Bach2调控CD45RA-Foxp3low T细胞影响B细胞功能及其在系统性红斑狼疮中作用的机制研究
项目介绍
AI项目解读
基本信息
- 批准号:81873863
- 项目类别:面上项目
- 资助金额:57.0万
- 负责人:
- 依托单位:
- 学科分类:H1107.自身免疫性疾病
- 结题年份:2022
- 批准年份:2018
- 项目状态:已结题
- 起止时间:2019-01-01 至2022-12-31
- 项目参与者:潘秀军; 马妍慧; 姚瑶; 刘毅; 韩丽; 陆一闻; 任懿倩;
- 关键词:
项目摘要
Systemic lupus erythematosus (SLE) is an autoimmune disease characterizes the disorder of the immune system, though the detail mechanisms are not fully clear.In our previous study, we found that the CD4+CD45RA-Foxp3low T (Fr.III) cells express high levels of costimulatory molecules and IL-17 inflammatory cytokines, promote B cells proliferation and differentiation into plasmablast cells. But the transcriptional factor Bach2 expression was much lower, and was negatively correlated with the SLE disease activity index. Overexpression of Bach2 into the Fr.III cells reduced IL-17 production and B cells proliferation. So we suggest a hypothesis that Bach2 could regulate Fr.III cytokines-secreting T cells function and promote B cells response in the SLE patients. First, we will study the role of Fr.III cells in the regulation of B cells response in the healthy controls and SLE patients. Second, we’d like to use the ChIP-Seq to explore the molecular mechanisms of how the Bach2 regulates Fr.III cells function and why the lower expression of Bach2 in SLE patients. Third, we will use Bach2 conditional knockout mouse to study the role of Fr.III cells in regulation of B cells response in the SLE mouse model. Our study will demonstrate a new insight of immunological mechanism into the pathogenesis of SLE, and may provide a new target for immune intervention therapy of SLE.
系统性红斑狼疮(SLE)是一种自身免疫性疾病,发病机制不明。我们前期研究发现SLE患者CD4+CD45RA-Foxp3lowT(Fr.III)细胞高表达共刺激分子和IL-17促进B细胞增值分化,但核转录因子Bach2表达明显降低,与SLE疾病活动指数呈负相关;Fr.III细胞过表达Bach2减少IL-17分泌和B细胞增殖分化。因此提出假设Bach2可通过调控Fr.III细胞影响B细胞功能进而参与SLE进程。本课题拟先探讨Fr.III细胞在正常人和SLE病人中对B细胞功能作用;再通过ChIP-Seq等研究Bach2对Fr.III细胞功能调节及SLE中调控Bach2表达分子通路;最后利用Bach2条件敲除鼠探索Bach2调控Fr.III细胞影响B细胞功能及其在SLE中作用机制。研究结果从全新视角阐明SLE发病免疫学新机制,为寻找免疫干预治疗提供新靶点。
结项摘要
系统性红斑狼疮是一种异质性自身免疫性疾病,其发病机制尚未明确,涉及免疫系统的紊乱。近年来,关于SLE治疗并没有突破性进展,因此深入探讨SLE发病机制,寻找新的治疗靶点,成为目前研究热点。本项目究发现SLE患者CD4+CD45RA-Foxp3low T(Fr.III)细胞增多,产生大量炎症因子IL-17,TNF,与B细胞共培养时发现SLE病人的Fr.III细胞可以减少B细胞凋亡,促进其增值并分化为浆母细胞,促进抗体IgG,IgA分泌。进一步研究发现SLE病人Fr.III细胞表达高水平共刺激分子ICOS,CD154,但核转录因子Bach2表达明显低于正常对照组,Fr.III细胞中Bach2的表达量与SLE疾病活动指数(SLEDAI)呈显著负相关; SLE病人 Fr.III细胞过表达Bach2后,和对照组相比,分泌IL-17能力显著下降,辅助B细胞增殖及分化成为浆母细胞能力下降;SLE病人血清刺激Fr.III细胞,Bach2表达降低,能够通过调控H3K27ac水平调控Bach2表达;进一步构建CD4+T细胞Bach2条件敲除鼠中,发现条件敲除鼠能够自发狼疮性肾炎,肺部和肾脏组织炎症性细胞浸润增加,小鼠体重下降,自身免疫性抗体anti-dsDNA IgG, anti-AND IgG抗体浓度增加;RNA测序发现Fr.III细胞富集了调控B细胞反应的相关通路,mir-148a在Fr.III细胞中表达增高,能够下调Bach2的表达。课题阐明Fr.III细胞对B细胞的促进作用并探讨相关的因子和通路;探索SLE病人Fr.III细胞Bach2降低的分子机制,探讨Bach2对Fr.III细胞数量功能调控的信号通路;进一步通过转基因鼠从体内研究Bach2在SLE中的作用,阐明Bach2通过调控Fr.III细胞影响B细胞功能的分子新机制,以及mir-148a调控Bach2的表达。研究结果从全新视角阐明SLE发病的免疫学新机制,为寻找免疫干预治疗SLE提供新思路及靶点,具有重要的科学意义和临床研究价值。
项目成果
期刊论文数量(5)
专著数量(0)
科研奖励数量(0)
会议论文数量(0)
专利数量(0)
PRMT5 Inhibition Promotes PD-L1 Expression and Immuno-Resistance in Lung Cancer.
PRMT5 抑制促进肺癌中 PD-L1 的表达和免疫抵抗
- DOI:10.3389/fimmu.2021.722188
- 发表时间:2021
- 期刊:Frontiers in immunology
- 影响因子:7.3
- 作者:Hu R;Zhou B;Chen Z;Chen S;Chen N;Shen L;Xiao H;Zheng Y
- 通讯作者:Zheng Y
Immune suppressive landscape in the human esophageal squamous cell carcinoma microenvironment.
人食管鳞状细胞癌微环境中的免疫抑制景观
- DOI:10.1038/s41467-020-20019-0
- 发表时间:2020-12-08
- 期刊:Nature communications
- 影响因子:16.6
- 作者:Zheng Y;Chen Z;Han Y;Han L;Zou X;Zhou B;Hu R;Hao J;Bai S;Xiao H;Li WV;Bueker A;Ma Y;Xie G;Yang J;Chen S;Li H;Cao J;Shen L
- 通讯作者:Shen L
数据更新时间:{{ journalArticles.updateTime }}
{{
item.title }}
{{ item.translation_title }}
- DOI:{{ item.doi || "--"}}
- 发表时间:{{ item.publish_year || "--" }}
- 期刊:{{ item.journal_name }}
- 影响因子:{{ item.factor || "--"}}
- 作者:{{ item.authors }}
- 通讯作者:{{ item.author }}
数据更新时间:{{ journalArticles.updateTime }}
{{ item.title }}
- 作者:{{ item.authors }}
数据更新时间:{{ monograph.updateTime }}
{{ item.title }}
- 作者:{{ item.authors }}
数据更新时间:{{ sciAawards.updateTime }}
{{ item.title }}
- 作者:{{ item.authors }}
数据更新时间:{{ conferencePapers.updateTime }}
{{ item.title }}
- 作者:{{ item.authors }}
数据更新时间:{{ patent.updateTime }}
其他文献
系统性红斑狼疮患者CD4+CD25+CD45RA-T 细胞中转录因子Bach2的表达及其影响
- DOI:--
- 发表时间:2018
- 期刊:上海交通大学学报(医学版)
- 影响因子:--
- 作者:陆一闻;郑英霞;潘秀军;聂红
- 通讯作者:聂红
其他文献
{{
item.title }}
{{ item.translation_title }}
- DOI:{{ item.doi || "--" }}
- 发表时间:{{ item.publish_year || "--"}}
- 期刊:{{ item.journal_name }}
- 影响因子:{{ item.factor || "--" }}
- 作者:{{ item.authors }}
- 通讯作者:{{ item.author }}
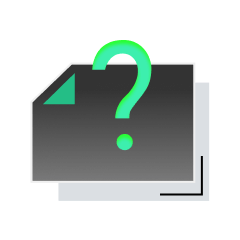
内容获取失败,请点击重试
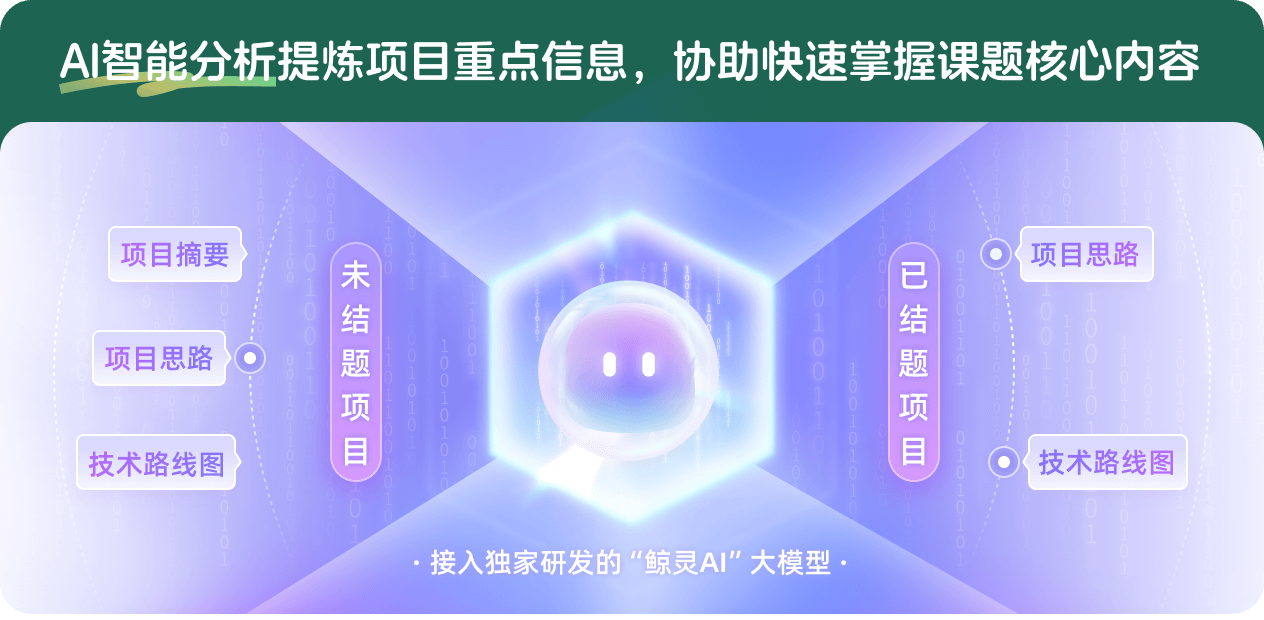
查看分析示例
此项目为已结题,我已根据课题信息分析并撰写以下内容,帮您拓宽课题思路:
AI项目摘要
AI项目思路
AI技术路线图
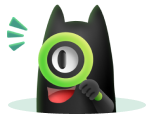
请为本次AI项目解读的内容对您的实用性打分
非常不实用
非常实用
1
2
3
4
5
6
7
8
9
10
您认为此功能如何分析更能满足您的需求,请填写您的反馈:
郑英霞的其他基金
蛋白精氨酸甲基化转移酶PRMT5调控PPARG促进巨噬细胞M2极化及其在肿瘤中作用的机制研究
- 批准号:82371738
- 批准年份:2023
- 资助金额:49.00 万元
- 项目类别:面上项目
利用单细胞测序技术研究PRMT5在多发性硬化和克罗恩病中的不同作用及其机制研究
- 批准号:
- 批准年份:2020
- 资助金额:55 万元
- 项目类别:面上项目
蛋白精氨酸甲基化转移酶PRMT5调节T细胞亚群分化及其在炎症性肠病中作用的机制研究
- 批准号:81571525
- 批准年份:2015
- 资助金额:58.0 万元
- 项目类别:面上项目
类风湿性关节炎关中PKCa下调与FOXP3磷酸化和Treg细胞功能降低机制研究
- 批准号:81202351
- 批准年份:2012
- 资助金额:23.0 万元
- 项目类别:青年科学基金项目
相似国自然基金
{{ item.name }}
- 批准号:{{ item.ratify_no }}
- 批准年份:{{ item.approval_year }}
- 资助金额:{{ item.support_num }}
- 项目类别:{{ item.project_type }}
相似海外基金
{{
item.name }}
{{ item.translate_name }}
- 批准号:{{ item.ratify_no }}
- 财政年份:{{ item.approval_year }}
- 资助金额:{{ item.support_num }}
- 项目类别:{{ item.project_type }}