面向众包标注的真值推断与监督分类关键问题研究
项目介绍
AI项目解读
基本信息
- 批准号:61603186
- 项目类别:青年科学基金项目
- 资助金额:22.0万
- 负责人:
- 依托单位:
- 学科分类:F0603.机器学习
- 结题年份:2019
- 批准年份:2016
- 项目状态:已结题
- 起止时间:2017-01-01 至2019-12-31
- 项目参与者:夏彬; 倪铭; 吴铭; 许小强; 崔诗程; 陈晗婧; 王笑;
- 关键词:
项目摘要
Recently, data annotation tasks can be completed by ordinary Internet users through crowdsourcing platforms. Since labeling qualities of labelers in crowdsourcing are different, supervised learning from these crowdsourced labeled data is full of challenges. This proposal focuses on ground truth inference and supervised learning for crowdsourcing from the aspect of machine learning, providing novel theories and methods for building supervised classification learning systems based on crowdsourced labeled data. Based on the classic statistical-query learnable theory and considering the specific characteristics of crowdsourcing, we first study the relationship among sample features, classifiers, labeling qualities, the performance of ground truth inference algorithms, and the qualities of learned models, and then build up a set of fundamental theories which can supervise ground truth inference and model training. Secondly, in order to improve the quality of integrated labels, we study novel algorithms for multi-class ground truth inference which are based on the fusion of concept-level features and physical-level features of examples, and novel methods which can correct integrated mislabeled examples. Thirdly, in order to improve the performance of learned models under an active learning paradigm, we study finer-grain sampling strategies, labeling optimization methods and labeler selection strategies based on temporal modeling for labeling qualities. Finally, we build up a prototype system for ground truth inference and supervised classification, using crowdsourced data to verify practical values of our research outcomes.
近年来,数据标注任务可以方便地通过众包平台由互联网上的普通用户完成。由于众包标注者的标注质量参差不齐,利用众包标注数据进行监督学习模型训练充满挑战。本项目致力于研究众包标注数据机器学习中真值推断与监督分类问题,为基于众包标注数据构建监督分类学习系统提供新理论与新方法。首先,从经典的统计查询可学习理论出发,结合众包标注相关特性,研究预算约束条件下,样本特征、分类器、标注者质量与真值推断性能及学习模型质量之间的关系,建立指导真值推断和模型训练的基础理论;其次,研究基于样本“概念层”与“物理层”特征融合的多分类真值推断算法和“集成误标”样本标签纠正方法,以提高集成标签质量;再次,研究主动学习范式下更加精细的样本选择策略、标签优化方法以及基于标注质量时序建模的标注者选择策略,以提高学习模型的性能。最后,构建面向众包标注的真值推断与监督分类的原型系统,以验证研究成果的应用价值。
结项摘要
众包环境的不确定性使得利用众包标注数据进行机器学习充满挑战。项目聚焦面向众包标注的真值推断与监督分类中的关键问题。首先,研究了众包标签真值推断算法。针对偏置标注,提出适应性加权多数投票推断算法,平衡了标注者对两类样本的投票权重。针对多分类多标签任务,提出基于混合多努利分布的推断算法以发掘并利用标签之间的相关性。针对样本和标签稀疏性,提出单一参数建模标注者质量与样本难度的鲁棒性推断模型。这些算法显著提升了真值推断的准确度。其次,研究了基于标签噪声纠正的标签集成方法。提出迭代双层聚类标签集成算法,通过对概念层和物理层特征进行交叉聚类分析,发现并纠正概念层集成标签中的误标。提出基于模型预测标签噪声纠正的标签集成算法,通过高标签质量样本构建的集成学习模型发现并纠正低标签质量样本中的误标。再次,研究了面向众包标注数据的精细化预测模型学习方法。提出四种众包噪声标签利用方法和基于样本复制的集成学习算法,提升了预测模型的泛化性能。提出三种主动学习样本选择策略,降低了标注成本。最后,以开源软件的形式将项目原型系统中的核心算法和数据向研究社区开放。研究成果推进了人机协同人工智能的发展且具备广泛的应用前景。
项目成果
期刊论文数量(12)
专著数量(0)
科研奖励数量(0)
会议论文数量(6)
专利数量(1)
Improving Crowdsourced Label Quality Using Noise Correction
使用噪声校正提高众包标签质量
- DOI:10.1109/tnnls.2017.2677468
- 发表时间:2018
- 期刊:IEEE Transactions on Neural Networks and Learning Systems
- 影响因子:10.4
- 作者:Jing Zhang(张静);Victor S. Sheng;Tao Li;Xindong Wu
- 通讯作者:Xindong Wu
Ensemble Learning from Crowds
从人群中进行集成学习
- DOI:10.1080/01419870.2018.1485961
- 发表时间:2019
- 期刊:IEEE Transactions on Knowledge and Data Engineering
- 影响因子:8.9
- 作者:Jing Zhang(张静);Min Wu;Victor S. Sheng
- 通讯作者:Victor S. Sheng
Label Noise Correction and Application in Crowdsourcing
标签噪声校正及其在众包中的应用
- DOI:--
- 发表时间:2016
- 期刊:Expert Systems with Applications
- 影响因子:8.5
- 作者:Bryce Nicholson;Victor S. Sheng;Jing Zhang
- 通讯作者:Jing Zhang
Weak-Labeled Active Learning With Conditional Label Dependence for Multilabel Image Classification
具有条件标签依赖性的弱标签主动学习用于多标签图像分类
- DOI:10.1109/tmm.2017.2652065
- 发表时间:2017
- 期刊:IEEE Transactions on Multimedia
- 影响因子:7.3
- 作者:Jian Wu;Shiquan Zhao;Victor S. Sheng;Jing Zhang;Chen Ye;Pengpeng Zhao;Zhiming Cui
- 通讯作者:Zhiming Cui
Active Learning with Label CorrelationExploration for Multi-Label Image Classification
多标签图像分类的标签相关探索主动学习
- DOI:--
- 发表时间:2017
- 期刊:IET Computer Vision
- 影响因子:1.7
- 作者:Jian Wu;Chen Ye;Victor S. Sheng;Jing Zhang;Pengpeng Zhao;Zhiming Cui
- 通讯作者:Zhiming Cui
数据更新时间:{{ journalArticles.updateTime }}
{{
item.title }}
{{ item.translation_title }}
- DOI:{{ item.doi || "--"}}
- 发表时间:{{ item.publish_year || "--" }}
- 期刊:{{ item.journal_name }}
- 影响因子:{{ item.factor || "--"}}
- 作者:{{ item.authors }}
- 通讯作者:{{ item.author }}
数据更新时间:{{ journalArticles.updateTime }}
{{ item.title }}
- 作者:{{ item.authors }}
数据更新时间:{{ monograph.updateTime }}
{{ item.title }}
- 作者:{{ item.authors }}
数据更新时间:{{ sciAawards.updateTime }}
{{ item.title }}
- 作者:{{ item.authors }}
数据更新时间:{{ conferencePapers.updateTime }}
{{ item.title }}
- 作者:{{ item.authors }}
数据更新时间:{{ patent.updateTime }}
其他文献
基于TCGA数据库的肝癌发生关键基因筛选 Identification Key Genes of Hepatocellular Carcinoma Base on TCGA Database
- DOI:10.12677/hjs.2015.41001
- 发表时间:2015-01-02
- 期刊:
- 影响因子:--
- 作者:贾俊君;何宁;张静;姜骊;周燕飞;周琳;郑树森
- 通讯作者:郑树森
A strained Si-channel NMOSFET with low field mobility enhancement of about 140% using a SiGe virtual substrate
A%20strained%20Si-channel%20NMOSFET%20with%20low%20field%20mobility%20enhancement%20of%20about%20140%%20using%20a%20SiGe%20virtual%20substrate
- DOI:--
- 发表时间:2024-09-14
- 期刊:
- 影响因子:--
- 作者:崔伟;唐昭焕;谭开洲;张静;钟怡;胡辉勇;徐世六;李平;胡刚毅
- 通讯作者:胡刚毅
Thermodynamic Optimization of TmCl3-ACl (A = Na, K, Rb, Cs)Phase Diagrams
TmCl3-ACl 的热力学优化·A = Na, K, Rb, Cs·相图
- DOI:--
- 发表时间:2024-09-14
- 期刊:
- 影响因子:--
- 作者:叶信宇;张静;孙益民;王玉;谈珺珺
- 通讯作者:谈珺珺
安多霖对微波辐照致大鼠脑神经元尼氏体损伤的预防作用
- DOI:--
- 发表时间:2011
- 期刊:中国体视学与图像分析
- 影响因子:--
- 作者:彭瑞云;李静;张静;高亚兵;王水明;董霁;赵黎;王少霞;周红梅
- 通讯作者:周红梅
级联倍频过程中谐波场量子噪声压缩的增强
- DOI:--
- 发表时间:2017
- 期刊:量子光学学报
- 影响因子:--
- 作者:张静;王艳芳;李妮;杨荣国
- 通讯作者:杨荣国
其他文献
{{
item.title }}
{{ item.translation_title }}
- DOI:{{ item.doi || "--" }}
- 发表时间:{{ item.publish_year || "--"}}
- 期刊:{{ item.journal_name }}
- 影响因子:{{ item.factor || "--" }}
- 作者:{{ item.authors }}
- 通讯作者:{{ item.author }}
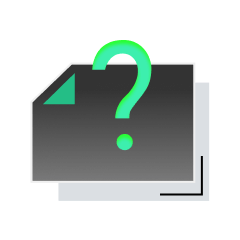
内容获取失败,请点击重试
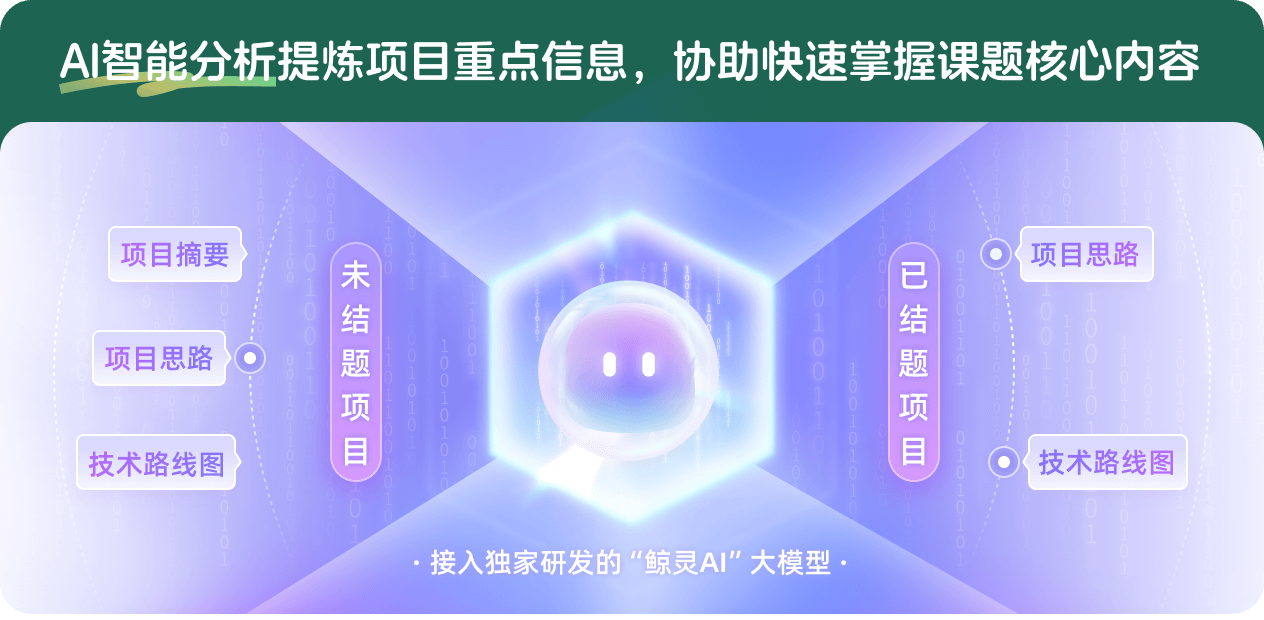
查看分析示例
此项目为已结题,我已根据课题信息分析并撰写以下内容,帮您拓宽课题思路:
AI项目摘要
AI项目思路
AI技术路线图
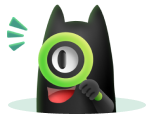
请为本次AI项目解读的内容对您的实用性打分
非常不实用
非常实用
1
2
3
4
5
6
7
8
9
10
您认为此功能如何分析更能满足您的需求,请填写您的反馈:
张静的其他基金
面向众包标注数据的机器学习方法研究
- 批准号:
- 批准年份:2020
- 资助金额:59 万元
- 项目类别:面上项目
众包大数据多源异构融合与知识学习
- 批准号:91846104
- 批准年份:2018
- 资助金额:43.0 万元
- 项目类别:重大研究计划
相似国自然基金
{{ item.name }}
- 批准号:{{ item.ratify_no }}
- 批准年份:{{ item.approval_year }}
- 资助金额:{{ item.support_num }}
- 项目类别:{{ item.project_type }}
相似海外基金
{{
item.name }}
{{ item.translate_name }}
- 批准号:{{ item.ratify_no }}
- 财政年份:{{ item.approval_year }}
- 资助金额:{{ item.support_num }}
- 项目类别:{{ item.project_type }}