 期刊:Journal of Visual Communication and Image Representation
- 影响因子:2.6
- 作者:Xuan Liu;Na Li;Yong Xia
- 通讯作者:Yong Xia
数据更新时间:{{ journalArticles.updateTime }}
{{
item.title }}
{{ item.translation_title }}
- DOI:{{ item.doi || "--"}}
- 发表时间:{{ item.publish_year || "--" }}
- 期刊:{{ item.journal_name }}
- 影响因子:{{ item.factor || "--"}}
- 作者:{{ item.authors }}
- 通讯作者:{{ item.author }}
数据更新时间:{{ journalArticles.updateTime }}
{{ item.title }}
- 作者:{{ item.authors }}
数据更新时间:{{ monograph.updateTime }}
{{ item.title }}
- 作者:{{ item.authors }}
数据更新时间:{{ sciAawards.updateTime }}
{{ item.title }}
- 作者:{{ item.authors }}
数据更新时间:{{ conferencePapers.updateTime }}
{{ item.title }}
- 作者:{{ item.authors }}
数据更新时间:{{ patent.updateTime }}
其他文献
羧甲基壳聚糖对Nd~(3+)的吸附性能
- DOI:10.16577/j.cnki.42-1215/tb.2016.07.022
- 发表时间:2016
- 期刊:材料保护
- 影响因子:--
- 作者:罗序燕;祝婷;夏勇;邓金梅;刘峰生
- 通讯作者:刘峰生
双模式涡旋光束的轨道角动量的精确识别
- DOI:--
- 发表时间:2021
- 期刊:激光与光电子学进展
- 影响因子:--
- 作者:叶玉儿;李军依;曹萌;夏勇
- 通讯作者:夏勇
第三讲 化学稳定分子的激光减速、冷却及其MOT技术
- DOI:--
- 发表时间:2018
- 期刊:物理
- 影响因子:--
- 作者:夏勇;汪海玲;许亮;印建平
- 通讯作者:印建平
基于粗糙集特征级的融合肺结节检测算法
- DOI:--
- 发表时间:2016
- 期刊:电视技术
- 影响因子:--
- 作者:张俊杰;周涛;夏勇;王文文
- 通讯作者:王文文
贵州水银洞卡林型金矿床方解石稀土元素地球化学特征与找矿意义
- DOI:--
- 发表时间:2020
- 期刊:矿物学报
- 影响因子:--
- 作者:徐碧良;谭亲平;夏勇;赵亦猛;陈明;王泽鹏
- 通讯作者:王泽鹏
其他文献
{{
item.title }}
{{ item.translation_title }}
- DOI:{{ item.doi || "--" }}
- 发表时间:{{ item.publish_year || "--"}}
- 期刊:{{ item.journal_name }}
- 影响因子:{{ item.factor || "--" }}
- 作者:{{ item.authors }}
- 通讯作者:{{ item.author }}
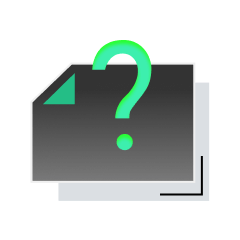
内容获取失败,请点击重试
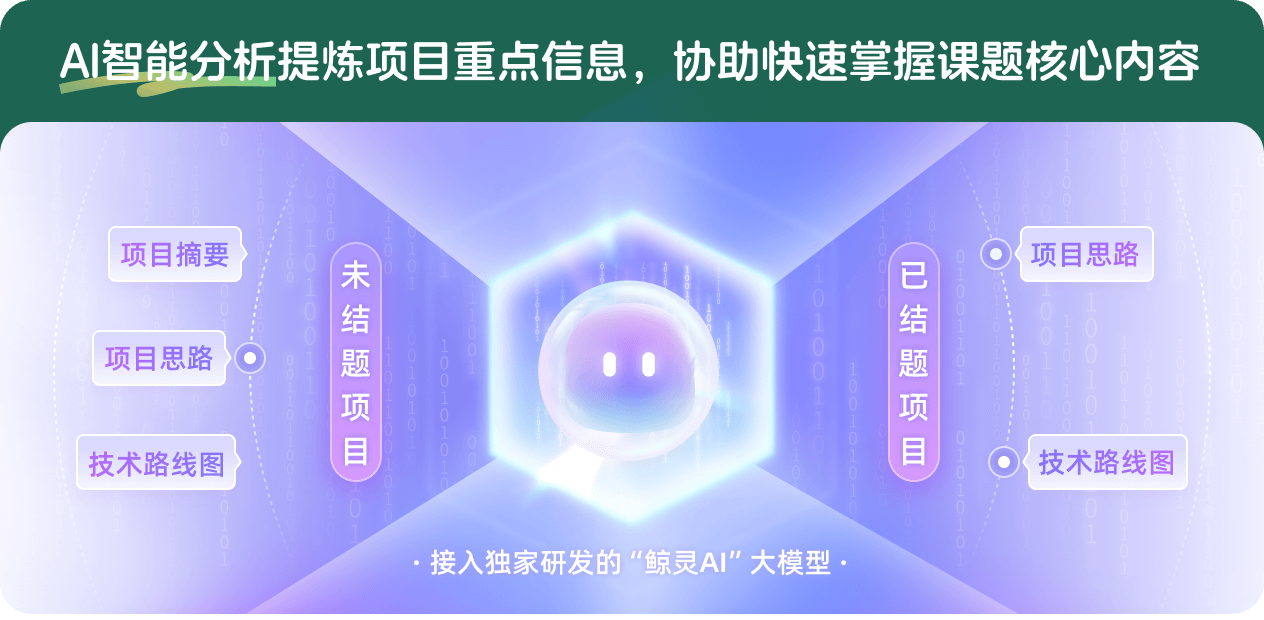
查看分析示例
此项目为已结题,我已根据课题信息分析并撰写以下内容,帮您拓宽课题思路:
AI项目摘要
AI项目思路
AI技术路线图
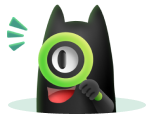
请为本次AI项目解读的内容对您的实用性打分
非常不实用
非常实用
1
2
3
4
5
6
7
8
9
10
您认为此功能如何分析更能满足您的需求,请填写您的反馈:
夏勇的其他基金
基于自集成、域关联和人机协同的胸片智能诊断技术
- 批准号:62171377
- 批准年份:2021
- 资助金额:64 万元
- 项目类别:面上项目
智能化肺结节辅助诊断中的人工智能技术研究
- 批准号:61771397
- 批准年份:2017
- 资助金额:64.0 万元
- 项目类别:面上项目
相似国自然基金
{{ item.name }}
- 批准号:{{ item.ratify_no }}
- 批准年份:{{ item.approval_year }}
- 资助金额:{{ item.support_num }}
- 项目类别:{{ item.project_type }}
相似海外基金
{{
item.name }}
{{ item.translate_name }}
- 批准号:{{ item.ratify_no }}
- 财政年份:{{ item.approval_year }}
- 资助金额:{{ item.support_num }}
- 项目类别:{{ item.project_type }}