高维约束矩阵回归的优化理论与算法
项目介绍
AI项目解读
基本信息
- 批准号:11671029
- 项目类别:面上项目
- 资助金额:48.0万
- 负责人:
- 依托单位:
- 学科分类:A0405.连续优化
- 结题年份:2020
- 批准年份:2016
- 项目状态:已结题
- 起止时间:2017-01-01 至2020-12-31
- 项目参与者:孙捷; 孟庆民; 翟怀远; 苏之华; 刘延青; 潘丽丽; 樊军; 修贤超; 陈丙振;
- 关键词:
项目摘要
High-dimensional constrained matrix regression refers to non-convex constraint statistical regression with the multivariate responses and multivariate predictors in the higher-dimensional setting. Its mathematical model is a matrix optimization, which is NP-hard and has a wide range of applications in areas such as machine learning and artificial intelligence, gene expression analysis, neural networks, medical imaging and diagnosis, risk management. This project focuses on optimization theory and algorithm of high-dimensional constrained matrix regression, which mainly contents the following parts: Firstly, we consider the statistical and optimization properties of high-dimensional matrix regression with equation, low-rank or sparse constraints and its various relaxations, and established high-dimensional model selection and parameter selection criteria and optimal conditions, which guarantee to solve relaxation problem easily and get the approximate effective errors; Secondly, we propose algorithms and give their convergent analyses which should be applied to solve large-scale matrix optimizations with fast and stable performance; Finally, we give numerical experiment and its application in medical imaging and diagnosis, and propose the practically useful programming. This projection will promote the cross integration between statistical and mathematical branches in operations research , and is valuable for rapid development of statistical optimization. Then it provides the theoretical and algorithmic foundations for solving problems in practice.
高维约束矩阵回归是指高维情况下带非凸约束的多响应多预测统计回归问题,其数学模型是一个NP-难的矩阵优化,它在机器学习与人工智能、医学影像疾病诊疗、基因表达分析、脑神经网络、风险管理等领域有广泛应用。本项目欲开展高维约束矩阵回归的优化理论与算法研究,主要内容包括: 探讨高维情况下带有等式和低秩稀疏等非凸约束的矩阵回归及各种松弛优化模型的统计和优化性质, 建立高维数据的模型选择标准和参数选取理论,以期给出各种矩阵优化模型的适用条件,保证松弛问题易求解、近似效果好且误差可控;进行算法设计与理论分析, 以期得到收敛速度快、稳定性能好、适合求解大规模矩阵优化问题的算法;进行数值实验及在医学影像疾病诊疗中的应用研究,以期得到具有实用价值的计算程序。本研究能促进统计学等多学科领域在最优化的融合与交叉,有利于统计优化的快速发展,为解决实际问题提供理论与算法支撑。
结项摘要
高维约束矩阵回归是指高维情况下带非凸约束的多响应多预测统计回归问题,其数学模型是一个NP-难的矩阵优化,它在机器学习与人工智能、医学影像疾病诊疗、基因表达分析、脑神经网络、风险管理等领域有广泛应用。本项目开展高维约束矩阵回归的优化理论与算法研究,主要内容包括: 探讨高维情况下带有等式和低秩稀疏等非凸约束的矩阵回归及各种松弛优化模型的统计和优化性质。针对高维统计模型,研究其对偶理论、一阶/二阶最优性条件、模型自由度、模型参数选择等,并分析模型的统计性质。在算法设计方面,针对凸/非凸稀疏优化模型,利用数据的结构特征,设计快速有效的优化算法,并探讨算法的收敛性分析。在实际应用方面,结合本项目所提出的模型和算法,将它们应用于视频监控的前景提取、工业过程的故障检测、磁共振成像的异常分析、人脸识别的特征选择等领域,进行数值实验及在医学影像疾病诊疗中的应用研究,得到了具有实用价值的计算程序。本研究促进统计学等多学科领域在最优化的融合与交叉,有利于统计优化的快速发展,为解决实际问题提供了理论与算法支撑。
项目成果
期刊论文数量(23)
专著数量(0)
科研奖励数量(0)
会议论文数量(0)
专利数量(0)
The linearized alternating direction method of multipliers for low-rank and fused LASSO matrix regression model
低秩融合LASSO矩阵回归模型乘子线性化交替方向法
- DOI:10.1080/02664763.2020.1742296
- 发表时间:2020-03
- 期刊:Journal of Applied Statistics
- 影响因子:1.5
- 作者:Li Mei
- 通讯作者:Li Mei
AN IMPROVED TOTAL VARIATION REGULARIZED RPCA FOR MOVING OBJECT DETECTION WITH DYNAMIC BACKGROUND
一种改进的全变差正则化RPCA动态背景运动目标检测
- DOI:10.3934/jimo.2019024
- 发表时间:2020
- 期刊:Journal of Industrial and Management Optimization
- 影响因子:1.3
- 作者:Xiu Xianchao;Yang Ying;Liu Wanquan;Kong Lingchen;Shang Meijuan
- 通讯作者:Shang Meijuan
Face recognition based on manifold constrained joint sparse sensing with K-SVD
基于K-SVD流形约束联合稀疏感知的人脸识别
- DOI:10.1007/s11042-018-6071-9
- 发表时间:2018-11
- 期刊:Multimedia Tools and Applications
- 影响因子:3.6
- 作者:Jingjing Liu
- 通讯作者:Jingjing Liu
High-dimensional least square matrix regression via elastic net penalty
通过弹性净惩罚的高维最小二乘矩阵回归
- DOI:--
- 发表时间:2017
- 期刊:Pacific Journal of Optimization
- 影响因子:0.2
- 作者:Bingzhen Chen;Lingchen Kong
- 通讯作者:Lingchen Kong
The l-1-penalized quantile regression for traditional chinese medicine syndrome manifestation
中医证候表现的l-1惩罚分位数回归
- DOI:--
- 发表时间:2017
- 期刊:Pacific Journal of Optimization
- 影响因子:0.2
- 作者:Liu Yanqing;Liu Guokai;Xiu Xianchao;Zhou Shenglong
- 通讯作者:Zhou Shenglong
数据更新时间:{{ journalArticles.updateTime }}
{{
item.title }}
{{ item.translation_title }}
- DOI:{{ item.doi || "--"}}
- 发表时间:{{ item.publish_year || "--" }}
- 期刊:{{ item.journal_name }}
- 影响因子:{{ item.factor || "--"}}
- 作者:{{ item.authors }}
- 通讯作者:{{ item.author }}
数据更新时间:{{ journalArticles.updateTime }}
{{ item.title }}
- 作者:{{ item.authors }}
数据更新时间:{{ monograph.updateTime }}
{{ item.title }}
- 作者:{{ item.authors }}
数据更新时间:{{ sciAawards.updateTime }}
{{ item.title }}
- 作者:{{ item.authors }}
数据更新时间:{{ conferencePapers.updateTime }}
{{ item.title }}
- 作者:{{ item.authors }}
数据更新时间:{{ patent.updateTime }}
其他文献
Some new results for Z-transformation on the Lorentz Cone
洛伦兹锥 Z 变换的一些新结果
- DOI:--
- 发表时间:2015
- 期刊:Pacific Journal of Optimization
- 影响因子:0.2
- 作者:孔令臣;J. Tao;罗自炎;修乃华
- 通讯作者:修乃华
坚持本研一体化,加强统计优化课程建设
- DOI:--
- 发表时间:2022
- 期刊:创新教育研究
- 影响因子:--
- 作者:孔令臣
- 通讯作者:孔令臣
对称锥互补问题的一个惩罚NR函数(英文)
- DOI:--
- 发表时间:--
- 期刊:数学进展
- 影响因子:--
- 作者:孔令臣;修乃华;秦林霞;KONG Lingchen~*,XIU Naihua~(**),QIN Linxia~(***) (
- 通讯作者:KONG Lingchen~*,XIU Naihua~(**),QIN Linxia~(***) (
半定矩阵秩极小的非凸精确松弛
- DOI:--
- 发表时间:2013
- 期刊:应用数学学报
- 影响因子:--
- 作者:秦林霞;修乃华;孔令臣
- 通讯作者:孔令臣
其他文献
{{
item.title }}
{{ item.translation_title }}
- DOI:{{ item.doi || "--" }}
- 发表时间:{{ item.publish_year || "--"}}
- 期刊:{{ item.journal_name }}
- 影响因子:{{ item.factor || "--" }}
- 作者:{{ item.authors }}
- 通讯作者:{{ item.author }}
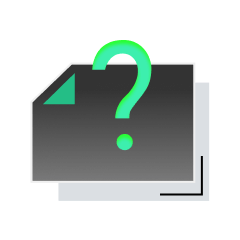
内容获取失败,请点击重试
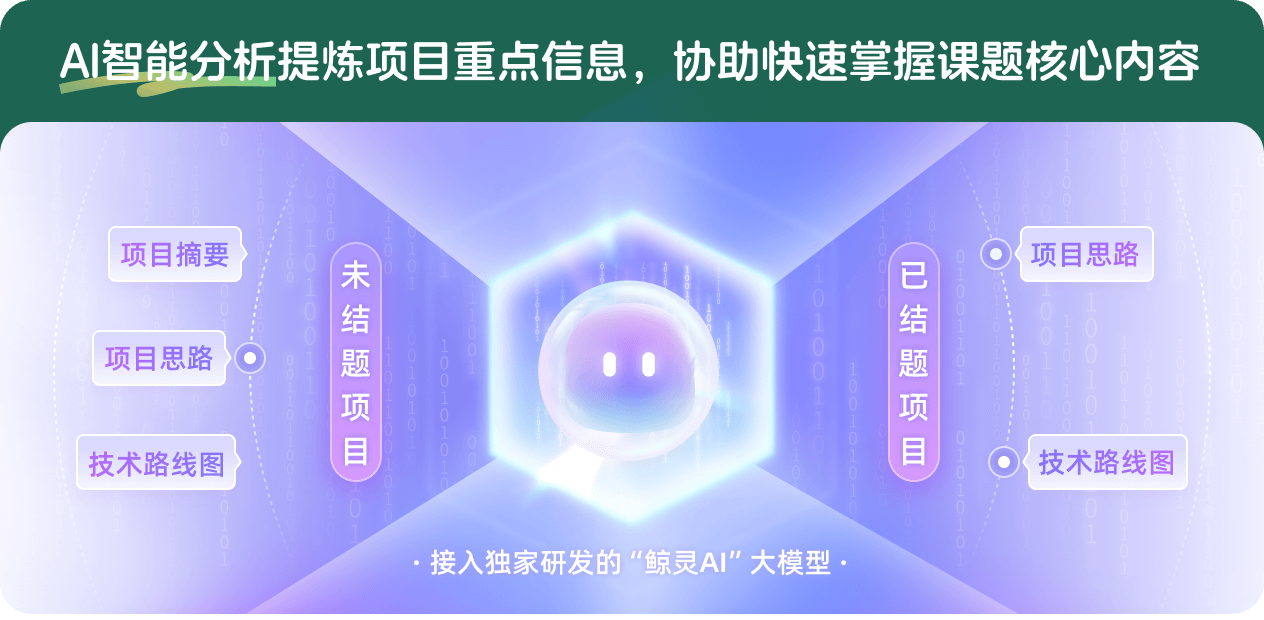
查看分析示例
此项目为已结题,我已根据课题信息分析并撰写以下内容,帮您拓宽课题思路:
AI项目摘要
AI项目思路
AI技术路线图
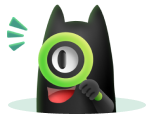
请为本次AI项目解读的内容对您的实用性打分
非常不实用
非常实用
1
2
3
4
5
6
7
8
9
10
您认为此功能如何分析更能满足您的需求,请填写您的反馈:
孔令臣的其他基金
高维稳健隐私回归的优化模型理论与算法研究
- 批准号:12371322
- 批准年份:2023
- 资助金额:43.5 万元
- 项目类别:面上项目
统计优化与学习天元数学交流项目
- 批准号:12126201
- 批准年份:2021
- 资助金额:20.0 万元
- 项目类别:数学天元基金项目
统计优化与人工智能天元数学交流项目
- 批准号:12026201
- 批准年份:2020
- 资助金额:20.0 万元
- 项目类别:数学天元基金项目
机器学习与优化天元数学交流项目
- 批准号:11826004
- 批准年份:2018
- 资助金额:29.0 万元
- 项目类别:数学天元基金项目
矩阵秩极小问题的松弛理论与算法研究
- 批准号:11171018
- 批准年份:2011
- 资助金额:43.0 万元
- 项目类别:面上项目
相似国自然基金
{{ item.name }}
- 批准号:{{ item.ratify_no }}
- 批准年份:{{ item.approval_year }}
- 资助金额:{{ item.support_num }}
- 项目类别:{{ item.project_type }}
相似海外基金
{{
item.name }}
{{ item.translate_name }}
- 批准号:{{ item.ratify_no }}
- 财政年份:{{ item.approval_year }}
- 资助金额:{{ item.support_num }}
- 项目类别:{{ item.project_type }}