基于多级视觉注意力和可分离相关滤波的在线视觉跟踪研究
项目介绍
AI项目解读
基本信息
- 批准号:61806037
- 项目类别:青年科学基金项目
- 资助金额:22.0万
- 负责人:
- 依托单位:
- 学科分类:F0604.机器感知与机器视觉
- 结题年份:2021
- 批准年份:2018
- 项目状态:已结题
- 起止时间:2019-01-01 至2021-12-31
- 项目参与者:张俊星; 刘俊杰; 刘梓墨; 诸葛云志; 曾怡; 王萌; 杨朔;
- 关键词:
项目摘要
Online visual tracking plays a key role on intelligence video surveillance, and is also a hot and difficult study topic in computer vision and video processing. With respect to effectively mine deep visual features and robustly construct appearance models in object tracking, this project will conduct researches from two perspectives: multi-level visual attention and separated correlation filter models. For the multi-level visual attention model, we will exploit the deep neural networks to model multiple visual attention schemes (spatial, layer, channel and temporal attentions) in a unified framework. This model will online mine deep visual features to facilitate locating the tracked object in current frames, and therefore will improve the representation ability of object appearance. For the separated correlation filter model, we will propose the separation assumption to divide the original filter into the product of a discriminative filter and a reliable filter, and introduce different regularization terms to depict both discrimination and reliability. The global optimization model will be constructed and then be solved using the alternating direction method of multipliers algorithm, which will lead to a robust object appearance model. Finally, we will combine both multi-level visual attention model and separated correlation filter model to develop robust and accurate tracking algorithms. The extensive experiments will be conducted to verify the proposed new theories and algorithms using large-scale standard benchmarks. The findings of this project will support the theoretical study and technical progress for online visual tracking algorithms.
在线视觉跟踪是智能视频监控的关键环节,是计算机视觉和视频处理领域的研究热点和难点。本项目针对跟踪问题中有效深度特征挖掘和鲁棒外观模型建构问题,从多级视觉注意力模型和可分离相关滤波模型两方面展开研究。针对多级视觉注意力模型,拟在深度神经网络中统一建模空间、层、通道、时间等多种视觉注意力机制,在线挖掘有利于当前时刻定位目标的深度视觉特征,提升目标外观特征表达能力;针对可分离相关滤波模型,拟提出可分离假设将原始滤波器拆分为判决性滤波器和可靠性滤波器的乘积,引入不同形式的正则化项来刻画判决性和可靠性属性,建立整体优化模型并利用交替迭代算法求解,构建鲁棒目标外观模型。随后,结合多级视觉注意力模型和可分离相关滤波模型,提出鲁棒精确的在线视觉跟踪算法。本项目最终提出的新理论、新方法将在大尺度标准测评数据库上进行大量的实验验证,研究成果将对在线视觉跟踪算法的理论研究和技术进步提供依据。
结项摘要
在线视觉跟踪是智能视频监控的关键环节,是计算机视觉和视频处理领域的研究热点和难点。本项目针对跟踪问题中有效深度特征挖掘和鲁棒外观模型建构问题,主要从多级视觉注意力模型和可分离相关滤波模型两个方面系统地研究了在线目标跟踪问题,并将研究经验扩展到目标检测和识别领域。项目提出了联合运动和外观信息跟踪模型、多尺度车标检测模型、特征分组多相机多行人模型等有效的跟踪/检测模型及算法,在精度和速度上均取得了较为突出的进展,在多个标准测评中取得了领先的结果。在本项目资助下,课题组共发表论文8篇(SCI检索6篇),其中包括IEEE TIP、IEEE TITS、ICASSP等国际权威期刊及会议。基于上述部分成果,课题组负责人获国家民委中青年英才等奖励。
项目成果
期刊论文数量(7)
专著数量(0)
科研奖励数量(1)
会议论文数量(1)
专利数量(0)
基于UpYOLO的现实场景车标检测算法研究
- DOI:--
- 发表时间:2020
- 期刊:计算机应用研究
- 影响因子:--
- 作者:陈丽君;薄纯娟;张俊星
- 通讯作者:张俊星
Jointly Modeling Motion and Appearance Cues for Robust RGB-T Tracking
联合建模运动和外观线索以实现稳健的 RGB-T 跟踪
- DOI:10.1109/tip.2021.3060862
- 发表时间:2021
- 期刊:IEEE Transactions on Image Processing
- 影响因子:10.6
- 作者:Zhang Pengyu;Zhao Jie;Bo Chunjuan;Wang Dong;Lu Huchuan;Yang Xiaoyun
- 通讯作者:Yang Xiaoyun
A Novel Multi-target Multi-camera Tracking Approach based on Feature Grouping
一种基于特征分组的多目标多摄像机跟踪新方法
- DOI:10.1016/j.compeleceng.2021.107153
- 发表时间:2021
- 期刊:Computers and Electrical Engineering
- 影响因子:4.3
- 作者:Xu Jian;Bo Chunjuan;Wang Dong
- 通讯作者:Wang Dong
Multi-Scale Vehicle Logo Detector
多尺度车辆标志检测器
- DOI:10.1007/s11036-020-01722-0
- 发表时间:2021-02
- 期刊:Multi-Scale Vehicle Logo Detector
- 影响因子:--
- 作者:Zhang Junxing;Chen Lijun;Bo Chunjuan;Yang Shuo
- 通讯作者:Yang Shuo
VLD-45: A Big Dataset for Vehicle Logo Recognition and Detection
VLD-45:车辆标志识别和检测大数据集
- DOI:10.1109/tits.2021.3062113
- 发表时间:2022-12
- 期刊:IEEE Transactions on Intelligent Transportation Systems
- 影响因子:8.5
- 作者:Yang Shuo;Bo Chunjuan;Zhang Junxing;Gao Pengxiang;Li Yujie;Serikawa Seiichi
- 通讯作者:Serikawa Seiichi
数据更新时间:{{ journalArticles.updateTime }}
{{
item.title }}
{{ item.translation_title }}
- DOI:{{ item.doi || "--"}}
- 发表时间:{{ item.publish_year || "--" }}
- 期刊:{{ item.journal_name }}
- 影响因子:{{ item.factor || "--"}}
- 作者:{{ item.authors }}
- 通讯作者:{{ item.author }}
数据更新时间:{{ journalArticles.updateTime }}
{{ item.title }}
- 作者:{{ item.authors }}
数据更新时间:{{ monograph.updateTime }}
{{ item.title }}
- 作者:{{ item.authors }}
数据更新时间:{{ sciAawards.updateTime }}
{{ item.title }}
- 作者:{{ item.authors }}
数据更新时间:{{ conferencePapers.updateTime }}
{{ item.title }}
- 作者:{{ item.authors }}
数据更新时间:{{ patent.updateTime }}
其他文献
其他文献
{{
item.title }}
{{ item.translation_title }}
- DOI:{{ item.doi || "--" }}
- 发表时间:{{ item.publish_year || "--"}}
- 期刊:{{ item.journal_name }}
- 影响因子:{{ item.factor || "--" }}
- 作者:{{ item.authors }}
- 通讯作者:{{ item.author }}
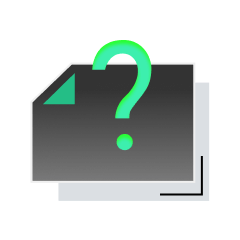
内容获取失败,请点击重试
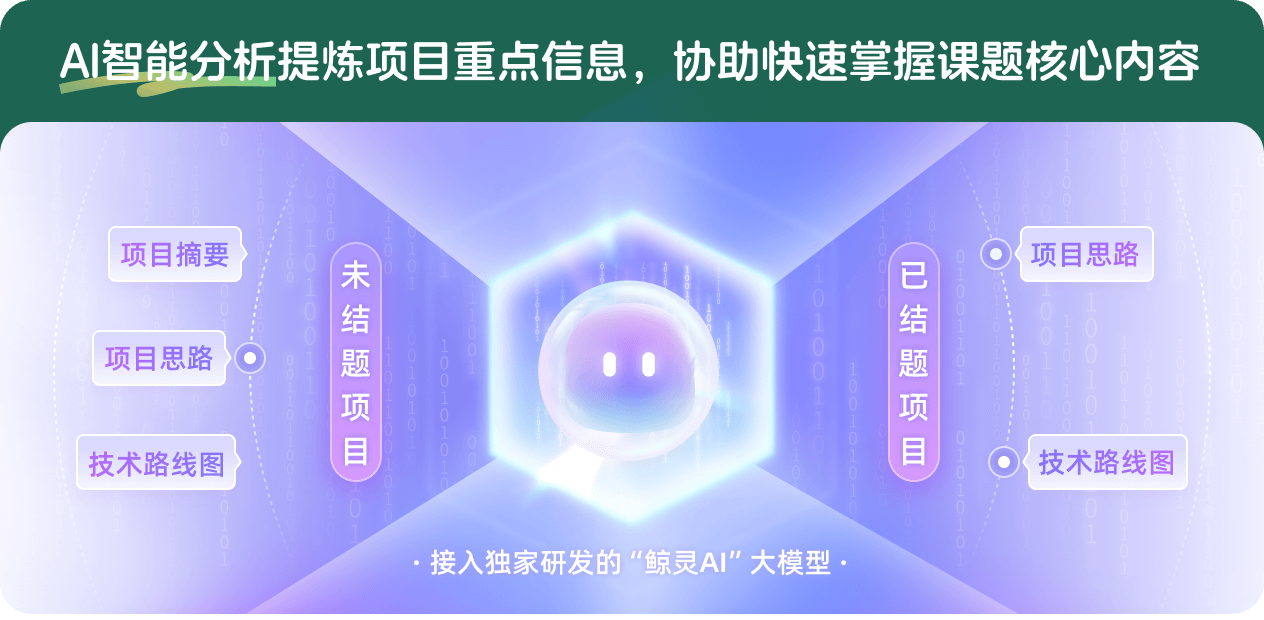
查看分析示例
此项目为已结题,我已根据课题信息分析并撰写以下内容,帮您拓宽课题思路:
AI项目摘要
AI项目思路
AI技术路线图
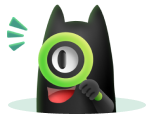
请为本次AI项目解读的内容对您的实用性打分
非常不实用
非常实用
1
2
3
4
5
6
7
8
9
10
您认为此功能如何分析更能满足您的需求,请填写您的反馈:
薄纯娟的其他基金
长时视觉目标跟踪中若干关键问题研究
- 批准号:
- 批准年份:2021
- 资助金额:57 万元
- 项目类别:面上项目
相似国自然基金
{{ item.name }}
- 批准号:{{ item.ratify_no }}
- 批准年份:{{ item.approval_year }}
- 资助金额:{{ item.support_num }}
- 项目类别:{{ item.project_type }}
相似海外基金
{{
item.name }}
{{ item.translate_name }}
- 批准号:{{ item.ratify_no }}
- 财政年份:{{ item.approval_year }}
- 资助金额:{{ item.support_num }}
- 项目类别:{{ item.project_type }}