基于稀疏表达与间隙度描述的纹理分析研究
项目介绍
AI项目解读
基本信息
- 批准号:61602184
- 项目类别:青年科学基金项目
- 资助金额:20.0万
- 负责人:
- 依托单位:
- 学科分类:F0210.计算机图像视频处理与多媒体技术
- 结题年份:2019
- 批准年份:2016
- 项目状态:已结题
- 起止时间:2017-01-01 至2019-12-31
- 项目参与者:黄艳; 罗玉; 廖晓伟; 许若涛; 王树锋; 毛婷伟; 李梦溪;
- 关键词:
项目摘要
Texture is one of the most fundamental features in natural images and videos, and texture analysis is one of the fundamental problems in computer vision, which plays an important role in a lot of computer vision applications. In the past decade, great progress has been made in texture analysis. However, there is still room for improvement in existing methods. This project aims at developing effective texture analysis methods from three aspects: local texture pattern extraction with sparse dictionary learning, global texture feature description using lacunarity analysis, and higher-level texture representation via sparse coding. We propose to develop invariant dictionary learning methods for enhancing the robustness of local texture pattern extraction to viewpoint changes and environmental variations. Regarding the processing of high-resolution data, we propose to develop structured tensor dictionary learning methods, which are expected to reduce the computational cost in local texture pattern extraction. In order to capture the details of spatial and temporal distributions of local texture patterns, we propose to develop global texture features by examining the multi-scale behavior of lacunarity of patterns. In order to effectively extract the higher-level representation from global features of data, we propose to introduce ensemble learning and kernel tricks for developing supervised sparse coding methods which can well handle nonlinearities of data. This project will provide a promising way for texture feature extraction to boost its performance and improve its applicability.
纹理是自然图像和视频的最基本特征之一,而纹理分析一直是计算视觉领域的基本问题,也是众多计算视觉应用的关键环节。纹理分析的研究在过去十年取得了很大进展,但现有方法在性能和实用性方面仍有很大的改进空间。本项目拟从三个方面开展对纹理分析方法的研究:基于稀疏字典学习的纹理局部模式提取方法、基于间隙度分析的全局纹理描述方法和基于稀疏表达的高层次纹理特征提取方法。我们拟提出不变性字典学习方法,以提升局部模式提取对拍摄视角变化及环境影响的鲁棒性。针对高清图像和视频数据,我们拟提出结构化张量字典学习方法,以减少局部模式提取的计算开销。为捕捉局部模式的空间和时间布局细节,我们拟通过分析局部模式间隙度的多尺度行为构建全局纹理特征。为有效提取高层次纹理特征,我们拟引入集成学习机制和核方法,提出能高效刻画数据非线性的有监督稀疏编码方法。本课题的开展将会为提升纹理分析性能和增强其实用性提供新思路。
结项摘要
纹理是视觉数据的最基本特征之一,能为很多计算视觉任务提供重要的线索。本项目对图像/视频的纹理特征提取和描述开展了深入的研究,基于稀疏表达和间隙度描述子,围绕局部特征、全局特征、高层次特征三个方面提出了有效的纹理分析方法,并发展出一系列针对图像的稀疏表达方法。主要的研究成果包括:(1) 提出了基于字典学习的纹理局部特征分析方法,并应用于视觉数据局部纹理填充以及弱监督纹理分割,在这两个任务中皆取得了世界一流的实验结果;(2) 提出了面向视频的时空多重间隙谱,用于提取视频中的动态纹理特征,在多个动态纹理数据集上取得了世界一流的分类结果;(3)结合结构化稀疏编码和集成学习,提出了有效的数据集特征提纯方法,该方法能用于纹理图像乃至自然图像的高层次特征提取,并在多个图像分类任务中取得了优秀的结果; (4) 把雨滴图层建模为特定模式的纹理,针对其特有的属性,提出一种能用卷积操作实现的雨滴检测方法,该方法能有效地辅助卷积网络去除图像中的雨滴遮挡。基于以上成果,项目开展期间共发表了13篇高水平学术论文,其中包括2篇CCF-A类会议论文,6篇JCR-1区期刊论文,以及5篇JCR-2区期刊论文。项目的研究成果能应用于图像/视频的分类、分割和处理任务中,同时为稀疏表达、字典学习,以及深度学习领域提供了新模型和新思路。
项目成果
期刊论文数量(11)
专著数量(0)
科研奖励数量(1)
会议论文数量(2)
专利数量(2)
Image-based action recognition using hint-enhanced deep neural network
使用提示增强深度神经网络进行基于图像的动作识别
- DOI:--
- 发表时间:2017
- 期刊:Neurocomputing
- 影响因子:6
- 作者:Tangquan Qi;Yong Xu;Yuhui Quan;Yaodong Wang;Haibin Ling
- 通讯作者:Haibin Ling
Barzilai–Borwein-based adaptive learning rate for deep learning
基于 Barzilai–Borwein 的深度学习自适应学习率
- DOI:10.1016/j.patrec.2019.08.029
- 发表时间:2019
- 期刊:Pattern Recognition Letters
- 影响因子:5.1
- 作者:Liang Jinxiu;Xu Yong;Bao Chenglong;Quan Yuhui;Ji Hui
- 通讯作者:Ji Hui
Deeply Exploiting Long-Term View Dependency for 3D Shane Recognition
深入利用长期视图依赖性进行 3D Shane 识别
- DOI:10.1109/access.2019.2934650
- 发表时间:2019
- 期刊:IEEE Access
- 影响因子:3.9
- 作者:Xu Yong;Zheng Chaoda;Xu Ruotao;Quan Yuhui
- 通讯作者:Quan Yuhui
Removing Reflection From a Single Image With Ghosting Effect
使用重影效果消除单个图像的反射
- DOI:10.1109/tci.2019.2899320
- 发表时间:2020-01-01
- 期刊:IEEE TRANSACTIONS ON COMPUTATIONAL IMAGING
- 影响因子:5.4
- 作者:Huang, Yan;Quan, Yuhui;Ji, Hui
- 通讯作者:Ji, Hui
Attention with structure regularization for action recognition
用于动作识别的结构正则化注意力
- DOI:10.1016/j.cviu.2019.102794
- 发表时间:2019
- 期刊:Computer Vision and Image Understanding
- 影响因子:4.5
- 作者:Quan Yuhui;Chen Yixin;Xu Ruotao;Ji Hui
- 通讯作者:Ji Hui
数据更新时间:{{ journalArticles.updateTime }}
{{
item.title }}
{{ item.translation_title }}
- DOI:{{ item.doi || "--"}}
- 发表时间:{{ item.publish_year || "--" }}
- 期刊:{{ item.journal_name }}
- 影响因子:{{ item.factor || "--"}}
- 作者:{{ item.authors }}
- 通讯作者:{{ item.author }}
数据更新时间:{{ journalArticles.updateTime }}
{{ item.title }}
- 作者:{{ item.authors }}
数据更新时间:{{ monograph.updateTime }}
{{ item.title }}
- 作者:{{ item.authors }}
数据更新时间:{{ sciAawards.updateTime }}
{{ item.title }}
- 作者:{{ item.authors }}
数据更新时间:{{ conferencePapers.updateTime }}
{{ item.title }}
- 作者:{{ item.authors }}
数据更新时间:{{ patent.updateTime }}
其他文献
其他文献
{{
item.title }}
{{ item.translation_title }}
- DOI:{{ item.doi || "--" }}
- 发表时间:{{ item.publish_year || "--"}}
- 期刊:{{ item.journal_name }}
- 影响因子:{{ item.factor || "--" }}
- 作者:{{ item.authors }}
- 通讯作者:{{ item.author }}
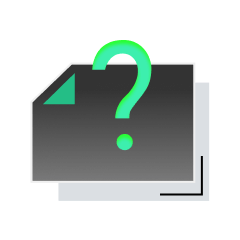
内容获取失败,请点击重试
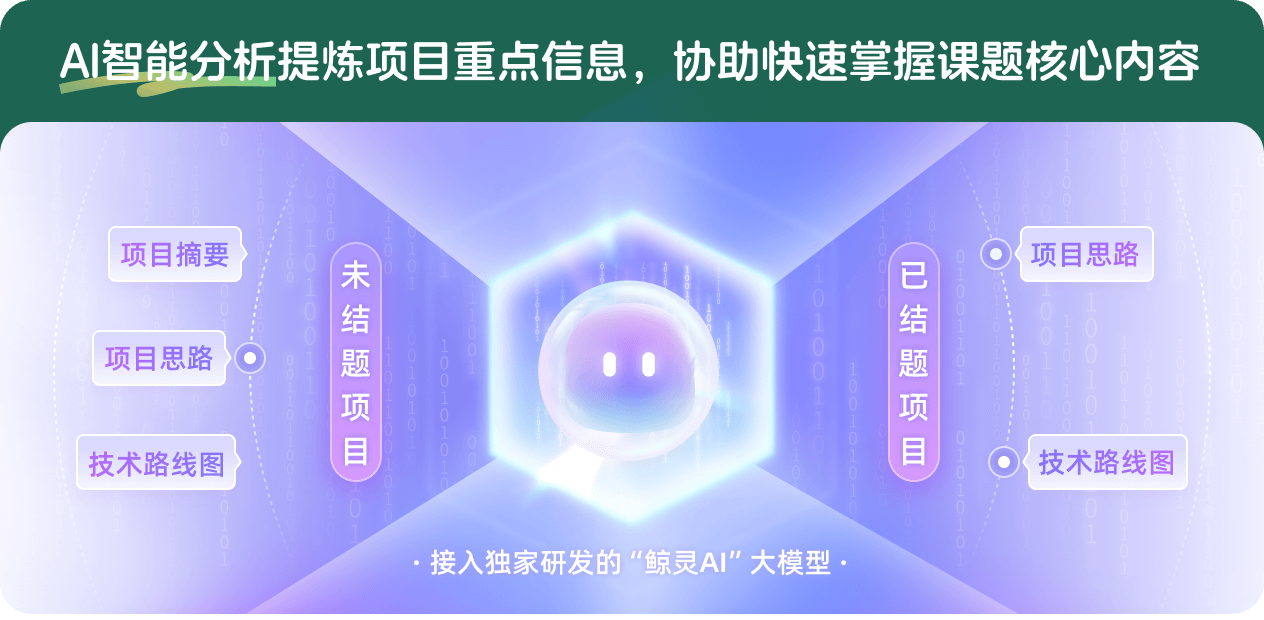
查看分析示例
此项目为已结题,我已根据课题信息分析并撰写以下内容,帮您拓宽课题思路:
AI项目摘要
AI项目思路
AI技术路线图
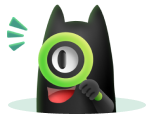
请为本次AI项目解读的内容对您的实用性打分
非常不实用
非常实用
1
2
3
4
5
6
7
8
9
10
您认为此功能如何分析更能满足您的需求,请填写您的反馈:
全宇晖的其他基金
图像压缩感知的无监督深度学习模型与方法
- 批准号:62372186
- 批准年份:2023
- 资助金额:50 万元
- 项目类别:面上项目
面向非特定流形结构化数据的稀疏编码关键技术研究
- 批准号:61872151
- 批准年份:2018
- 资助金额:61.0 万元
- 项目类别:面上项目
相似国自然基金
{{ item.name }}
- 批准号:{{ item.ratify_no }}
- 批准年份:{{ item.approval_year }}
- 资助金额:{{ item.support_num }}
- 项目类别:{{ item.project_type }}
相似海外基金
{{
item.name }}
{{ item.translate_name }}
- 批准号:{{ item.ratify_no }}
- 财政年份:{{ item.approval_year }}
- 资助金额:{{ item.support_num }}
- 项目类别:{{ item.project_type }}