不对称趋势周期分解与随机边界-方法与应用
项目介绍
AI项目解读
基本信息
- 批准号:71601129
- 项目类别:青年科学基金项目
- 资助金额:15.0万
- 负责人:
- 依托单位:
- 学科分类:G0105.管理统计理论与方法
- 结题年份:2019
- 批准年份:2016
- 项目状态:已结题
- 起止时间:2017-01-01 至2019-12-31
- 项目参与者:Richard Startz; 王泓仁; 罗穗; 张恪瑜; 闵雨晨;
- 关键词:
项目摘要
Assessing long-term trend and short-term fluctuation is important for macroeconomics researchers and policy makers. If the trend-cycle decomposition we used better captures the characteristics of data, the closer the decomposition will reflect the real economic situation, and the more suitable the economic policies will be. In this project, we extend existing trend-cycle decomposition to allow widely-recognized asymmetry in the business cycle. The form of asymmetry we adopt originates from the stochastic frontier models, which is usually adopted to assess production efficiency of individual firms. We find similarity between the description of trend/cycle in macroeconomics and frontier/inefficiency in stochastic frontier models. However, difference between micro and macro data prevents us from directly applying stochastic frontier model to decompose trend and cycle. In this project, we first adjust stochastic frontier models by allowing for serial correlation, based upon which we build our basic models. Next, we build our extended models by allowing for fat-tail phenomenon and asymmetry often observed in financial data. Because the models we focus on cannot be dealt with using traditional maximum likelihood estimation, we estimate all models by Bayesian approach. We will analyze China macroeconomic data in this project, but we believe the models we develop have great potential to be applied to a wide range of economic and financial time series analysis.
衡量经济的长期趋势与短期波动是宏观经济研究与政策制定的重要依据。若使用的趋势周期分解愈贴合数据特性,所得到的结果就愈能描述真事的经济现况,也就能更准确的制定合适的经济政策。在本项目中,我们拓展现有的趋势周期分解,使其允许经济周期的不对称性。我们采用的不对称形式起源于估计个体厂商生产效率常用的随机边界模型。我们发现经济学家对趋势及周期的描述,与随机边界模型中的前沿及具单边偏离性质的无效率,若合符节。然而,微观数据与宏观数据的差异不允许我们直接使用随机边界模型区分趋势与周期。在本项目的基本模型中,我们调整随机边界模型,使其适用于时间序列分析。接下来我们拓展基本模型的应用性,允许金融数据常见的肥尾现象与不对称性。由于我们关心的模型均无法使用传统的最大似然估计法处理,我们利用贝叶斯方法估计所有模型。我们将分析中国宏观数据的趋势周期性质,但本项目发展出的模型有潜力可被利用在更广泛的经济金融时间序列。
结项摘要
在本项目中,我们拓展现有的趋势周期分解,使其允许经济周期的不对称性。我们采用的不对称形式起源于估计个体厂商生产效率常用的随机边界模型。在本项目的基本模型中,我们调整随机边界模型,使其适用于时间序列分析。接下来我们拓展基本模型的应用性,允许金融数据常见的肥尾现象与不对称性。我們成功的推出基本模型的马尔可夫链蒙特卡罗估計法,並寫出多篇論文發表于国际期刊。其中最重要的成果已接受發表于国际顶级期刊Journal of Money, Credit & Banking。在这篇文章中,我们发现股票价格包含了很多关于不确定性的信息。特别是月度的最大和最小股票收益率包含了和平均收益率不同的细信息。我们在计量模型中引入不对称分布,因为最大和最小股票收益率具有极强的偏度。此分布就是本计划研究的重点:偏正态分布。此举在不确定性的文献也属首创。我们相信利用更符合实际的分布假设可以让我们取得更精确的不确定性估计值。新的不确定性指标可以分辨良性与恶性的不确定性,而我们研究指出恶性的不确定性才是造成经济迟滞的主要因素。这项发现有极大的政策与实际意义,政策制定者需要关注恶性的不确定性以提前防范经济体系的迟滞,而一般大众需要关注恶性的不确定性以避免经济损失。
项目成果
期刊论文数量(6)
专著数量(0)
科研奖励数量(0)
会议论文数量(0)
专利数量(0)
Potential output and inflation dynamics after the Great Recession
大衰退后的潜在产出和通货膨胀动态
- DOI:--
- 发表时间:2018
- 期刊:Empirical Economics
- 影响因子:3.2
- 作者:Yu-Fan Huang;Sui Luo
- 通讯作者:Sui Luo
Dynamic responses of real output to financial spreads
实际产出对金融利差的动态响应
- DOI:--
- 发表时间:2019
- 期刊:International Review of Economics & Finance
- 影响因子:4.5
- 作者:Yu-Fan Huang
- 通讯作者:Yu-Fan Huang
Can Stock Volatility Be Benign? New Measurements and Macroeconomic Implications
股票波动可以是良性的吗?
- DOI:10.2139/ssrn.3085470
- 发表时间:--
- 期刊:Journal of Money, Credit and Banking
- 影响因子:--
- 作者:Yu-Fan Huang;Sui Luo
- 通讯作者:Sui Luo
Flexible panel stochastic frontier model with serially correlated errors
具有序列相关误差的灵活面板随机前沿模型
- DOI:--
- 发表时间:2018
- 期刊:Economics Letters
- 影响因子:2
- 作者:Yu-Fan Huang;Sui Luo;Hung-Jen Wang
- 通讯作者:Hung-Jen Wang
An Efficient Exact Bayesian Method for State Space Models with Stochastic Volatility
具有随机波动性的状态空间模型的高效精确贝叶斯方法
- DOI:--
- 发表时间:--
- 期刊:Studies in Nonlinear Dynamics & Econometrics
- 影响因子:0.8
- 作者:Yu-Fan Huang
- 通讯作者:Yu-Fan Huang
数据更新时间:{{ journalArticles.updateTime }}
{{
item.title }}
{{ item.translation_title }}
- DOI:{{ item.doi || "--"}}
- 发表时间:{{ item.publish_year || "--" }}
- 期刊:{{ item.journal_name }}
- 影响因子:{{ item.factor || "--"}}
- 作者:{{ item.authors }}
- 通讯作者:{{ item.author }}
数据更新时间:{{ journalArticles.updateTime }}
{{ item.title }}
- 作者:{{ item.authors }}
数据更新时间:{{ monograph.updateTime }}
{{ item.title }}
- 作者:{{ item.authors }}
数据更新时间:{{ sciAawards.updateTime }}
{{ item.title }}
- 作者:{{ item.authors }}
数据更新时间:{{ conferencePapers.updateTime }}
{{ item.title }}
- 作者:{{ item.authors }}
数据更新时间:{{ patent.updateTime }}
其他文献
其他文献
{{
item.title }}
{{ item.translation_title }}
- DOI:{{ item.doi || "--" }}
- 发表时间:{{ item.publish_year || "--"}}
- 期刊:{{ item.journal_name }}
- 影响因子:{{ item.factor || "--" }}
- 作者:{{ item.authors }}
- 通讯作者:{{ item.author }}
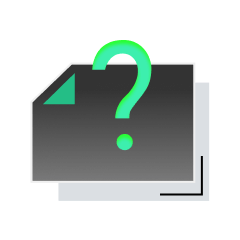
内容获取失败,请点击重试
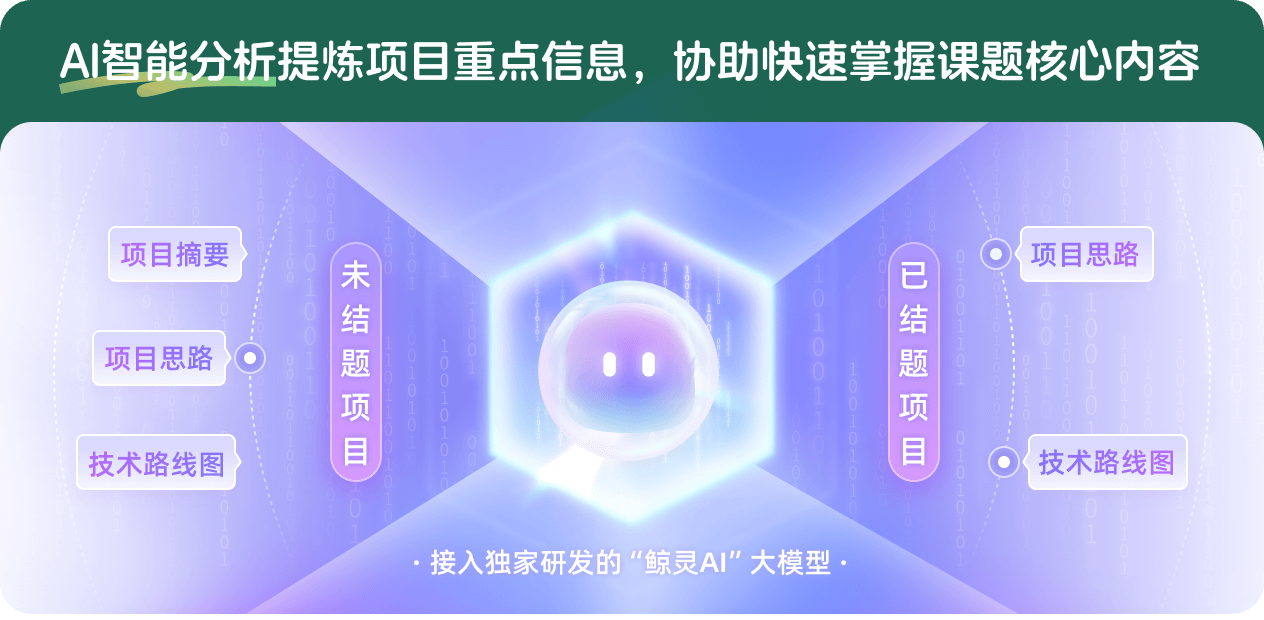
查看分析示例
此项目为已结题,我已根据课题信息分析并撰写以下内容,帮您拓宽课题思路:
AI项目摘要
AI项目思路
AI技术路线图
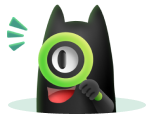
请为本次AI项目解读的内容对您的实用性打分
非常不实用
非常实用
1
2
3
4
5
6
7
8
9
10
您认为此功能如何分析更能满足您的需求,请填写您的反馈:
相似国自然基金
{{ item.name }}
- 批准号:{{ item.ratify_no }}
- 批准年份:{{ item.approval_year }}
- 资助金额:{{ item.support_num }}
- 项目类别:{{ item.project_type }}
相似海外基金
{{
item.name }}
{{ item.translate_name }}
- 批准号:{{ item.ratify_no }}
- 财政年份:{{ item.approval_year }}
- 资助金额:{{ item.support_num }}
- 项目类别:{{ item.project_type }}