基于压缩学习的深度神经网络专用处理芯片研究
项目介绍
AI项目解读
基本信息
- 批准号:61876039
- 项目类别:面上项目
- 资助金额:62.0万
- 负责人:
- 依托单位:
- 学科分类:F0608.智能系统与人工智能安全
- 结题年份:2022
- 批准年份:2018
- 项目状态:已结题
- 起止时间:2019-01-01 至2022-12-31
- 项目参与者:高凤楠; 陈更生; 环宇翔; 徐佳唯; 褚皓明; 金怡; 赵宇晨;
- 关键词:
项目摘要
Smart devices with learning capabilities are the key enabler for ubiquitous intelligence in the context of the Internet of Things (IoT). The project aims at sub-10 mW deep learning processor for embedded IoT devices. Compressed learning that incorporates compressive sampling and deep neural network (DNN) is proposed. Learning (training and inference) is directly performed in the measurement domain, without the need of a signal reconstruction process. Power reduction is achieved by reduced data samples through a compressive sampling frontend. Trade-offs will be intensively investigated with respects to the sparsity of input data, compression ratio, and the architecture of the DNN. A reconfigurable application-specific processor will be designed by exploiting techniques of network compression, near-zero approximation and dynamic precision, resulting in an algorithm-architecture-circuits co-design for power-aware hardware optimization. The design will be evaluated in FPGA and implemented in a 55 nm or below CMOS process, targeting >15X energy efficiency improvements over conventional approaches.
深度神经网络处理器如何满足物联网终端本地智能计算和能耗要求,已经成为一个亟待解决的关键科学问题。本项目面向物联网终端,研究<10mW深度神经网络专用处理芯片。项目拟通过压缩采样与深度学习的结合,即压缩学习,直接在观测域对压缩的数据进行处理,降低数据输入量并避免复杂的信号重建,以提高处理的能效。本项目将:1)建立压缩学习的仿真模型与设计框架,联合优化压缩采样参数和深度神经网络结构;2)利用近似计算扩展模型稀疏度,并进行模型压缩;3)针对压缩学习模型优化处理架构和计算单元,实现算法模型在专用硬件上的高效映射。拟研专用处理芯片将在55nm或更先进CMOS工艺下进行流片验证。相比传统的直接采用原始数据的深度学习方法,目标达到>15X的能效提升。项目对减少“云-端”通信负担与延时,实现物联网终端本地化智能,构建分布式智能网络有重要意义。
结项摘要
本项目面向智能物联网终端与边缘节点智能化与高能效的需求,研究了基于压缩采样与神经网络结合的压缩学习方法,利用物联网信号稀疏与神经网络模型稀疏的特性,从降低输入信号维度和模型复杂度等两个方面,实现了物联网智能信息处理的低功耗化。项目建立了端到端的压缩学习框架与网络搜索方法;发展了近零稀疏和非线性量化的稀疏扩展方法;提出了基于脉冲神经网络的物联网稀疏信号训练方法;完成了支持压缩学习的可重构加速器、对数量化加速器关键模块、脉冲神经网络处理器单元等芯片的设计仿真、FPGA原型验证、MPW流片测试等工作,在模型压缩率、网络准确度、芯片功率与能效等关键指标方面均达到预期目标。本项目执行了预期的各项研究计划,发表了包括IEEE TCAS-I、IEEE TBCAS、IEEE TCAS-II、IEEE TII等数篇高水平论文,在健康物联网应用中进行了应用验证,培养毕业了8位研究生。本项目形成的技术方案与关键电路对构建高能效智能物联网芯片与系统的有重要参考价值。
项目成果
期刊论文数量(13)
专著数量(0)
科研奖励数量(0)
会议论文数量(13)
专利数量(3)
Multi-Sensor Fusion for Navigation and Mapping in Autonomous Vehicles: Accurate Localization in Urban Environments
用于自动驾驶车辆导航和地图绘制的多传感器融合:城市环境中的精确定位
- DOI:10.1142/s2301385020500168
- 发表时间:2020-07-01
- 期刊:UNMANNED SYSTEMS
- 影响因子:5.3
- 作者:Li Qingqing;Queralta, Jorge Pena;Westerlund, Tomi
- 通讯作者:Westerlund, Tomi
A Memory-Efficient CNN Accelerator Using Segmented Logarithmic Quantization and Multi-Cluster Architecture
使用分段对数量化和多集群架构的内存高效 CNN 加速器
- DOI:10.1109/tcsii.2020.3038897
- 发表时间:2021-06
- 期刊:IEEE Transactions on Circuits and Systems II: Express Briefs
- 影响因子:--
- 作者:Jiawei Xu;Yuxiang Huan;Boming Huang;Haoming Chu;Yi Jin;Lirong Zheng;Zhuo Zou
- 通讯作者:Zhuo Zou
Base-Reconfigurable Segmented Logarithmic Quantization and Hardware Design for Deep Neural Networks
深度神经网络的基本可重构分段对数量化和硬件设计
- DOI:10.1007/s11265-020-01557-8
- 发表时间:2020-07
- 期刊:Journal of Signal Processing Systems
- 影响因子:--
- 作者:Jiawei Xu;Yuxiang Huan;Yi Jin;Haoming Chu;Lirong Zheng;Zhuo Zou
- 通讯作者:Zhuo Zou
Self-Aware Distributed Deep Learning Framework for Heterogeneous IoT Edge Devices
适用于异构物联网边缘设备的自我感知分布式深度学习框架
- DOI:10.1016/j.future.2021.07.010
- 发表时间:2021
- 期刊:Future Generation Computer Systems
- 影响因子:--
- 作者:Yi Jin;Jiawei Cai;Jiawei Xu;Yuxiang Huan;Yan Yulong;Bin Huang;Yongliang Guo;Lirong Zheng;Zhuo Zou
- 通讯作者:Zhuo Zou
AIOC: An All-In-One-Card Hardware Design for Financial Market Trading System
AIOC:金融市场交易系统一体化卡硬件设计
- DOI:10.1109/tcsii.2022.3167312
- 发表时间:2022
- 期刊:IEEE Transactions on Circuits and Systems II: Express Briefs
- 影响因子:--
- 作者:Boming Huang;Yuxiang Huan;Hao Jia;Chen Ding;Yulong Yan;Bin Huang;Lirong Zheng;Zhuo Zou
- 通讯作者:Zhuo Zou
数据更新时间:{{ journalArticles.updateTime }}
{{
item.title }}
{{ item.translation_title }}
- DOI:{{ item.doi || "--"}}
- 发表时间:{{ item.publish_year || "--" }}
- 期刊:{{ item.journal_name }}
- 影响因子:{{ item.factor || "--"}}
- 作者:{{ item.authors }}
- 通讯作者:{{ item.author }}
数据更新时间:{{ journalArticles.updateTime }}
{{ item.title }}
- 作者:{{ item.authors }}
数据更新时间:{{ monograph.updateTime }}
{{ item.title }}
- 作者:{{ item.authors }}
数据更新时间:{{ sciAawards.updateTime }}
{{ item.title }}
- 作者:{{ item.authors }}
数据更新时间:{{ conferencePapers.updateTime }}
{{ item.title }}
- 作者:{{ item.authors }}
数据更新时间:{{ patent.updateTime }}
其他文献
其他文献
{{
item.title }}
{{ item.translation_title }}
- DOI:{{ item.doi || "--" }}
- 发表时间:{{ item.publish_year || "--"}}
- 期刊:{{ item.journal_name }}
- 影响因子:{{ item.factor || "--" }}
- 作者:{{ item.authors }}
- 通讯作者:{{ item.author }}
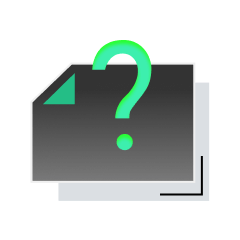
内容获取失败,请点击重试
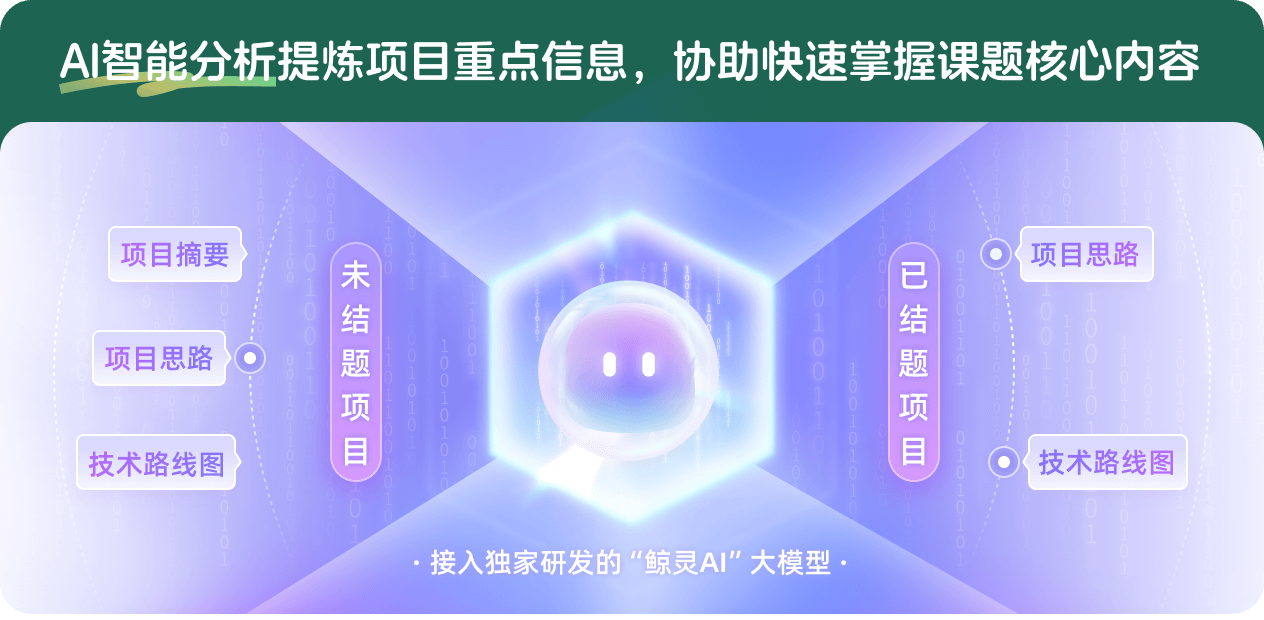
查看分析示例
此项目为已结题,我已根据课题信息分析并撰写以下内容,帮您拓宽课题思路:
AI项目摘要
AI项目思路
AI技术路线图
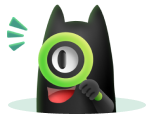
请为本次AI项目解读的内容对您的实用性打分
非常不实用
非常实用
1
2
3
4
5
6
7
8
9
10
您认为此功能如何分析更能满足您的需求,请填写您的反馈:
邹卓的其他基金
面向类脑计算与人工智能芯片的集成电路高效设计方法
- 批准号:62011530132
- 批准年份:2020
- 资助金额:39.3 万元
- 项目类别:国际(地区)合作与交流项目
相似国自然基金
{{ item.name }}
- 批准号:{{ item.ratify_no }}
- 批准年份:{{ item.approval_year }}
- 资助金额:{{ item.support_num }}
- 项目类别:{{ item.project_type }}
相似海外基金
{{
item.name }}
{{ item.translate_name }}
- 批准号:{{ item.ratify_no }}
- 财政年份:{{ item.approval_year }}
- 资助金额:{{ item.support_num }}
- 项目类别:{{ item.project_type }}