白介素21受体在肺动脉高压肺血管重构中的作用及机制
项目介绍
AI项目解读
基本信息
- 批准号:81970046
- 项目类别:面上项目
- 资助金额:55万
- 负责人:
- 依托单位:
- 学科分类:H0109.急性肺损伤和急性呼吸窘迫综合征
- 结题年份:
- 批准年份:2019
- 项目状态:未结题
- 起止时间:2019至
- 项目参与者:王涛;
- 关键词:
项目摘要
结项摘要
项目成果
期刊论文数量(0)
专著数量(0)
科研奖励数量(0)
会议论文数量(0)
专利数量(0)
数据更新时间:{{ journalArticles.updateTime }}
{{
item.title }}
{{ item.translation_title }}
- DOI:{{ item.doi || "--"}}
- 发表时间:{{ item.publish_year || "--" }}
- 期刊:{{ item.journal_name }}
- 影响因子:{{ item.factor || "--"}}
- 作者:{{ item.authors }}
- 通讯作者:{{ item.author }}
数据更新时间:{{ journalArticles.updateTime }}
{{ item.title }}
- 作者:{{ item.authors }}
数据更新时间:{{ monograph.updateTime }}
{{ item.title }}
- 作者:{{ item.authors }}
数据更新时间:{{ sciAawards.updateTime }}
{{ item.title }}
- 作者:{{ item.authors }}
数据更新时间:{{ conferencePapers.updateTime }}
{{ item.title }}
- 作者:{{ item.authors }}
数据更新时间:{{ patent.updateTime }}
其他文献
敲除背根神经节Fcgr1减弱类风湿关节炎模型大鼠NF-kB/NLRP3通路活化
- DOI:10.3969/j.issn.1001-6325.2021.07.006
- 发表时间:2021
- 期刊:基础医学与临床
- 影响因子:--
- 作者:刘帆;苏思;王涛;袁勃;马超
- 通讯作者:马超
Numerical analysis of the influence of the fuel injection timing and ignition position in a direct-injection natural gas engine
直喷式天然气发动机喷油正时和点火位置影响的数值分析
- DOI:10.1016/j.enconman.2017.03.004
- 发表时间:2017-10
- 期刊:Energy Conversion and Management
- 影响因子:10.4
- 作者:王涛;张欣;张纪宝;侯效森
- 通讯作者:侯效森
交替协调子结构混合试验方法研究
- DOI:10.6052/j.issn.1000-4750.2018.02.s007
- 发表时间:2019
- 期刊:工程力学
- 影响因子:--
- 作者:杜春波;王涛;郄毅
- 通讯作者:郄毅
Fuzzy adaptive control of multivariable nonlinear systems
多变量非线性系统的模糊自适应控制
- DOI:10.1109/fuzz.2002.1005011
- 发表时间:2002-08
- 期刊:Fuzzy Sets and Systems
- 影响因子:3.9
- 作者:佟绍成;唐涧涛;王涛
- 通讯作者:王涛
局部消融治疗术后单发复发肝细胞癌长期生存研究
- DOI:10.19538/j.cjps.issn1005-2208.2020.06.19
- 发表时间:2020
- 期刊:中国实用外科杂志
- 影响因子:--
- 作者:史瑶平;池嘉昌;施东华;王涛;崔丹;王智;翟博
- 通讯作者:翟博
其他文献
{{
item.title }}
{{ item.translation_title }}
- DOI:{{ item.doi || "--" }}
- 发表时间:{{ item.publish_year || "--"}}
- 期刊:{{ item.journal_name }}
- 影响因子:{{ item.factor || "--" }}
- 作者:{{ item.authors }}
- 通讯作者:{{ item.author }}
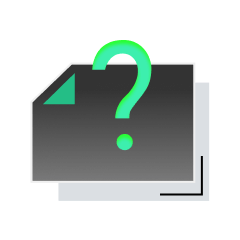
内容获取失败,请点击重试
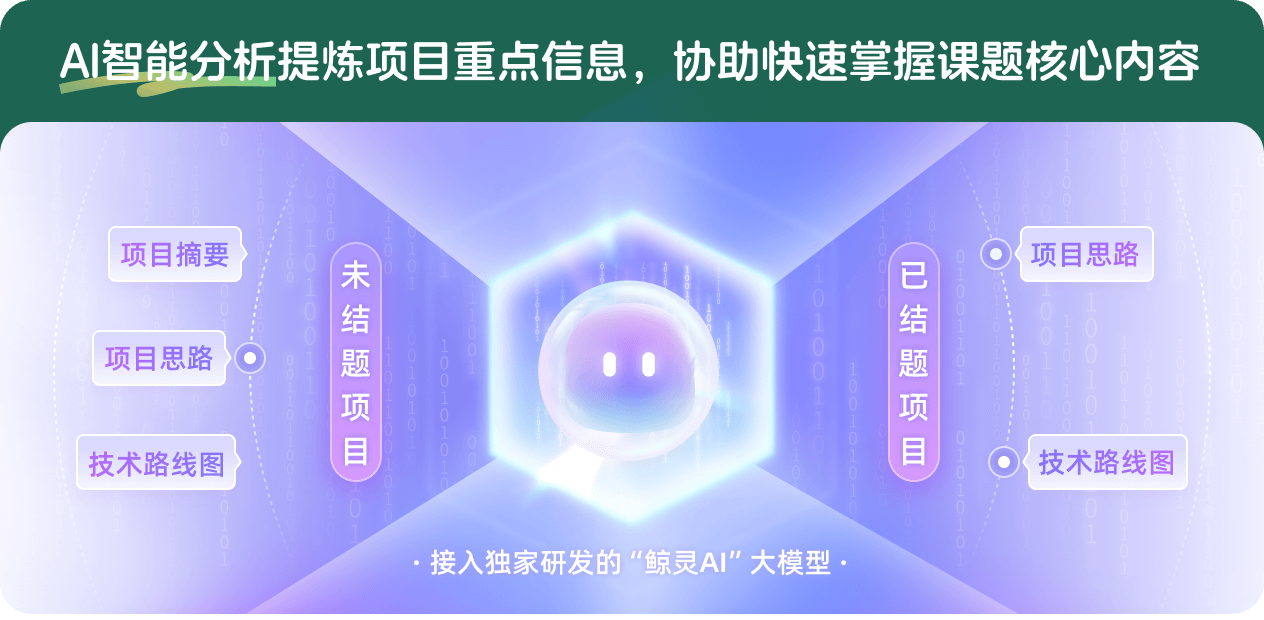
查看分析示例
此项目为未结题,我已根据课题信息分析并撰写以下内容,帮您拓宽课题思路:
AI项目摘要
AI项目思路
AI技术路线图
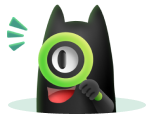
请为本次AI项目解读的内容对您的实用性打分
非常不实用
非常实用
1
2
3
4
5
6
7
8
9
10
您认为此功能如何分析更能满足您的需求,请填写您的反馈:
王涛的其他基金
氧化三甲胺通过PERK上调巨噬细胞炎症因子分泌在肺动脉高压中的作用与机制
- 批准号:82241024
- 批准年份:2022
- 资助金额:52 万元
- 项目类别:面上项目
肺动脉高压的血小板特征及其调控肺血管重塑的机制
- 批准号:
- 批准年份:2022
- 资助金额:50 万元
- 项目类别:
氧化三甲胺通过PERK上调巨噬细胞炎症因子分泌在肺动脉高压中的作用与机制
- 批准号:82270053
- 批准年份:2022
- 资助金额:52.00 万元
- 项目类别:面上项目
白介素21受体在外周动脉疾病中的促新生血管形成作用及其机制的研究
- 批准号:81700426
- 批准年份:2017
- 资助金额:20.0 万元
- 项目类别:青年科学基金项目
相似国自然基金
{{ item.name }}
- 批准号:{{ item.ratify_no }}
- 批准年份:{{ item.approval_year }}
- 资助金额:{{ item.support_num }}
- 项目类别:{{ item.project_type }}
相似海外基金
{{
item.name }}
{{ item.translate_name }}
- 批准号:{{ item.ratify_no }}
- 财政年份:{{ item.approval_year }}
- 资助金额:{{ item.support_num }}
- 项目类别:{{ item.project_type }}