硝酸盐转运蛋白OsNRT2.3b参与调控水稻吸收转运菲的机制研究
项目介绍
AI项目解读
基本信息
- 批准号:
- 项目类别:青年科学基金项目
- 资助金额:30万
- 负责人:
- 依托单位:
- 学科分类:
- 结题年份:
- 批准年份:2021
- 项目状态:未结题
- 起止时间:2021至
- 项目参与者:王小文;
- 关键词:
项目摘要
结项摘要
项目成果
期刊论文数量(0)
专著数量(0)
科研奖励数量(0)
会议论文数量(0)
专利数量(0)
数据更新时间:{{ journalArticles.updateTime }}
{{
item.title }}
{{ item.translation_title }}
- DOI:{{ item.doi || "--"}}
- 发表时间:{{ item.publish_year || "--" }}
- 期刊:{{ item.journal_name }}
- 影响因子:{{ item.factor || "--"}}
- 作者:{{ item.authors }}
- 通讯作者:{{ item.author }}
数据更新时间:{{ journalArticles.updateTime }}
{{ item.title }}
- 作者:{{ item.authors }}
数据更新时间:{{ monograph.updateTime }}
{{ item.title }}
- 作者:{{ item.authors }}
数据更新时间:{{ sciAawards.updateTime }}
{{ item.title }}
- 作者:{{ item.authors }}
数据更新时间:{{ conferencePapers.updateTime }}
{{ item.title }}
- 作者:{{ item.authors }}
数据更新时间:{{ patent.updateTime }}
其他文献
2型糖尿病患者肥胖状态与肱踝脉搏波传导速度的相关性
- DOI:10.16462/j.cnki.zhjbkz.2019.09.014
- 发表时间:2019
- 期刊:中华疾病控制杂志
- 影响因子:--
- 作者:黄哲;许蓓蓓;王小文;姚珊珊;曹桂莹;李曼;曹亚英;田耀华;吴瑶;黄少平;刘晓芬;秦雪英;唐迅;李劲;吴涛;陈大方;胡永华
- 通讯作者:胡永华
基于多层次灰色关联分析模型的陕西各市可持续发展能力比较
- DOI:--
- 发表时间:--
- 期刊:四川环境
- 影响因子:--
- 作者:王敏;蒋立荣;徐杰峰;王小文
- 通讯作者:王小文
基于知觉控制的用户界面设计研究
- DOI:--
- 发表时间:--
- 期刊:中国图象图形学报
- 影响因子:--
- 作者:王小文;蔚 娣;李 珍;华庆一;李 倩
- 通讯作者:李 倩
BP人工神经网络在环境空气SO_2质量浓度预测中的应用
- DOI:--
- 发表时间:2014
- 期刊:环境工程
- 影响因子:--
- 作者:韦安磊;王小文;冯舒;张娟娟
- 通讯作者:张娟娟
芳香类有机物生成氯化消毒副产物
- DOI:--
- 发表时间:--
- 期刊:环境科学,2006,27(8):1603-1607
- 影响因子:--
- 作者:王小文;张晓健;陈超;郝小飞
- 通讯作者:郝小飞
其他文献
{{
item.title }}
{{ item.translation_title }}
- DOI:{{ item.doi || "--" }}
- 发表时间:{{ item.publish_year || "--"}}
- 期刊:{{ item.journal_name }}
- 影响因子:{{ item.factor || "--" }}
- 作者:{{ item.authors }}
- 通讯作者:{{ item.author }}
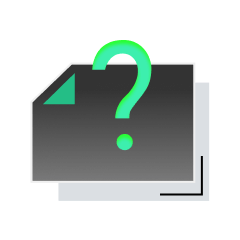
内容获取失败,请点击重试
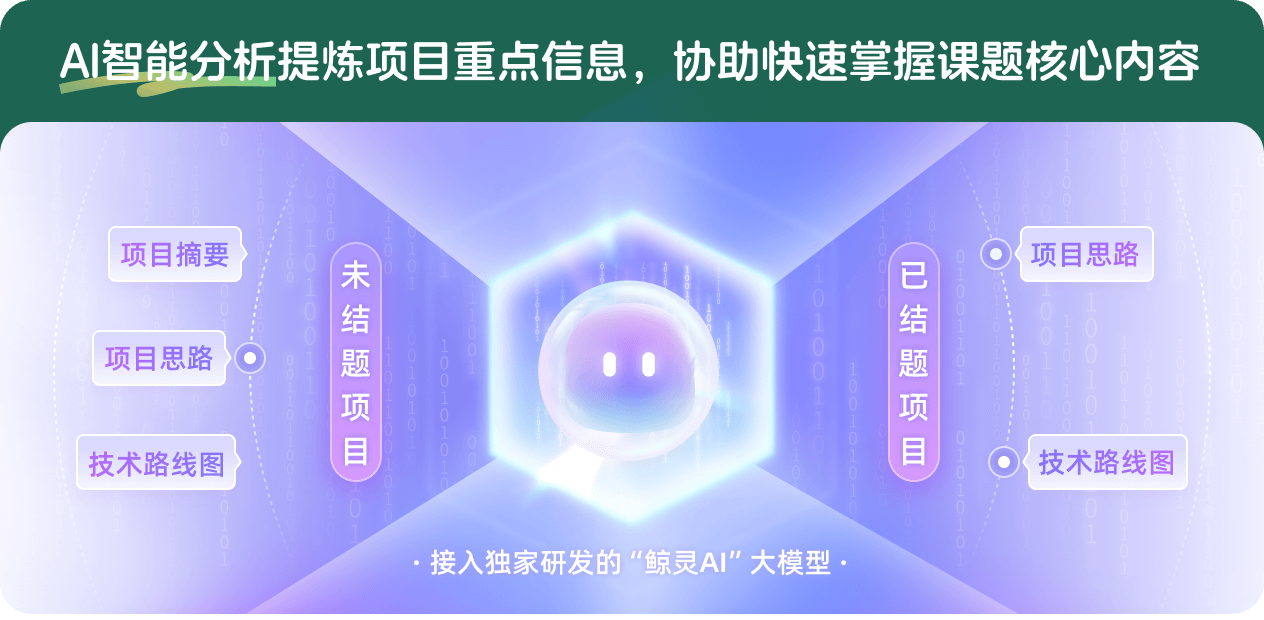
查看分析示例
此项目为未结题,我已根据课题信息分析并撰写以下内容,帮您拓宽课题思路:
AI项目摘要
AI项目思路
AI技术路线图
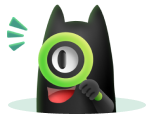
请为本次AI项目解读的内容对您的实用性打分
非常不实用
非常实用
1
2
3
4
5
6
7
8
9
10
您认为此功能如何分析更能满足您的需求,请填写您的反馈:
王小文的其他基金
硝酸盐转运蛋白OsNRT2.3b参与调控水稻吸收转运菲的机制研究
- 批准号:42107428
- 批准年份:2021
- 资助金额:24.00 万元
- 项目类别:青年科学基金项目
相似国自然基金
{{ item.name }}
- 批准号:{{ item.ratify_no }}
- 批准年份:{{ item.approval_year }}
- 资助金额:{{ item.support_num }}
- 项目类别:{{ item.project_type }}
相似海外基金
{{
item.name }}
{{ item.translate_name }}
- 批准号:{{ item.ratify_no }}
- 财政年份:{{ item.approval_year }}
- 资助金额:{{ item.support_num }}
- 项目类别:{{ item.project_type }}