大规模张量特征值问题的优化算法研究
项目介绍
AI项目解读
基本信息
- 批准号:11771210
- 项目类别:面上项目
- 资助金额:48.0万
- 负责人:
- 依托单位:
- 学科分类:A0405.连续优化
- 结题年份:2021
- 批准年份:2017
- 项目状态:已结题
- 起止时间:2018-01-01 至2021-12-31
- 项目参与者:周光辉; 王丽平; 刘浩; 杨维维; 马腾飞; 庞瑞; 朱文焕;
- 关键词:
项目摘要
In the last decade,the tensor eigenvalue problem becomes a hot research issue. This problem has numerous applications in high order data analysis, automate control, magnetic resonance imaging , quantum information, signal processing, higher order Markov chains, spectral graph theory, multi-label learning and so on. In general, it is NP-hard to compute eigenvalues of a tensor,and the current research results are suitable for small scaleproblems. It is meaningful to study the efficient methods for solving large scale tensor eigenvalue problems.. This program will study the optimization methods for solving large scale tensor eigenvalue problems. Four parts of researches are: (1) the low order convergent algorithms for solving real tensor eigenvalue problem;(2)the high order convergent algorithms for large scale tensor eigenvalue problems;(3)the research on the computing properties of tensor and vector;(4) the methods for large scale special tensor eigenvalue problem. The study will propose some basic research contribution and some efficient algorithms for solving tensor eigenvalue problems, and establish the their convengent theories.
近十多年来,张量特征值问题的研究成为一个热点研究问题. 这一问题在高维数据分析、自动控制、核磁共振成像、量子信息、信号处理、高阶马尔科夫链、超图理论、多标记学习等众多领域都有重要应用. 张量特征值问题是NP难的,目前的研究成果主要解小规模问题,因此研究大规模特征值问题的优化算法是非常有意义的. . 本课题将研究大规模张量特征值问题的各类优化算法,研究内容包括:(1)大规模张量特征值的低阶收敛优化方法研究;(2) 大规模实对称张量特征值的高阶收敛算法研究;(3)张量与多重向量计算性质的研究;(4) 大规模特殊张量特征值的算法研究. 本课题的研究为张量的特征值问题提供基础理论与更多有效的算法,并建立相关算法的收敛性理论.
结项摘要
近十多年来,张量特征值问题的研究成为一个热点研究问题. 这一问题在高维数据分析、 自动控制、核磁共振成像、量子信息、信号处理、高阶马尔科夫链、超图理论、多标记学习等众多领域都有重要应用。本课题研究了大规模张量特征值问题的各类优化算法,研究内容包括:(1)大规模张量特征值的收敛优化方法研究;(2) 大规模实对称张量特征值的高阶收敛算法研究;(3)大规模特殊张量特征值的算法研究;(4) 一般与大规模张量特征值的逆迭代算法的研究。在课题资助下,共发表高水平论文12篇,完成博士论文3篇,这些研究成果为张量的特征值问题提供基础理论与更多有效的算法,为人工智能等领域的应用提供了技术支撑。
项目成果
期刊论文数量(11)
专著数量(0)
科研奖励数量(0)
会议论文数量(0)
专利数量(0)
Computing the largest H-eigenvalue of large-scale tensors generated from directed hypergraphs
计算有向超图生成的大规模张量的最大 H 特征值
- DOI:10.1007/s40314-021-01554-y
- 发表时间:2021
- 期刊:COMPUTATIONAL & APPLIED MATHEMATICS
- 影响因子:2.6
- 作者:Zhang Xin;Ni Qin;Sheng Zhou;Ge Zhili
- 通讯作者:Ge Zhili
Improved convergence results of an efficient Levenberg–Marquardt method for nonlinear equations
改进非线性方程的高效 Levenberg–Marquardt 方法的收敛结果
- DOI:10.1007/s12190-021-01599-6
- 发表时间:--
- 期刊:Journal of Applied Mathematics and Computing
- 影响因子:2.2
- 作者:曾梅兰;周光辉
- 通讯作者:周光辉
A generalization of inverse power method for computing eigenpairs of symmetric tensors
计算对称张量特征对的逆幂法的推广
- DOI:10.1080/03081087.2021.1902461
- 发表时间:--
- 期刊:Linear and Multilinear Algebra
- 影响因子:1.1
- 作者:Zhou Sheng;Qin Ni
- 通讯作者:Qin Ni
Globally convergent inverse iteration algorithm for finding the largest eigenvalue of a nonnegative weakly irreducible tensors
用于查找非负弱不可约张量的最大特征值的全局收敛逆迭代算法
- DOI:--
- 发表时间:2021
- 期刊:Pacific Journal of Optimization
- 影响因子:0.2
- 作者:盛州;倪勤
- 通讯作者:倪勤
Local convergence analysis of inverse iteration algorithm for computing the H-spectral radius of a nonnegative weakly irreducible tensor
计算非负弱不可约张量H谱半径的逆迭代算法的局部收敛性分析
- DOI:10.1016/j.cam.2019.02.014
- 发表时间:2019
- 期刊:Journal of Computational and Applied Mathematics
- 影响因子:2.4
- 作者:Sheng Zhou;Ni Qin;Yuan Gonglin
- 通讯作者:Yuan Gonglin
数据更新时间:{{ journalArticles.updateTime }}
{{
item.title }}
{{ item.translation_title }}
- DOI:{{ item.doi || "--"}}
- 发表时间:{{ item.publish_year || "--" }}
- 期刊:{{ item.journal_name }}
- 影响因子:{{ item.factor || "--"}}
- 作者:{{ item.authors }}
- 通讯作者:{{ item.author }}
数据更新时间:{{ journalArticles.updateTime }}
{{ item.title }}
- 作者:{{ item.authors }}
数据更新时间:{{ monograph.updateTime }}
{{ item.title }}
- 作者:{{ item.authors }}
数据更新时间:{{ sciAawards.updateTime }}
{{ item.title }}
- 作者:{{ item.authors }}
数据更新时间:{{ conferencePapers.updateTime }}
{{ item.title }}
- 作者:{{ item.authors }}
数据更新时间:{{ patent.updateTime }}
其他文献
基于共轭梯度的锥模型信赖域算法
- DOI:--
- 发表时间:2011
- 期刊:高等学校计算数学学报
- 影响因子:--
- 作者:陆晓平;倪勤
- 通讯作者:倪勤
强迫下降的三项共轭梯度法
- DOI:--
- 发表时间:2012
- 期刊:数值计算与计算机应用
- 影响因子:--
- 作者:陈龙卫;倪勤;张欣
- 通讯作者:张欣
强迫下降的三项共轭梯度法
- DOI:--
- 发表时间:2012
- 期刊:数值计算与计算机应用
- 影响因子:--
- 作者:陈龙卫;倪勤;张欣
- 通讯作者:张欣
一个新锥模型信赖域算法
- DOI:--
- 发表时间:--
- 期刊:已投《高等学校计算数学学报》
- 影响因子:--
- 作者:吴海平;倪勤
- 通讯作者:倪勤
HBx-siRNA快速筛选体系的建立和
- DOI:--
- 发表时间:--
- 期刊:浙江大学学报(医学版) 2004,33(4):300-305,310.
- 影响因子:--
- 作者:倪勤;刘克洲*;羊正纲;姚航平
- 通讯作者:姚航平
其他文献
{{
item.title }}
{{ item.translation_title }}
- DOI:{{ item.doi || "--" }}
- 发表时间:{{ item.publish_year || "--"}}
- 期刊:{{ item.journal_name }}
- 影响因子:{{ item.factor || "--" }}
- 作者:{{ item.authors }}
- 通讯作者:{{ item.author }}
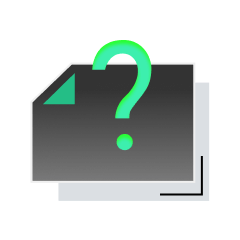
内容获取失败,请点击重试
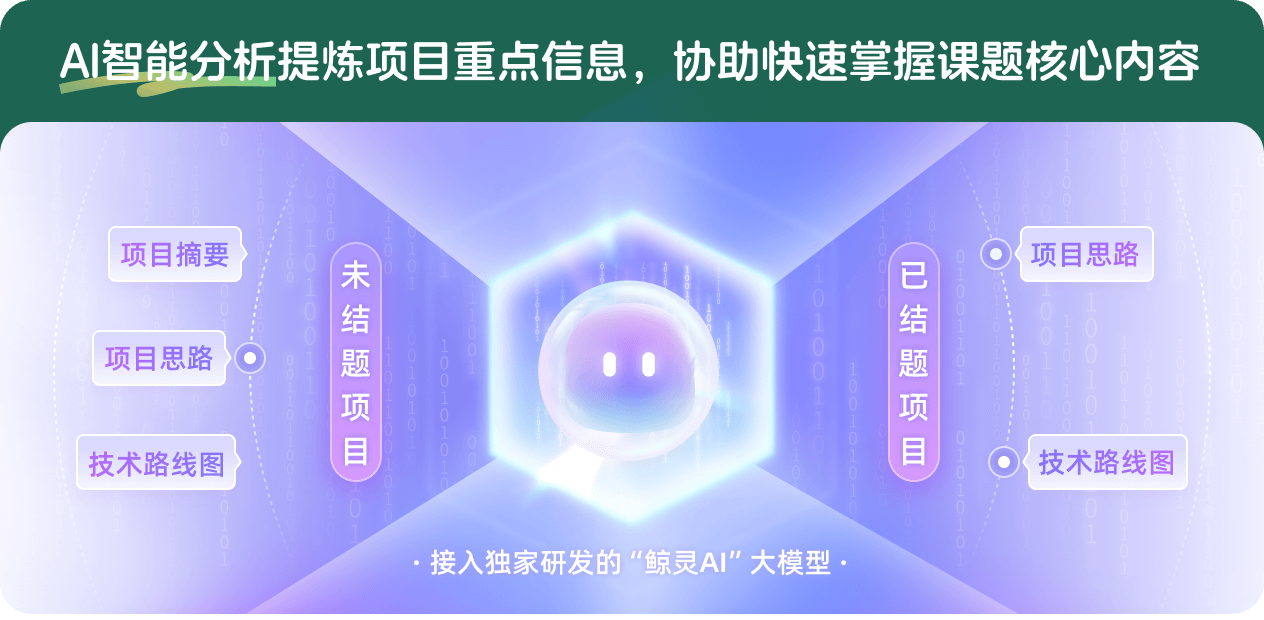
查看分析示例
此项目为已结题,我已根据课题信息分析并撰写以下内容,帮您拓宽课题思路:
AI项目摘要
AI项目思路
AI技术路线图
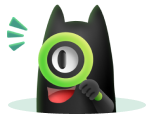
请为本次AI项目解读的内容对您的实用性打分
非常不实用
非常实用
1
2
3
4
5
6
7
8
9
10
您认为此功能如何分析更能满足您的需求,请填写您的反馈:
倪勤的其他基金
基于分式调比的新非二次模型算法研究
- 批准号:11071117
- 批准年份:2010
- 资助金额:29.0 万元
- 项目类别:面上项目
移动渐近线和锥模型信赖域方法的研究
- 批准号:10471062
- 批准年份:2004
- 资助金额:10.0 万元
- 项目类别:面上项目
非线性最优化直接搜索法研究及其在气动优化设计的应用
- 批准号:10071037
- 批准年份:2000
- 资助金额:10.0 万元
- 项目类别:面上项目
稠密和稀疏大规模非线性最优化理论、方法和应用软件
- 批准号:19771047
- 批准年份:1997
- 资助金额:5.5 万元
- 项目类别:面上项目
相似国自然基金
{{ item.name }}
- 批准号:{{ item.ratify_no }}
- 批准年份:{{ item.approval_year }}
- 资助金额:{{ item.support_num }}
- 项目类别:{{ item.project_type }}
相似海外基金
{{
item.name }}
{{ item.translate_name }}
- 批准号:{{ item.ratify_no }}
- 财政年份:{{ item.approval_year }}
- 资助金额:{{ item.support_num }}
- 项目类别:{{ item.project_type }}