高维数据分析中的收缩估计与似然推断
项目介绍
AI项目解读
基本信息
- 批准号:11571154
- 项目类别:面上项目
- 资助金额:50.0万
- 负责人:
- 依托单位:
- 学科分类:A0402.统计推断与统计计算
- 结题年份:2019
- 批准年份:2015
- 项目状态:已结题
- 起止时间:2016-01-01 至2019-12-31
- 项目参与者:荆炳义; 周望; 严定琪; 胡潇潇; 陈进源; 杨帆; 韦阳; 嵇智; 冯斐;
- 关键词:
项目摘要
High-dimensional data analysis and modeling are hot topics in modern statistics and related fields. This project proposes the shrinkage estimations and likelihood inference for high-dimensional statistical analysis. For the former, motivated by Stein’s unbiased risk estimate (SURE) and empirical Bayes, we will develop a class of novel and innovative shrinkage estimators for high-dimensional mean vector in the heteroscedastic hierarchical normal models with the dependence structure, covariates, sparse and many general error criteria. The optimality properties of these estimators will be carefully studied. For the latter, the project combines the penalized methods, empirical likelihood, and jackknife methods, and introduces the penalized jackknife empirical likelihood methods for the high-dimensional data analysis. It will focus on the study of variable selection and statistical inference problems based on the U-statistics structure estimation equations, canonical correlation analysis, Z-estimate and the general relative error criterion. Again, the project will carefully investigate the asymptotic properties of these methods. Meanwhile, to illustrate the advantages of these methods, we will conduct extensive simulation studies and apply these methods to some real data sets such as image processing, medical science, and compressed sensing. In conclusion, the project will be expected to not only have broaden impact on the theory of SURE, empirical Bayes and empirical likelihood, but also provide more robust, more accurate, less computationally intensive methods to scientific fields such as image processing and bioinformatics.
高维数据分析与建模是目前统计及相关领域研究的热点。本项目拟结合收缩估计和似然推断两方面研究高维数据的统计分析。对于前者,我们将基于Stein无偏风险估计 (SURE) 与经验贝叶斯的思想,构建异方差多元正态模型在分量相依、存在协变量、稀疏以及非均方误差准则下高维均值向量的收缩估计,在一定条件下获得估计的最优性和渐近性质;对于后者,本项目将综合应用惩罚经验似然与刀切法,提出高维惩罚刀切经验似然法,研究基于U-统计量结构估计方程、典型相关分析、Z-估计和广义相对误差准则下的变量选择和推断问题,将证明所提方法的极限性质。同时,本项目将通过模拟研究对这些方法与已有方法进行比较,以展现所提方法的优势。这些新方法将被应用于分析一些图像、医学、压缩传感的实际数据。通过本项目研究,可望在理论上丰富SURE、经验贝叶斯和经验似然的研究,同时也拓宽其应用领域,为图像处理、生物信息等领域提供更稳健、有效的方法。
结项摘要
高维数据的统计分析在生物、经济、环境等领域有着广泛的应用背景。本项目基于经验贝叶斯、Stein无偏风险估计、经验似然等方法,对高维数据中的同时估计与统计推断问题展开了方法论的研究。我们研究了:异质(异源)一维正态总体的均值的大规模同时估计,在方差不同且未知的情形下,提出了双收缩SURE估计;具有一般协方差结构的异质多维正态总体,建立了均值向量的同时估计;高维数据的聚类问题,提出了基于收缩估计和学习向量量化算法的增强K均值聚类法;结合SURE估计与线性分类器,提出了更准确的高维分类算法;异质Pareto总体中参数的同时估计,建立了风险参数的同时估计;广义相对误差估计理论,构建了乘法回归模型中增维参数的经验似然置信区间;构造了线性回归模型中误差项方差的刀切经验似然置信区间;Lorenz占优的检验问题,提出了Lorenz曲线差值的刀切经验似然推断方法;构建了高维数据情形下,非光滑U-型估计函数中参数的置信区间;基于U-型估计方程的变量选择和估计问题,提出了惩罚刀切经验似然方法;考虑了选择后推断问题,建立了基于惩罚经验似然的选择后推断方法。对以上估计和检验,我们证明了相应的渐近性质,进行了大量的模拟研究并应用于一些医学研究、环境、保险等领域实际数据的分析。这些方法是对经验贝叶斯、收缩估计理论和经验似然方法的丰富和发展,也为生物、医学、金融、经济等领域的科学研究和实际应用提供了可靠的方法支持和理论指导。
项目成果
期刊论文数量(7)
专著数量(0)
科研奖励数量(0)
会议论文数量(0)
专利数量(0)
Improving K-means method via shrinkage estimation and LVQ algorithm
通过收缩估计和 LVQ 算法改进 K-means 方法
- DOI:10.1080/03610918.2019.1620274
- 发表时间:2019-05
- 期刊:Communications in Statistics - Simulation and Computation
- 影响因子:--
- 作者:Li Zhouping;Wang Hui
- 通讯作者:Wang Hui
Empirical likelihood and general relative error criterion with divergent dimension
发散维数的经验似然和一般相对误差准则
- DOI:10.1080/02331888.2017.1296443
- 发表时间:2017-03
- 期刊:Statistics
- 影响因子:1.9
- 作者:Li Zhouping;Liu Yiming;Liu Zhi
- 通讯作者:Liu Zhi
Jackknife empirical likelihood for the error variance in linear models
线性模型中误差方差的刀切经验似然
- DOI:10.1080/10485252.2017.1285028
- 发表时间:2017-02
- 期刊:Journal of Nonparametric Statistics
- 影响因子:1.2
- 作者:Lin Hui Ling;Li Zhouping;Wang Dongliang;Zhao Yichuan
- 通讯作者:Zhao Yichuan
On SURE-Type Double Shrinkage Estimation
关于SURE型双收缩率估计
- DOI:--
- 发表时间:2015
- 期刊:Journal of the American Statistical Association
- 影响因子:3.7
- 作者:Jing, Bing-Yi;Li,Zhouping;Pan, Guangming;Zhou, Wang
- 通讯作者:Zhou, Wang
增维非光滑估计方程的刀切经验似然方法
- DOI:--
- 发表时间:2019
- 期刊:中国科学:数学
- 影响因子:--
- 作者:韦阳;李周平;杨帆
- 通讯作者:杨帆
数据更新时间:{{ journalArticles.updateTime }}
{{
item.title }}
{{ item.translation_title }}
- DOI:{{ item.doi || "--"}}
- 发表时间:{{ item.publish_year || "--" }}
- 期刊:{{ item.journal_name }}
- 影响因子:{{ item.factor || "--"}}
- 作者:{{ item.authors }}
- 通讯作者:{{ item.author }}
数据更新时间:{{ journalArticles.updateTime }}
{{ item.title }}
- 作者:{{ item.authors }}
数据更新时间:{{ monograph.updateTime }}
{{ item.title }}
- 作者:{{ item.authors }}
数据更新时间:{{ sciAawards.updateTime }}
{{ item.title }}
- 作者:{{ item.authors }}
数据更新时间:{{ conferencePapers.updateTime }}
{{ item.title }}
- 作者:{{ item.authors }}
数据更新时间:{{ patent.updateTime }}
其他文献
一种结构类型可调的复杂输运网络演化模型及仿真
- DOI:--
- 发表时间:2014
- 期刊:小型微型计算机系统
- 影响因子:--
- 作者:李周平;杨坚争;韩景倜
- 通讯作者:韩景倜
基于复杂分层网络的城际路网级联失效可靠性仿真
- DOI:--
- 发表时间:2015
- 期刊:计算机应用研究
- 影响因子:--
- 作者:李周平;韩景倜;肖宇
- 通讯作者:肖宇
基于分层复杂网络的城际路网空间结构特征
- DOI:--
- 发表时间:2014
- 期刊:公路交通科技
- 影响因子:--
- 作者:李周平;韩景倜;杨坚争;肖宇
- 通讯作者:肖宇
其他文献
{{
item.title }}
{{ item.translation_title }}
- DOI:{{ item.doi || "--" }}
- 发表时间:{{ item.publish_year || "--"}}
- 期刊:{{ item.journal_name }}
- 影响因子:{{ item.factor || "--" }}
- 作者:{{ item.authors }}
- 通讯作者:{{ item.author }}
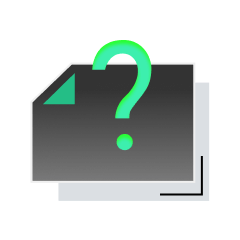
内容获取失败,请点击重试
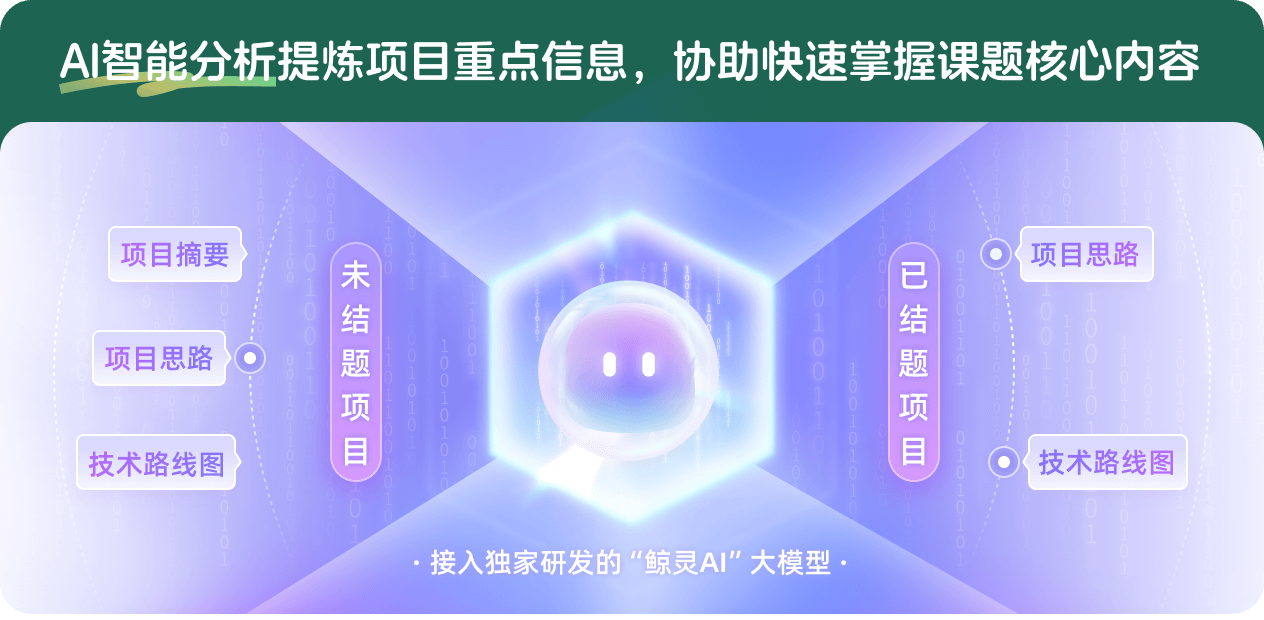
查看分析示例
此项目为已结题,我已根据课题信息分析并撰写以下内容,帮您拓宽课题思路:
AI项目摘要
AI项目思路
AI技术路线图
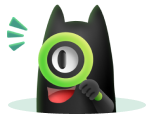
请为本次AI项目解读的内容对您的实用性打分
非常不实用
非常实用
1
2
3
4
5
6
7
8
9
10
您认为此功能如何分析更能满足您的需求,请填写您的反馈:
李周平的其他基金
基于刀切经验似然方法的有效统计推断
- 批准号:11201207
- 批准年份:2012
- 资助金额:22.0 万元
- 项目类别:青年科学基金项目
相似国自然基金
{{ item.name }}
- 批准号:{{ item.ratify_no }}
- 批准年份:{{ item.approval_year }}
- 资助金额:{{ item.support_num }}
- 项目类别:{{ item.project_type }}
相似海外基金
{{
item.name }}
{{ item.translate_name }}
- 批准号:{{ item.ratify_no }}
- 财政年份:{{ item.approval_year }}
- 资助金额:{{ item.support_num }}
- 项目类别:{{ item.project_type }}